Biostatistics and Bioinformatics in Clinical Trials
Donald A. Berry and Kevin R. Coombes
• The process of conducting cancer research must change in the face of prohibitive costs and limited patient resources.
• Biostatistics has a tremendous impact on the level of science in cancer research, especially in the design and conducting of clinical trials.
• The Bayesian statistical approach to clinical trial design and conduct can be used to develop more efficient and effective cancer studies.
• Modern technology and advanced analytic methods are directing the focus of medical research to subsets of disease types and to future trials across different types of cancer.
• A consequence of the rapidly changing technology for generating “omics” data is that biological assays are often not stable long enough to discover and validate a model in a clinical trial.
• Bioinformaticians must use technology-specific data normalization procedures and rigorous statistical methods to account for sample collecting, batch effects, multiple testing, confounding covariates, and any other potential biases.
• Best practices in developing prediction models include public access to the information, rigorous validation of the model, and model lockdown prior to its use in patient care management.
Biostatistics Applied to Cancer Research
The Bayesian approach predates the frequentist approach. Thomas Bayes developed his treatise on inverse probabilities in the 1750s, and it was published posthumously in 1763 as “An Essay towards Solving a Problem in the Doctrine of Chances.” Laplace picked up the Bayesian thread in the first quarter of the nineteenth century with the publication of Théorie Analytique des Probabilités. The works of both scientists were important and had the potential to quantify uncertainty in medicine. Indeed, Laplace’s work influenced Pierre Louis in France in the second quarter of the nineteenth century as Louis developed his “numerical method.” Louis’ method involved simple tabulations of outcomes, an approach that was largely rejected by the medical establishment of the time. The stumbling block was not how to draw inferences from data tabulations.1 Rather, the counter argument and the prevailing medical attitude of the time was that each patient was unique and the patient’s doctor was uniquely suited to determine diagnosis and treatment. Vestiges of this attitude survive today.
In the two hundred years after Bayes, the discipline of statistics was influenced by probability theory, and in particular, games of chance, dating to the early 1700s.4–4 This view focused on probability distributions of outcomes of experiments, assuming a particular value of a parameter. A simple example is the binomial distribution. This distribution gives the probabilities of outcomes of a specified number of tosses of a coin with known probability of heads, which is the distribution’s parameter. The binomial distribution continues to be important today. For example, it is used in designing cancer clinical trials in which the end point is dichotomous (tumor response or not, say) and assuming a predetermined sample size.
Clinical Trials
Consider a single-arm clinical trial with the objective of evaluating r. The null value of r is taken to be 20%. The alternative value is r = 50%. The trial consists of treating n = 20 patients. The exact number of responses is not known in advance, but it is known to be either 0 or 1 or 2 on up to 20. The relevant probability distribution of the outcome is binomial, with one distribution for r = 20% and a second distribution for r = 50%. These distributions are shown in Figure 19-1, with red bars for r = 20% and blue bars for r = 50%. More generally, there is a different binomial distribution for each possible value of r.
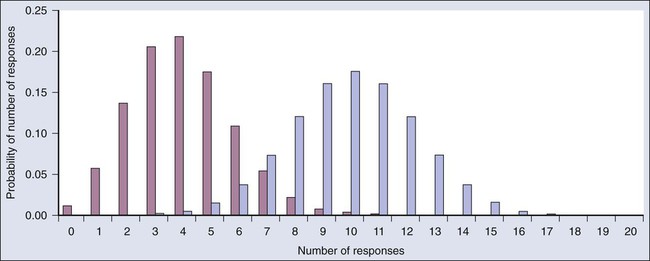
Both the frequentist and Bayesian approaches to clinical trial design and analysis utilize distributions such as those shown in Figure 19-1, but they use them differently.
Frequentist Approach
The frequentist approach to inference is based on error rates. A type I error is rejecting a null hypothesis when it is true, and a type II error is accepting the null hypothesis when it is false, in which case the alternative hypothesis is assumed to be true. It seems reasonable to reject the null, r = 20% (red bars in Fig. 19-1) in favor of the alternative, r = 50% (blue bars in Fig. 19-1) if the number of responses is sufficiently large. Candidate values of “large” might reasonably be where the red and blue bars in Figure 19-1 start to overlap, perhaps nine or greater, eight or greater, seven or greater, or six or greater.
The type I error rates for these rejection rules are the respective sums of the heights of the red bars in Figure 19-1. For example, when the cut point is 9, the type I error rate is the sum of the heights of the red bars for 9, 10, 11, etc., which is 0.007387 + 0.002031 + 0.000462 + … = 0.0100. When the cut points are 8, 7, and 6, the respective type I error rates are 0.0321, 0.0867, and 0.1958. One convention is to define the cut point so that the type I error rate is no greater than 0.05. The largest of the candidate type I error rates that is less than 0.05 is 0.0321, the test that rejects the null hypothesis if there are eight or more responses. The type II error rate is calculated from the blue bars in Figure 19-1, where the alternative hypothesis is assumed to be true. The sum of the heights of the blue bars for 0 up to 7 responses is 0.1316. Convention is to consider the complementary quantity and call it “statistical power:” 0.8684, which rounds off to 87%.
The distinction between “rate” and “probability” in the aforementioned description is important, and failing to discriminate these terms has led to much confusion in medical research.5 The “type I error rate” is a probability only when assuming that the null hypothesis is true. Probabilities of events requiring the truth of the null hypothesis are not available in the frequentist approach, and indeed this is a principal contrast with the Bayesian approach (described later).
Bayesian Approach
The calculation is intuitive when viewed as a tree diagram, as in Figure 19-2. Figure 19-2, A, shows the full set of probabilities. The first branching shows the two possible parameters, r = 20% and r = 50%. The probabilities shown in the figure, 0.50 for both, will be discussed later. The data are shown in the next branching, with the observed data, nine responses (nine resp) on one branch and all other data on the other. The probability of the data given r, which statisticians call the likelihood of r, is the height of the bar in Figure 19-1 corresponding to nine responses, the red bar for r = 20%, and the blue bar for r = 50%. The rightmost column in Figure 19-2, A, gives the probability of both the data and r along the branch in question. For example, the probability of r = 20% and “nine resp” is 0.50 multiplied by 0.0074.
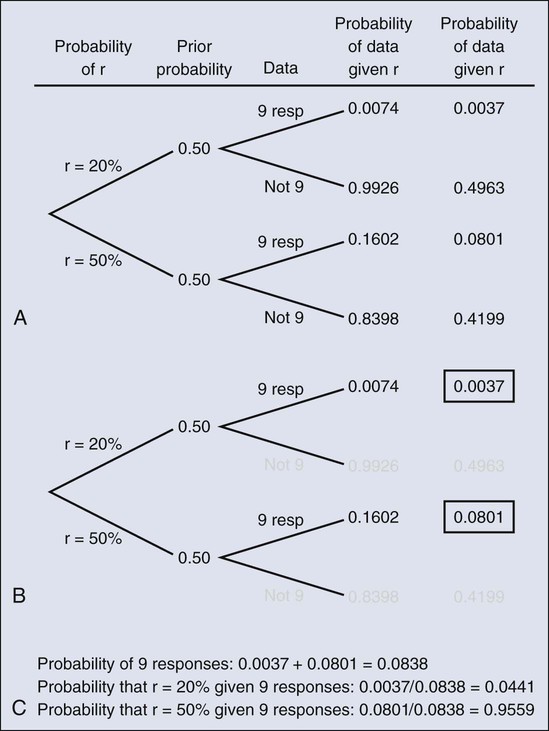
The probability of r = 20% given the experimental results depends on the probability of r = 20% without any condition, its so-called prior probability. The analog in finding the positive predictive value of a diagnostic test is the prevalence of the condition or disease in question. Prior probability depends on other evidence that may be available from previous studies of the same therapy in the same disease, or related therapies in the same disease or different diseases. Assessment may differ depending on the person making the assessment. Subjectivity is present in all of science; the Bayesian approach has the advantage of making subjectivity explicit and open.6
When the prior probability equals 0.50, as assumed in Figure 19-2, the posterior probability of r = 20% is 0.0441. Obviously, this is different from the frequentist P value of 0.0100 calculated earlier. Posterior probabilities and P values have very different interpretations. The P value is the probability of the actual observation, 9 responses, plus that of 10, 11, etc. responses, assuming the null distribution (the red bars in Fig. 19-1). The posterior probability conditions on the actual observation of nine responses and is the probability of the null hypothesis—that the red bars are in fact correct—but assuming that the true response rate is a priori equally likely to be 20% and 50%.
As an example of such a report, Figure 19-3 shows the relationship between the prior and posterior probabilities. The figure indicates that the posterior probability is moderately sensitive to the prior. Someone whose prior probability of r = 20% is 0 or 1 will not be swayed by the data. However, as Figure 19-3 indicates, the conclusion that r = 20% has low probability is robust over a broad range of prior probabilities. A conclusion that r = 20% has moderate to high probability is possible only for someone who was convinced that r = 20% in advance of the trial.
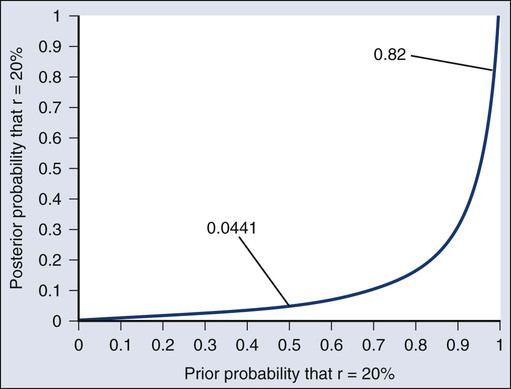
In the example, r was assumed to be either 20% or 50%. It would be unusual to be certain that r is one of two values and that no other values are possible. A more realistic example would be to allow r to have any value between 0 and 1 but to associate weights with different values depending on the degree to which the available evidence supports those values. In such a case, prior probabilities can be represented with a histogram (or density). A common assumption is one that reflects no prior information that any particular interval of values of r is more probable than any other interval of the same width. The corresponding density is flat over the entire interval from 0 to 1 and is shown in red in Figure 19-4, A.
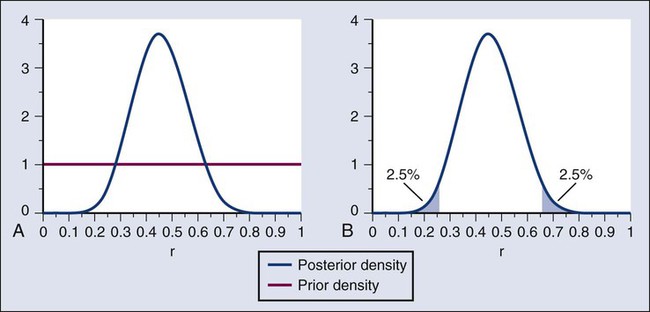
The probability of the observed results (9 responses and 11 nonresponses) for a given r is proportional to r9(1-r)11, with the likelihood of r based on the observed results. The prior density is updated by multiplying it by the likelihood. Because the prior density is constant, this multiplication preserves the shape of the likelihood. Thus the posterior density equals just the likelihood itself, shown in green in Figure 19-4, A.
The Bayesian analog of the frequentist confidence interval is called a probability interval or a credibility interval. A 95% credibility interval is shown in Figure 19-4, B: r = 26% to 66%. It is similar to but different from the 95% confidence interval discussed earlier: 23% to 64%. Confidence intervals and credibility intervals calculated from flat prior densities tend to be similar, and indeed they agree in most circumstances when the sample size is large. However, their interpretations differ. A credible interval has a particular probability that the parameter lies in the interval. Statements involving probability or chance or likelihood cannot be made for confidence intervals.
Any interval is an inadequate summary of a posterior density. For example, although r = 45% and r = 65% are both in the 95% credibility interval in the aforementioned example (Fig. 19-4), the posterior density shows the former to be five times as probable as the latter.
A characteristic of the Bayesian approach is the synthesis of evidence. The prior density incorporates what is known about the parameter or parameters in question. For example, suppose another trial is conducted under the same circumstances as the aforementioned example trial, and suppose the second trial yields 15 responses among 40 patients. Figure 19-5 shows the prior density and the likelihoods from the first and second trials. Multiplying likelihood number 1 by the prior density gives the posterior density, as shown in Figure 19-4. This now serves as the prior density for the next trial. Multiplying that density by likelihood number 2 gives the posterior density based on the data from both trials, and is shown in Figure 19-5. The order of observation is not important. Updating first on the basis of the second trial gives the same result. Indeed, multiplying the prior density, likelihood number 1, and likelihood number 2 in Figure 19-5 together gives the posterior density shown in the figure.
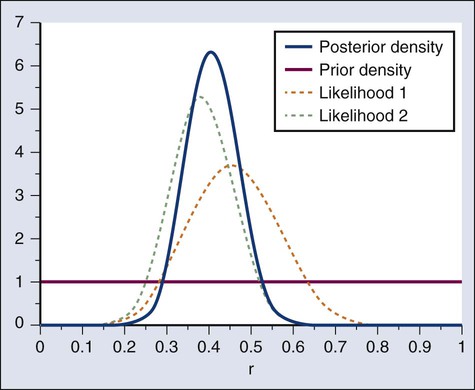
The calculations of Figure 19-5 assume that r is the same in both trials. This assumption may not be correct. Different trials may well have different response rates, say r1 and r2. In the Bayesian approach, these two parameters have a joint prior density. One way to incorporate into the prior distribution the possibility of correlated r1 and r2 is to use a hierarchical model in which r1 and r2 are regarded to be sampled from a probability density that is unknown, and therefore this density itself has a probability distribution. More generally there may be multiple sources of information that are correlated and multiple parameters that have an unknown probability distribution. A hierarchical model allows for borrowing strength across the various sources depending in part on the similarity of the results.9–9
Adaptive Designs of Clinical Trials
Randomization was introduced into scientific experimentation by R.A. Fisher in the 1920s and 1930s and applied to clinical trials by A.B. Hill in the 1940s.10 Hill’s goal was to minimize treatment assignment bias, and his approach changed medicine in a fundamentally important way. The RCT is now the gold standard of medical research. A mark of its impact is that the RCT has changed little during the past 65 years, except that RCTs have gotten bigger. Traditional RCTs are simple in design and address a small number of questions, usually only one. However, progress is slow, not because of randomization but because of limitations of traditional RCTs. Trial sample sizes are prespecified. Trial results sometimes make clear that the answer was present well before the results became known. The only adaptations considered in most modern RCTs are interim analyses with the goal of stopping the trial on the basis of early evidence of efficacy or for safety concerns. There are usually few interim analyses, and stopping rules are conservative. As a consequence, few trials stop early.
Figure 19-6 shows the sample size distribution for 10,000 trials under the assumption that r = 50%. The estimated type II error rate is 0.1987, which is the proportion of these 10,000 trials that reached n = 20 without ever concluding that the posterior probability of r = 50% is at least 95%. The sample size distribution when r = 20% is not shown but is easy to describe: 9702 of the trials (97.02%) went to the maximum sample size of n = 20 without hitting the posterior probability boundary and the other 3% stopped early (at various values of n < 20) with an incorrect conclusion that r = 50%. Thus 3% is the estimated type I error rate. The “estimated” aspect of these statements is because there is some error due to simulation. Based on 10,000 iterations, the standard error of the estimated power is small, but positive: 0.4%. The standard error of the type I error rate is less than 0.2%.
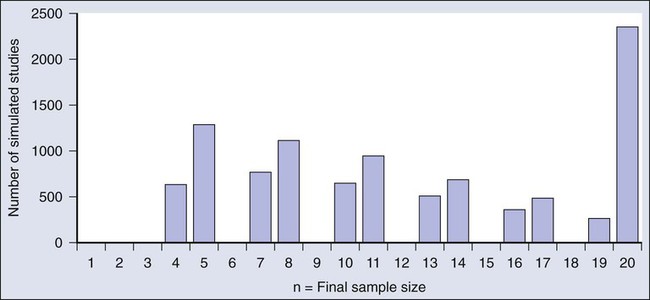
Because the Bayesian approach allows for updating knowledge incrementally as data accrue, even after every observation, it is ideally suited for building efficient and informative adaptive clinical trials.13–13 The Bayesian approach serves as a tool. As evinced in the example above, simulations enable calculating traditional frequentist measures of type I error rate and power. The Bayesian approach allows for addressing all aspects of drug development: What is the appropriate dose? What is the duration? What are concomitant therapies? What is the sequence? What is the patient subpopulation? Adaptations include dropping arms, adding arms, changing randomization proportions, changing the accrual rate, decreasing or increasing the total sample size, and modifying the patient population admitted to the trial.
Taking an adaptive approach is fruitless when there is no information to which to adapt. In particular, for long-term end points, there may be little information available when making an adaptive decision. However, early indications of therapeutic effect are sometimes available, including longitudinal biomarkers and measurements of tumor burden, for example. These indications can be correlated with long-term clinical outcome to enable better interim decisions.8,13
Biomarker-Driven Adaptive Clinical Trials and a Case Study
A standard approach to biomarker research is to examine interactions between biomarkers and treatment effects retrospectively, at the end of the trial. Examining interactions in ongoing clinical trials has many advantages.13 One is that requiring biomarker information for randomization means that information will be available for all patients, thus avoiding the “data missingness” problem that plagues retrospective biomarker studies.14 Another advantage is that when patients in a definable biomarker subset are not benefiting from a particular therapy, that subset can be dropped from the trial. In a multiarmed trial, biomarker subtypes that do not respond to a particular treatment can be excluded from that treatment arm, possibly gradually, using adaptive randomization.12 A consequence of excluding nonresponders is that focusing on responders means trials can become smaller. And of course, excluding patients who do not benefit reduces the extent of overtreatment of trial participants.
An adaptive biomarker-driven clinical trial can begin by including all the patients who meet the enrollment criteria for the trial, but then can continue by restricting enrollment to individuals with biomarker profiles that match those of the responding population as the results accumulate over the course of the trial. Biomarker subsets can be identified in advance, generated on the basis of the data in the trial, or some combination of the two can be used by incorporating historical data via the prior distribution, as previously described. Any approach is subject to multiplicities.5 Basing an assessment on the responding subsets is particularly prone to false-positive conclusions and requires a level of within-trial empirical validation. The extent of validation is a design characteristic that can be determined prospectively. False-positive rates and statistical power can be evaluated and controlled, usually requiring simulations. (Additional evaluations of biomarker-driven adaptive clinical trial designs are available in the literature.15–18)
An example of a complicated biomarker-driven, adaptively randomized clinical trial is I-SPY 213,19,20 (http://clinicaltrials.gov/show/NCT01042379) (http://www.ispy2.org/). The setting is neoadjuvant treatment for breast cancer in which the end point is pathological complete response (pathCR), which the U.S. Food and Drug Administration (FDA) has recently determined to be a registration end point in high-risk early breast cancer (http://www.fda.gov/downloads/Drugs/GuidanceComplianceRegulatoryInformation/Guidances/UCM305501.pdf). Investigational drugs are added to a taxane in the initial cycles of standard therapy. Similar trials are being explored in other diseases, including lymphoma.21
The Pace of Technological Change
The first microarrays that could simultaneously measure the messenger RNA (mRNA) expression levels of thousands of genes were developed in the mid 1990s.22,23 Within a few years, they were being used to study cancer.24–28 The technology evolved rapidly. For example, one of the major manufacturers of microarrays, Affymetrix, began with HuGeneFL GeneChip arrays that contained 6800 probe sets. They advanced over the course of little more than a decade through the U95A (12,000 probe sets), U133A (22,000), U133Plus2.0 (54,000), Human Gene ST 1.0 and 2.0, and Human Exon ST 1.0 and 2.0 microarrays. The typical time between the introductions of successive generations of microarray platforms was 2 or 3 years. Nevertheless, as that decade ended, researchers were increasingly moving away from microarray technology and toward RNA sequencing technology as their primary tool to measure mRNA abundance. The technologies used to measure DNA copy number alterations went through a similar evolution during this period.
The Breadth of Technologies
At the same time that microarray technologies were expanding to allow the entire transcriptome to be assayed in a single experiment, new technologies were emerging that focused on other biologically important molecules. The Cancer Genome Atlas (TCGA) recently started assaying approximately 500 tumors per cancer type using a broad spectrum of omics technologies.29 Initial plans called for microarray approaches to measure mRNA expression, microRNA (miRNA) expression, DNA copy number alteration, and methylation. These plans were supplemented by direct Sanger sequencing of a predefined set of cancer-related genes and by proteomic techniques, including mass spectrometry and reverse-phase protein lysate arrays. More recently, TCGA began applying second-generation sequencing technology to study DNA (whole genome or exome sequencing), RNA (RNA-Seq), and methylation (chromatin immunoprecipitation on sequencing, or ChIP-Seq) in these tumor samples.30,31
In at least one case, an entire class of biologically interesting molecules is itself relatively new. The first miRNA was discovered in 1993 by cloning the lin-4 gene in Caenorhabditis elegans.32 That there were numerous (highly conserved) miRNAs in different species did not become known until the simultaneous publication of three papers in 2001.35–35 A year later, Calin and colleagues36 demonstrated that miRNAs played an important role in cancer by showing that the “tumor suppressor genes” contained in the minimal deleted region of a recurrent deletion of chromosome 13q in chronic lymphocytic leukemia consisted of miRNA-15a and miRNA-16-1. Version 19 of the reference database, miRBase (http://www.mirbase.org/), maintained by the Wellcome Trust Sanger Institute, now lists 1600 precursors and 2042 mature human miRNAs.39–39 In addition, of course, there are now microarrays that simultaneously measure (almost) all of them.
It is unreasonable to expect manufacturers of scientific instruments to have the expertise required to program the wide variety of sophisticated statistical methods needed to account for differences in experimental design. It is surprising, however, that manufacturers do not always know the best ways to process their own raw data. For example, the first Affymetrix microarrays were designed using multiple pairs of “perfect match” and “mismatch” (MM) probes to target each gene. The idea was that the MM probes could be used to estimate nonspecific cross-hybridization, and so their initial algorithm quantified the expression of a gene as the average difference between the perfect match and MM probes. Li and Wong40 recognized that different probes for the same gene have different affinities, and thus their mean expression will vary even within the same sample. They replaced the simple average with a statistical model that accounted for different probe affinities.40 Bolstad and colleagues41 and Irizarry and associates42 showed that the MM probes increased the noise in the summary measurements with no compensating gain in signal clarity; they introduced an improved statistical method known as the robust multiarray average (RMA). Most current analyses of Affymetrix gene expression data use RMA.
Many advances in the methods for processing and analyzing “omics” data sets have come from academics and are made available as open source software. BioConductor (http://www.bioconductor.org) is the largest repository of such software packages, written for the R statistical programming environment.43,44 The BioConductor repository is the equivalent of a hardware store for statisticians and bioinformaticians searching for tools with which to analyze their latest data set. An alternative approach is provided by GenePattern45 (http://www.broadinstitute.org/cancer/software/genepattern/). GenePattern is a Web service where data can be uploaded and analyzed by biologists as well as statisticians. Modules can be written and shared in a variety of programming languages, then assembled into reusable self-documenting pipelines.
Batch Effects and Experimental Design
Batch effects are an unavoidable characteristic of data collected using cutting-edge technologies on research-grade scientific instruments.46 The instruments are often temperamental, requiring frequent tuning and calibration. Reagents change, and new printings of the “same” microarray can be subtly different. As a result, a batch of tumor samples analyzed in November may differ in many ways (although not of biological interest) from a similar batch analyzed in February. These technological effects are often large enough to swamp any interesting biology, and they can occur on the time scale of days rather than months. Unless accounted for in the experimental design, batch effects can ruin a perfectly good experiment. For example, in 2002, Petricoin and colleagues47 published an article that claimed they had developed a tool that could be used to diagnose ovarian cancer based on proteomic patterns detected in serum samples. Their results were soon questioned; it eventually transpired that the signals they had detected were technological artifacts, caused by running all of the controls on their mass spectrometer before all of the cases.50–50
There are several ways to deal with batch effects. First, pay attention to experimental design. Apply the basic principles of randomization and blinding to ensure that the contrasts of interest (tumor vs. normal or responder vs. nonresponder, for example) are not confounded with any batch effects that may be present. Second, if batch effects are suspected, there are existing statistical methods to model them and, to some extent, remove them.53–53
Additional challenges arise in the context of clinical trials. The standard normalization methods for most omics technologies require analyzing the entire set of data at once, which is impossible when patients arrive one by one and a decision about how to randomly assign them to treatment arms depends on the results of an individual assay. In the context of Affymetrix microarrays, this issue was addressed by the introduction of “frozen RMA,” which computes the normalization parameters from a training set and then applies them to new arrays one at a time.54 Not all omics technologies have completely faced this issue. In many cases, as with the comparative CT (or ΔΔCT) method for quantitative, real-time, reverse-transcription polymerase chain reaction (qPCR),55 the best solution may be to run a normalization or calibration standard alongside every experimental sample.
Multiple Testing and Overfitting
The traditional statistical response to the problem of multiple testing is to apply a “Bonferroni correction” to the cutoff used to define significance. If you test N features, then the P value should be less than 0.05/N in order for you to claim significance at the 5% level. With 20,000 features, this requirement translates into a P value < .0000025. You probably think that this requirement is extreme, and you may be right. The Bonferroni correction is extremely conservative. It tries to control the “family-wise error rate”; in other words, it attempts to make sure there are no (type I) errors in what you call significant. Continuing our hypothetical experiment, suppose you test 20,000 features and find that 50 of them have a P value <2.5 × 10−6. In only 5% of such experiments would you expect to find any errors in the list of 50 features. A less conservative approach is to control the false discovery rate (FDR), the expected fraction of false-positive (FP) calls among all positive calls: FP/(FP + TP), where TP is a true-positive call.56 Numerous methods have been developed to estimate the FDR in omics experiments.57–61 A few of these methods also let you estimate the number or rate of false-negative calls. Sometimes called type II errors, false-negative calls have an opportunity cost in terms of potential discoveries that you never make.
Best Practices
In response to problems encountered with the premature use of gene-expression signatures in clinical trials at Duke University,62 the U.S. Institute of Medicine (IOM) convened a committee to clarify the steps needed to move omics-based signatures from their initial discovery into clinical trials where they can affect patient management and, ideally, improve patient outcomes.63 The most important committee recommendation was to draw a bright line after the discovery and validation of a predictive model and before its application in a clinical trial. Before crossing that line, the test should already be validated, preferably in an independent set of samples or, if that is not available, by cross-validation. A clinical assay should be developed in a CLIA-certified laboratory, and the complete computational procedure must be completely specified and “locked down.” Any change to the procedure requires revalidation of the method before using it to affect patient management. An example is changing the prespecified cutoffs that distinguish patients with high-risk disease from those with low-risk disease; this warrants revalidating the method.
The IOM recommendations build on a long history of related guidelines. For example, the Early Detection Research Network proposed a sequence of phases for the development of biomarkers intended for cancer screening.64 However, because the requirements for a good screening biomarker are different from those for a prognostic or predictive biomarker, the Early Detection Research Network biomarker phases are not universally applicable. The minimum information about a microarray experiment (MIAME) standard defines data structures for making microarray data publicly available.65 Many journals require authors of papers that use microarray data to deposit them in a public repository (such as the Gene Expression Omnibus or ArrayExpress) using the MIAME standard. The MIAME data structures apply directly to a wide variety of technologies. One weakness of MIAME and related standards like the “minimum information about a proteomics experiment” (MIAPE)66 is that, although they describe the collection of metadata about the technology used in an experiment, they do not include a structured way to store clinical or demographic data about the patients whose samples were used in the experiments. Other important guidelines include the reporting recommendations for tumor marker prognostic studies (REMARK),67,68 the CONSORT statement for randomized trials,69 and the STARD initiative for diagnostic studies.70
Discovery Phase
• Make the data and metadata publicly available;
• Make the computer code and analysis protocols publicly available;
• Confirm the model using an independent, preferably blinded, set of samples; and
• Lock down the model, including molecular measurements, computational procedures, and intended clinical use.
The recommendations to make the data and code available grew out of the problems encountered at Duke University,71,72 but they reflect a larger concern with reproducible research in computationally intensive sciences.73–76 Because it is impossible to fully understand the biological rationale behind a complex predictive model involving tens or hundreds of genes, it is critically important to be able to confirm that the statistical analysis to discover the model was performed both sensibly and reproducibly. Checking these results requires access to both the original data and the computer code used to analyze it.
The requirement to specify the intended clinical use is also critical. Such information should answer the following questions: In what group of patients will the signature be tested? Will the result of the test be used to screen patients for early diagnosis? Will it provide prognostic information? Will it help select therapy? Will it be used to monitor minimal residual disease or the possibility of recurrence? Different applications require different performance characteristics to demonstrate the usefulness of a biomarker or signature and thus require different experimental designs and different types of controls. Research scientists who have the expertise to develop omics signatures but are not accustomed to designing or running clinical trials can fail to think carefully about these issues. In one example, researchers reported the discovery of peptide patterns with nearly 100% sensitivity and specificity to detect prostate cancer.77 The problem was that the cancer specimens came from a group of men with a mean age of 67 years, whereas the control subjects came from a group composed of 58% women, with a mean age of 35 years.78
Evaluation of Clinical Utility
Not every clinical trial evaluating the utility of a predictive model needs an IDE; the fundamental criterion is whether the molecular signature/assay device is being used to direct patient management. A prospective-retrospective79 study design in which the signature is measured on archived specimens to determine whether it would have been useful should not require an IDE. Similarly, a prospective clinical trial in which the signature is passively measured but not used to determine patient care would not need an IDE.
Clustering is Not Prediction
Clustering algorithms, especially in the form of two-way clustered, colored heat maps, have been ubiquitous in the microarray literature since its inception.80,81 Just as a list of genes (a signature) is not enough to make predictions, neither is clustering. There are certainly scientific questions that can be appropriately answered by applying a clustering algorithm; the canonical example involves identifying natural biological subtypes within a larger class of cancer samples. It is, however, possible to identify subtypes with different response profiles. The most likely way to find subtypes related to outcome is to start by performing feature-by-feature statistical tests that identify individual predictors of outcome. After selecting the best predictors, the resulting list of genes (signature!) can be used to cluster the samples. A statistical test can then be performed to determine if the resulting “cluster membership” variable is a good predictor of outcome.
Case Studies
Oncotype DX
The series of studies performed by Genomic Health to establish its Oncotype DX assay followed most of the procedures recommended by the IOM Committee. First, the researchers asked a well-defined clinical question about a well-defined patient population. Their goal was to predict the risk of distant recurrence after tamoxifen treatment of women with node-negative, estrogen-receptor-positive (ER+) breast cancer. Second, they used four existing microarray data sets from the published literature to select 250 candidate genes. Third, they developed a new assay using qPCR to measure the expression levels of those 250 genes in tumor samples. Fourth, they validated the assay by obtaining data from tumor samples that had been collected in three independent clinical trials of breast cancer, involving a total of 447 women. They performed univariate analyses and selected 23 genes that were associated with the risk of recurrence in at least two out of three qPCR data sets. They then constructed multivariate predictive models and further reduced the set of predictors to a panel of 16 cancer-related genes and five reference genes. Their approach was simple but elegant, reducing a multidimensional problem into a single number, a recurrence score (RS), which made for a straightforward validation process. The algorithm included cutoffs to separate patients into low-, intermediate-, and high-risk categories and was completely specified during this step. Fifth, they tested the prospectively defined qPCR assay and recurrence score algorithm for their ability to predict recurrence in a retrospective set of 668 samples from the National Surgical Adjuvant Breast and Bowel Project (NSABP) B14 trial, for which paraffin blocks were available.82 Another study showed that RS did not predict recurrence in women with node-negative breast cancer (regardless of ER status) who had received no adjuvant chemotherapy, which suggests that the signature depends on either the ER positivity or on the treatment.83 Although the RS was built to be prognostic, samples from the NSABP B20 trial showed that it helped predict response to chemotherapy for women with node-negative, ER+ breast cancer, with no apparent benefit for women with low recurrence scores.84
BATTLE Trial
The Biomarker-integrated Approaches of Targeted Therapy for Lung Cancer Elimination (BATTLE) trial was a prospective phase 2, biomarker-based, adaptively randomized study in 255 patients who had been pretreated for non–small cell lung cancer (NSCLC).85,86 The trial consisted of four treatment arms of targeted therapies, each of which was associated with a prespecified set of biomarkers that were anticipated to predict the efficacy of the therapy: erlotinib (EGFR), sorafenib (KRAS/BRAF), vandetanib (VEGFR2), and bexarotene plus erlotinib (RXR/CCND1). The primary end point of the trial was 8-week disease control. After an initial equal randomization period, patients were adaptively randomized to one of the treatment arms, based on the molecular biomarkers analyzed in fresh core needle biopsy specimens. Overall results included a 46% 8-week disease control rate, median progression-free survival of 1.9 months (95% confidence interval [CI], 1.8-2.4), and median overall survival of 8.8 months (95% CI, 6.3-10.6). The results confirmed that EGFR mutations predicted better 8-week disease control with erlotinib; high VEGFR2 expression, with vandetanib; and high CCND1 expression, with bexarotene plus erlotinib. The BATTLE study showed that interactions between treatments and biomarkers can be successfully used to guide adaptive randomization.
A follow-up trial, BATTLE-2, is currently under way. This trial also has four treatment arms (sorafenib; erlotinib; erlotinib plus MK2206; and selumetinib plus MK2206) and involves a similar patient population (patients with an EGFR mutation or an EML4-ALK fusion are not eligible for the trial). The fundamental innovation in this trial is that it is divided into two stages in which equal numbers of patients are treated and evaluated. One marker (KRAS mutation) is used, along with observed outcomes, to guide adaptive randomization during the first stage. Twenty prespecified biomarkers or signatures are being measured. At the end of the first stage, a retrospective analysis will be performed to determine the best predictive markers for each treatment arm. These markers will then be used prospectively to guide adaptive randomization in the second half of the trial. Extensive simulations have been performed to determine the operating characteristics of the randomization scheme.87