Chapter 1 Seizure Prediction: Its Evolution and Therapeutic Potential
Introduction
Seizure prediction has a long history, starting in the 1970s1 with very small data sets looking only at preseizure (preictal) events minutes to seconds before seizures. It has progressed over the past almost 40 years up to current methods, which use mathematical to analyze continuous days of multiscale intracranial electroencephalogram (IEEG) recordings.2 Seizure prediction research, most important, has given hope for new warning and therapeutic devices to the 25% of epilepsy patients who cannot be successfully treated with drugs or surgery.3 One of the most insidious aspects of seizures is their unpredictability. In this light, in the absence of completely controlling a patient’s epilepsy, seizure prediction is an important aim of clinical management and treatment. From a broader view, seizure prediction research has also transformed the way we understand epilepsy and the basic mechanisms underlying seizure generation. Seizures were once viewed as isolated and abrupt events, but we now view them as processes that develop over time and space in epileptic networks. Thus, what started as a goal of predicting seizures for clinical applications has expanded into a field dedicated to understanding seizure generation.
The study of seizure generation necessarily encompasses a large collaborative effort between mathematicians, engineers, physicists, clinicians, and neuroscientists. However, it also requires large volumes of clinical data, which has led to more specific collaborations between epilepsy centers. These partnerships have come about through The International Seizure Prediction Group (ISPG), which held its Third Collaborative Workshop on Seizure Prediction in Freiburg, Germany, in October 2007. This workshop, and its two predecessors, allowed various groups to share computational methods, data, and ideas, and to focus on basic research and its translation to clinical relevance.
There is a large gulf between understanding how seizures are generated and the eventual goal of preventing seizure occurrence. Although studies in the literature have focused on prospectively testing seizure prediction methods,4,5 no study to date has yet confirmed the ability of a method to predict seizures better than random, or with accuracy sufficient for prospective clinical trials or eventual implementation in patients. Much of the blame for this performance failure resides in two important challenges, both of which have recently been solved. First, until recently, there was no consensus on the amount and quality of data required to conduct appropriate prospective prediction studies. Second, the statistical methods for designing experiments, and the metrics by which to judge successful algorithm performance, were not in place.2 Recent research has definitively advanced progress in these areas.6–8 Looking further ahead, for successful prediction devices to emerge, many technical questions will need to be resolved to design systems that not only warns the patient of a seizure but also intervenes to preempt it. For example, the intervention strategy (drug versus stimulation or other method), the clinical interface (sensors, classifiers, etc.), and the number and site of electrode placement are just a few of the problems under investigation that will need definitive solutions.
Historical Background
Epileptologists have long been aware that many patients with epilepsy know that their seizures are not abrupt in onset, and that they can often predict periods of time when seizures are more likely to occur. Many clinical findings support the idea that seizures are predictable. An increase in blood flow in the epileptic temporal lobe has been seen as much as 12 minutes before the onset of seizures.9,10 Clinical “prodromes” are noted in more than 50% of patients, according to Rajna et al.,11 with more refined measurements made recently by Haut et al.12,13 An increase in oxygen availability and blood oxygen level dependent signals on magnetic resonance imaging (MRI) have also been noted before seizures.14,15 Preictal changes in heart rate have been reported in several studies.16–18 However, these clinical findings do not reveal how long before a seizure the first changes in seizure generation occur or by what method a seizure might be predicted.
Seizure prediction research has evolved via diverse mathematical and engineering approaches, from its starting point in the 1970s. Viglione and Walsh not only implemented the first electronic classifier to solve this problem, in the form of an analog neural network, but they also created an actual device and tested it on patients with epilepsy19 (Figure 1-1). This device used scalp EEG electrodes to record signals for classification.1,20 Linear approaches looking at “absence” seizures using surface electrodes detected preictal changes up to 6 seconds before seizure onset.21 Another early study found differences between 1-minute preictal EEG epochs and control epochs.22 Using another approach, many studies have evaluated the rate of spikes in EEG before seizures with some23 finding predictive differences, but with the majority finding no predictive value.24,25 Novel mathematical approaches started with nonlinear systems using the largest Lyapunov exponent. They detected decreases in chaotic behavior in the minutes before temporal lobe epileptic seizures,26,27,28,29 with subsequent studies claiming prediction over hours5,30 (Figure 1-2). These and other early studies are important because they support the conceptual idea that seizures are not isolated events but rather develop over time.
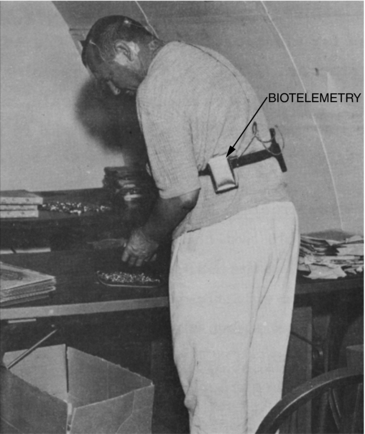
Figure 1–1 A patient using one of the earliest seizure warning devices invented by Viglione et al.19 The biotelemetry unit was developed by Biocom, Inc., Culver City, CA.
However, there were methodological problems with these early studies. First, they focused on the preictal period and did not include prolonged interictal recordings; thus there was doubt about the specificity of the findings with regard to time. Second, they also lacked specificity with regard to space because they used univariate measures and one-channel recordings. Third, most of these studies employed small numbers of patients and small selected data sets. Finally, and perhaps most important, these studies did not have specific, well-thought-out statistical criteria for success and did not demonstrate performance measured against a chance predictor. These criteria would later reveal hidden biases in experimental data sets, not apparent in initial studies. The identification of these difficulties was one of the main products of the First International Collaborative Workshop on Seizure Prediction.2
As the field evolved, progressive attempts were made to conquer these challenges. The first problem was addressed in multiple studies in which a number of mathematical measures, starting with the correlation dimension,31 were shown to distinguish preictal and interictal periods using expanded data sets. The second problem was addressed with bivariate and multivariate measures, such as the largest Lyapunov exponent of two channels,32–34 and other methods fusing information from multiple channels and measures over time,4,35,36 simulated neuronal cell bodies,37 and measures for phase synchronization and cross correlation.36,38,39
But none of these studies addressed the lack of large data sets, containing prolonged interictal periods and sufficient numbers of seizures for classifier training and testing. As a result, their findings came into question in later studies carried out on unselected and more extended EEG recordings. Though the correlation dimension31 reliably demonstrated preseizure changes, these changes were also seen at other times, when seizures did not occur, a problem of many early (and present) methods. For this reason, although many methods were considered promising, they were found not to predict seizures, as verified by other investigators using alternative statistical methods.40,41 Statistical concerns also engendered criticism of studies involving prediction work invoking Lyapunov exponents.42 The predictive value of accumulated energy measures35 was also called into question by Harrison et al. and Maiwald et al.43,44 though these groups did not separate recordings into wakefulness and sleep, as was required in the original study. Therefore, the suitability of nonlinear mathematics,45 as well as linear methods in seizure prediction, was called into question.
These questions spawned The First International Collaborative Workshop on Seizure Prediction, held in Bonn, Germany, in 2002. The goal of the workshop was to give a tutorial on state-of-the-art methods for predicting seizures to students and investigators, to share scientific ideas, to compare different mathematical methods on a large common data set (five continuous intracranial EEG recordings from patients undergoing presurgical evaluation for medically intractable epilepsy), and to set goals and standards for future work in the field. The meeting attendees published a collection of papers summarizing this meeting, including a summary paper outlining a consensus on future research in seizure prediction, under the name of “The International Seizure Prediction Group.”2 Suggestions for future research studies in the field included: (1) that some of the first thorough easiest studies to be performed, to guarantee sampling data from networks involved in generating seizures, are recordings demonstrating should examine data from very focal disorders, such as unilateral temporal lobe; (2) that seizure prediction should focus on EEG events rather than events having only overt clinical symptoms; (3) that a top priority for the field was to establish an international database of high-quality intracranial EEG recordings for collaborative research; (4) that it was key that prolonged recordings, with statistically sufficient amounts of interictal and ictal data, be analyzed; and (5) that there needed to be better development of statistical models for seizure prediction and a consensus on what constitutes success.2 These last two issues were seen as major impediments to success in the area of seizure prediction over the years. Unfortunately, there was, at that time, no consensus on what statistical performance measures should be used to present results. There was also much debate about the predictability of seizures from patients with types of epilepsy other than that originating from the temporal lobe.
Results from the individual group presentations at the meeting were of interest. The conclusions of studies on this large test data set showed poor performance for univariate measures46,47 and better performance for bi- and multivariate measures.30,48,49 Nonlinear measures were not found to outperform linear ones.49 Of interest, the use of machine learning for automated feature exploration was introduced at this meeting, though this method did not yield results significantly better than other multivariate measures.4
Presentations at the meeting also included results on spatial contributions to seizure generation in the brain. The finding of preictal changes in channels remote from the seizure onset zone4,48,49 necessarily changed the conceptual framework for understanding seizure generation. Whereas earlier studies taught that seizures are not abrupt in onset, but more likely develop over time, these new studies demonstrated that even focal seizures likely develop across a network of distributed regions that are functionally connected. These findings challenged the more common accepted view of the epileptic “focus.”
The conference ended on a humorous but poignant note summing up the results of different prediction methods presented. This was a quote from what would later be a paper by the Bonn group: “The null hypothesis of the non-existence of a pre-seizure state cannot be disproved.”49,50 Papers detailing the research and presentations of each group at this workshop were later published in a special edition of the journal Clinical Neurophysiology (vol. 116, no. 3, 2005).
Gathering Momentum
There was a 5-year hiatus between the first Seizure Prediction Workshop and the second, which took place in Bethesda, Maryland, in February 2006 and was sponsored by the National Institute of Neurological Disease and Stroke (NINDS). During this period, investigators in the field continued to critically examine their work and developed new insights into why prior methods had failed to produce reliable algorithms that could predict seizures better than random chance. This introspection was released in a flurry of talks and papers leading up to and after the meeting. This body of work focused primarily on statistical concerns about current methods for tracking seizure generation over time,6–8,34,41,49,51 but also on clinical reports from patients to bolster seizure prediction work, reinforcing the concept that seizures are generated over time.12,52 The second workshop provided an explosive outlet for these ideas, with harsh criticism of much of the early work in this field. Rather than interpreting this as a failure of the field of seizure prediction to make progress, most investigators came away from the meeting with a renewed sense of focus and a feeling that tremendous progress had been made. One of these leaps forward was the acceptance of seizure generation as likely being a “stochastic process.” In this scheme there are periods of increased probability of seizure onset, which may or may not lead to seizures, depending on unknown internal and external factors. This focused investigators interested in seizure generation on forecasting probabilities, rather than specific events.
During the time leading up to the Third Collaborative Workshop on Seizure Prediction, held in Freiburg, Germany, at the end of September 2007, it became clear that the priority items for progress in the field, identified at the first workshop in 2001, had been aggressively pursued. There was now a consensus on statistical methods required to prove success at seizure prediction (see).6,7 Standards for data to be used in prediction experiments were also put forward, and a model of seizure generation as a stochastic process in distributed neuronal networks was growing in popularity. At the same time, there was increasing interest in the role of neurophysiologic markers for epileptic brain and in the spatial and temporal evolution leading up to seizures. The idea behind this work is that there are particular neurophysiological markers of an epileptic brain that appear interictally and that may evolve in their temporal and spatial distribution as the probability of seizure increases.35 In particular, attention began to focus on high-frequency oscillations (HFOs) during this period.