Chapter 120 Brain–Computer Interfacing Prospects and Technical Aspects
It is possible that the brain–computer interface (BCI) has origins in this type of thinking. Thus, there is a logical extension of an electrical organ to control electrical devices. A BCI, sometimes called a brain–machine interface (BMI), is a thought translation device for direct communication pathways between a brain and an external device. We know the brain’s electrical activities are capable of pattern changes that can be actively conditioned. A simple example is production of alpha wave activity during meditation. The key problem with BCI is the neural interface. Neural signals need to be accurately detected and translated into useful command signals to effect control over computers or prostheses. The difference between BCIs and neuroprosthetics is the direction of the connection and this is increasingly blurred by feedback mechanisms. The terms can be used interchangeably, and both endeavor to achieve the same aims, such as restoring sight, hearing, movement, communication, or cognitive function. In many ways it is best described broadly as neuromodulation, the process of augmenting, inhibiting, modifying, or regulating the electrical or chemical neural interface to achieve therapeutic effect (Table 120-1). Neuromodulation is thus a very broad field covering every body function controlled by the central nervous system and embracing multiple clinical specialties. For the focus of this chapter we will restrict our discussion to classical BCI systems with signal acquisition, feature extraction, translation algorithm, and operational effect. The question is no longer whether damaged or degenerative brains can provide a foundation for BCI, as this is established, but rather how efficient can we make it?
Table 120-1 Neuromodulation: Merging Human and Machine
Motor | Sensory | Disease |
---|---|---|
|
BCI, brain–computer interface; DBS, deep brain stimulator; SC, spinal cord; VNS, vagus nerve stimulator.
The BCI development began by working backwards. Throughout the century, stimulation of the brain revealed fascinating aspects of how machines could control brain activities. Classic demonstrations of this were developed by Jose Delgado who was able to stop a charging bull with electric stimulation at specific sites within the brain.1 The stimulation gave the illusion of behavioral control. A practical extension of this developed into the deep brain stimulator (DBS), which is capable of controlling a variety of abnormal movements and is beginning to be applied to epilepsy, pain, psychiatric conditions, and numerous other diseases.2 Specific research on BCIs commenced in the 1970s at the University of California-Los Angeles and the expression “brain–computer interface” first appeared in scientific literature.3
The earliest clinical work suggested the possibility of neuromodulation by indirect means using noninvasive electromagnetic interfaces that are dominated by two “write-only” techniques—transcranial magnetic stimulation (TMS) and direct current stimulation (DCS)—and three “read-only” techniques—electroencephalography (EEG), magnetoencephalography (MEG), and functional magnetic resonance imaging (fMRI). There is no “read-write” noninvasive technology, but there is invasive technology capable of sensing, analyzing, and stimulating for effect. EEG is the most extensively studied BCI noninvasive interface (Fig. 120-1). In the 1980s, Farwell and Donchin4 developed a scalp EEG-based BCI using P300 (an EEG-evoked potential) signals to allow normal subjects to communicate words to a computer and thereby “speak” through a computer-driven speech synthesizer. The analyses suggest that this communication channel can be operated accurately at the rate of about 2.3 characters per minute. Speed can be increased by using paradigm matrixes (5 to 7 characters per minute), but severely impaired patients may not do well due to reduced attention span and other cognitive difficulties.5 A similar device allows volunteers wearing virtual reality helmets to use their P300 EEG signals to control elements in a virtual world including turning lights on and off.6
In the 1990s, Niels Birbaumer used EEG recordings of slow cortical potential to give paralyzed patients limited control over a computer cursor to write responses.7 Patients were trained to move a computer cursor by controlling their EEG activity. The training often took many months and the communication process was slow, requiring more than an hour for patients to write 100 characters. In 1998, Peckham and colleagues8 used a 64-electrode EEG skullcap to return limited hand movements to a quadriplegic. By concentrating on simple but opposite movements like up and down, the beta-rhythm EEG output was analyzed to identify patterns in the noise. Above average activity was set to “on” and below average to “off,” thus enabling the subject to control a computer that drives the nerve controllers embedded in his hands, restoring some movement. The essential step in all EEG BCIs is “recognition” of a specific control signal extracted from the Fourier components of the signal and identification of frequency bands related to each control action for each subject. The relatively long measurement period needed to obtain the low-frequency signatures imposes a significant limitation on how rapidly EEG BCI can transfer information. This approach requires a large computational investment of microprocessor arrays. The results are promising by providing communications conduits for severely disabled individuals. The accuracies of information transfer varying among individuals range from 65% to 98% (average 88%). Thus, there remain substantial barriers to using EEG as an effective and efficient controller of BCI.
Invasive or direct BCIs use probes that are implanted directly into the gray matter of the brain to produce the highest-quality signals in real time from areas of a few microns. Changes in spike frequency can be detected very rapidly. The first direct human BCI was an outgrowth of work on primates over a long period of time and demonstrated the ability of single motor cortex neurons to change their firing pattern in a plastic manner.9–11 Phillip Kennedy patented the use of a glass cone electrode filled with neural tissue to promote growth of local neurons into the electrode for recording. Kennedy and Bakay built the first cortical BCI by implanting neurotrophic electrodes into monkeys who could then quickly learn to voluntarily control the firing rates of individual and multiple neurons in the primary motor cortex by rewarding the generation of appropriate patterns of neural activity. The ability to record signals over a long period of time from the same neuron suggested that the activity of an individual neuron could be reliably used to control machinery. The first clinical approach was to restore communication to patients.12,13 The obvious machine to control was a computer, for through the computer it is possible to communicate with the world. The first paradigm was an on–off binary response (Fig. 120-2). The intention was to communicate by thought alone. The next endeavor was to learn to control individual units so that the patient was able to move a cursor across a computer screen and stop at the appropriate computer icons to produce computer-generated phrases such as “Hello, my name is JR.” The next step was to move in two directions and use the computer as a virtual typewriter to produce short responses by stopping on letters or punctuation icons (Fig. 120-3). This for the first time demonstrated unequivocally that cortical neurons engaged by movement intentions persist in the motor cortex years after disease or injury to the motor system, and that neuronal spikes and field potentials derived from motor cortex can be used by paralyzed patients to operate BCI. Thus movement programming remains. Also for the first time in humans, it was demonstrated that cortical motor neurons could learn non-motor tasks. Following this success, a company, Neural Signal, Inc. (Atlanta, GA), was created.
The second major clinical long-term study of patients with direct BCI is being conducted by Cyberkinetics Neurotechnology Systems, Inc. (Foxborough, MA). Similar to the neural signal background, this work grew out of primate studies.14–16 The procedures are very analogous to neural signal procedures, although the electrode is very different.17,18 The electrode uses 100 tines, and because of that the amount of information needed to be transferred is entirely too large to go through a radiofrequency transmitter; instead, the information is transferred directly to a head post and subsequently connected to the electronics. There are a variety of computer tasks that can be performed and even simple demonstrations of robotic movements (Fig. 120-4). Although the signals are theoretically stable, the initial patients have failed to demonstrate that a large number of signals can be maintained for more than 6 months without requiring intervention. In addition, there is a setup time required every day to evaluate which units are still active and able to be engaged. There is then an additional retraining time required. Although both BCI systems are extremely sophisticated, utility is still quite limited.19
Patient Selection
Because of the experimental nature of the procedure, the patients have been limited to intransigent medical conditions and thus must have severe fixed and/or progressive deficits. This has included patients with high cervical spinal cord injury, stroke (especially brain-stem stroke), cerebral palsy, and amyotrophic lateral sclerosis (ALS), but other diseases may qualify. The risks of surgery must be small to ensure benefit for the patient. Ethical issues are critical and need to be carefully addressed.20 This is a very vulnerable population who will accept high risks for the chance of minimal gain. An informed caregiver should be an integral part of the informed consent process.
The key to success of any surgical procedure is to carefully design the inclusion and exclusion criteria. There is always a temptation to include exceptional patients. This should be avoided. Inclusion criteria should reflect the patient’s primary needs and not stretch the limits of what is achievable. Thus, for a spinal-cord-injured (SCI) patient, it would be control over a motorized wheelchair, whereas for an amputee it would be fine motor control over a prosthetic device. Current interventions are for patients with severe disability. In the case of ALS, the clinical history is well recognized and obtaining permission for surgery before severe deficits occur could be an ideal situation to advance knowledge of what is possible for these patients. After severe deficits ensue, it will be harder to train and regain function. Similarly, although the stroke or SCI patient may need to have a high degree of disability, some residual motor activity, even if nonfunctional, could be extremely useful and might be essential for optimal function of BCI. Plasticity is necessary and any residual motor function may suggest that the circuitry is still readily available for the BCI. Again, even minimal residual sensory feedback may be ideal for advancing the learning curve and the development of analogs. Obviously, the brain must have some functional activity in order to be useful and an fMRI may be necessary to identify functional activity. These patients are generally quite sick and may require interventions of various kinds unrelated to the BCI surgery. These setbacks can be quite serious and the selection process should avoid patients who are metabolically unstable, and in general, are marginally fit for surgical intervention. Communication with these patients is essential and some form of communication has to be distinctly demonstrated. The more robust the communication, the better off the investigator and the patients are as they proceed in the trial.
The rationale for exclusion criteria need to be carefully considered. These must reflect the limits of both the device, as well as the limits of surgery. Exclusion criteria are: (1) medically unstable and unable to tolerate surgical procedures, (2) cognitively impaired—incapable of learning the protocol, (3) unable to adequately communicate to obtain proper consent and follow instructions, or (4) a lesion or atrophy in the intended implant area.21 A history suggesting poor wound healing, chronic infections, or cancer should be exclusion criteria, as these will impact ability to evaluate and use any type of interface. Furthermore, patients who are angry about their injury or emotionally unstable or depressed should be avoided. Acute injuries are to be avoided until it is clear that spontaneous recovery will not occur. On the other hand, it is probably reasonable to proceed with the implantation as soon as a probable permanent deficit is identified because the potential for plasticity is needed. However, studies from stroke and SCI patients demonstrate the potential for plasticity changes even months or years following injury so timing may be less critical than originally thought.22–25 The close interaction between neural interface devices and neuroplasticity via motor imagery training suggests that neural interface devices may improve or retain functional recovery.
Lead Configuration
There are basically three types of electrodes considered useful for recording cortical or subcortical activity: nonpenetrating, penetrating, and bioelectrode (Fig. 120-5). The characteristics of the electrode will determine which localizations are possible and the electronics follow. There are nonpenetrating surface recording electrodes, either from scalp EEG26–29 or from pial surface electrocorticogram (ECoG).30–32 The EEG activity is in the microvolt range and occasionally can be difficult to separate from electrical noise in the environment. The advantages are the ease of use, portability, temporal resolution, low setup cost, and low risks compared to invasive interfaces. The disadvantages are poor spatial resolution (field potentials recording a 3- to 5-cm radius), poor signal-to-noise separation, susceptibility to electromyographic noise, the extensive training required, and the low volume of output. Communication devices based on scalp EEG are commercially available. Recent applications have found mu and beta waves to be the most effective for BCI as they decrease activity during preparation and movement and thus reflect motor output pathways even for imagined movements.33 Patients with ALS or SCI have learned to use scalp EEG signals to communicate and operate simple orthoses.26,27 Steady-state, visual-evoked potentials can be used as an electrophysiologic correlate of visual spatial attention that may be harnessed to achieve control in BCI.34 Self-regulation of slow cortical potentials has been tested for BCI communication but is compromised by high intersubject variability.35
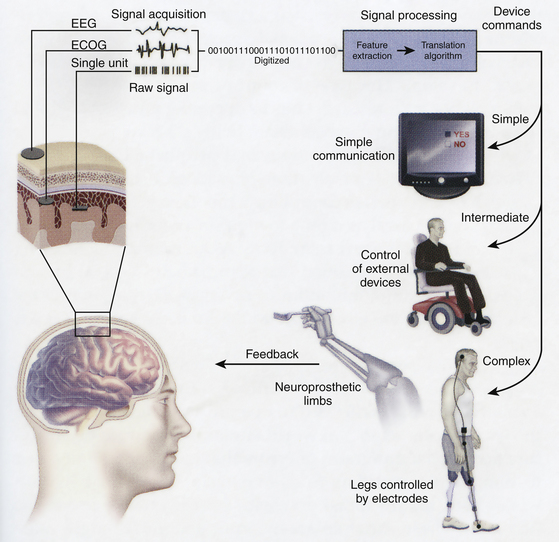
FIGURE 120-5 This schematic shows essential components of BCI system. The essential elements to practical functioning of a BCI platform follow.1 Signal acquisition: The BCI system’s recorded brain signal input (i.e., EEG, ECoG, single unit action potentials). This signal is then digitized for analysis.2 Signal processing, conversion of raw information into a useful device command: This involves both feature extraction, determination of a meaningful change in signal, and feature translation, the conversion of that signal alteration to a device command.3 Device output, overt command, or control functions administered by the BCI system. These outputs can range from simple forms of basic word processing and communication to higher levels of control such as driving a wheelchair or controlling a prosthetic limb. As a new output channel, the user must have feedback on his or her overt device output to improve performance of how to alter the electrophysiological signal. BCI, brain–computer interface.
(From Leuthardt EC, Schalk C, Moran D, et al. The emerging world of motor neuroprosthetics: a neurosurgical perspective. Neurosurgery. 2006;59:1-14, with permission.)
The ECoG is called a partially invasive BCI because the leads are implanted inside the skull but rest outside the brain rather than within the gray matter. The signal is far more robust (magnitude of five times) than scalp EEG where the cranium deflects and deforms signals. Signals can be acquired using either a subdural or epidural electrode placement. ECoG is a very promising BCI modality because it has higher spatial resolution (0.5–1 cm), better signal-to-noise ratio, wider frequency range, better long-term stability, and fewer training requirements than EEG BCI. There is a very low risk of cortical or leptomeningeal scar-tissue formation that will obscure the signal.36,37 The basic technique for electrode placement is well established in the treatment of epilepsy, and thus transfer to BCI has lower technical difficulty and lower clinical risk than invasive single-neuron recording. A disadvantage is that these are still relatively large regions that may or may not be able to be specifically and separately controlled for three-dimensional movements. Because the electrodes are on the surface, they can move and lose specific registration. Another disadvantage is the apparent low rate of information transfer. Signaling still requires detection of frequency changes, which require averaging that slows detection and data transfer. Currently, the study patients had severe epilepsy that required temporarily implanted invasive monitoring for localization prior to resection of an epileptogenic focus with rare exception.38 There are specifically designed cortical recording electrodes in development to provide better signals for BCI.
ECoG BCI was first trialed in humans in 2004 by Leuthardt and colleagues. The use of ECoG activity in closed-loop real-time control of a cursor to increase flexibility and potential utility of such recordings has been demonstrated, in association with motor or speech imagery.30 Recently, implanted 16-microwire arrays (1-mm electrode spacing) placed over the primary motor cortex while the patient performed simple contra- and ipsi-lateral wrist movements demonstrated that small regions of primary motor cortex (<5 mm) carry sufficient information to separate multiple facets of motor movements.31 Others have indicated that ECoG from other cortical regions involved in higher cognitive functions may serve as a readily self-controllable input for BCI.32 This research indicates that control is rapid, requires minimal training, and may be an ideal tradeoff with regard to signal fidelity and level of invasiveness. This suggests that a high level of control with minimal training requirements may be possible with ECoG BCI for people with motor disabilities.
The other types of electrode are penetrating. Implanted in the gray matter, these invasive devices produce the highest quality signals for BCI but are prone to scar tissue. This is the most popular electrode of BCI as the time scale shifts from 1 to 2 seconds to 200 to 400 milliseconds. These are implanted either in the cortical or subcortical areas. They may be individual or multiple. They may record field potentials (1 mm) or individual units (200–400 microns). High-amplitude local-field potential (LFP) recordings have the advantages of not requiring specific units to be identified, and theoretically can be maintained indefinitely by simply recording activity over a discrete area. In the motor cortex, these represent neural activity from widespread neurons that are temporally coupled and related to motor planning and preparatory activity rather than precise motor encoding.14 Elsewhere, LFP in parietal cortex may be useful to recognize intention and planning.39,40 Intracortical LFP signals were able to be used in a crude manner to control digital movements of a cyber hand.41 This system requires threshold data and there is only a very short detection delay. Extracranially obtained LFP can be used, but appear to be less useful due to limited information content and loss of signal. The disadvantages of LFP recording are that the field must respond to conditioning, which is in some ways more difficult than individual units and control of multiple degrees of freedom has not been demonstrated. These appear quite capable of providing binary or switching function in real time, but lack the robustness of proportional responses for fine prosthesis movements.
The most commonly used invasive electrodes are multiple prongs (Fig. 120-4A), such as the Utah or Michigan electrode arrays.42,43 Various versions of these arrays all suffer from a number of basic problems that have recently been reviewed.44,45 Over time, units are lost in what has been called the “fork in the Jell-O problem.” The brain is in constant motion and the tines can injure the tissue resulting in gliosis and damage to blood vessels and neurons with an approximately 100-micron radius.46 Designing the probe as a platform removes the handle of the fork but the connections can still add torque to the platform in terms of micromovements in response to Valsalva maneuvers or any brain movement such as from minor trauma. The presence of local electrical inhomogeneities (encapsulation, edema, coatings) around the electrode shank can substantially degrade the neural recordings. This has been frequently seen in monkey studies and clinical studies. There is then difficulty determining whether the same unit can be continuously recorded over time, and therefore, currently reregistration of the units is performed on a daily basis. With time, gliosis can surround the electrode and insulate it so that no action potential can be recorded.47 This provides a significant disadvantage for long-term programming and conditioning. An alternative is an array of microwires that can be implanted in loose bundles48 or fixed grids.49 It is unknown whether these behave differently in subcortical locations versus cortical, but it is unlikely that they would. The multitude of leads presents multiple problems for transmitting all the signals.
A new generation of electrodes should be based on the following known challenges:
1. There is a large gap between the number of routinely recorded and theoretically recordable neurons.
2. An ideal electrode should have a very small volume, commensurate with the neuron’s scale to minimize tissue injury.
3. There should be a large number of recording sites for monitoring many neurons simultaneously (nanoelectromechanical system–based devices might reduce the limitations inherent in wire electrode systems).
In order to overcome the loss of signal, multiple approaches to electrode design are being tested. Newer nanoporous silicon surface electrodes may be more biocompatible,50 but the problem is not solved. A very different recording device is the microelectromechanical system (MEMS) that allows recording from multiple cortical layers and as far away as 140 μm.51 The MEMS uses a tetrode to separate action potentials from more than 1000 neurons in three-dimensional space. Another promising technique uses a conducting polymer (PEDOT) that can be polymerized directly within living neural tissue resulting in an electrically conductive network that is integrated within the tissue.52 The PEDOT filaments penetrate out into the tissue far enough to bypass fibrous scar tissue and contact surrounding healthy neurons. A biologically inspired microelectrode uses ultrananocrystalline diamond (UNCD) to fabricate thin films with a unique combination of chemical inertness, controllable electrical conductivity, micromachinability, and biocompatibility to enable cell growth on the UNCD surface. This bioengineered surface is sensitive to the biological environment, thus providing a new platform for advanced nanoelectrodes.53,54 Addition of drug or trophic factors may also help.55
Finally, there is the bioelectrode. Electrodes such as the neurotrophic electrode integrate the regenerative biology with recording techniques. Briefly, the neurotrophic electrode consists of a chronically implanted conical glass cone through which neuronal processes can grow (Fig. 120-6). The cone contains gold contact recording wires (two to three) inserted through the wide end of the cone. These wires differentially record the electrical activity of the ingrown neuronal processes (axonal and dendritic).11 Prior to implantation, neurotrophic substances or peripheral nerve fascicles are placed inside the cone (Fig. 120-7). An autologous sciatic nerve was first used as the attractant for axonal growth following the work of Benfey and Aguayo,56 which showed Schwann cells from the sciatic nerve when placed in rat cortex would induce axonal sprouting from the underlying neurons. Schwann cells from any nerve source will provide the same effect. Following injury, Schwann cells will activate over 60 genes and produce growth factors, cytokines, neuropeptides, cytoskeleton proteins, and extracellular matrix molecules.57 Other sources of neurotrophic factors and matrix molecules can also prove effective.11,58
The key feature of this electrode compared with any other is that instead of bringing the electrode’s metal recording tip to the neurons, the neuronal processes grow against the electrode tip and are held there as a bridge of tissue isolated within the glass cone. Gliosis helps hold the electrode in place and the glass insulates the neuronal process within it so consistent and unambiguous action potentials are obtained. After a few weeks, the processes that grew into the core from surrounding neurons become myelinated and can be recorded but it takes 3 to 4 months for the signals to mature. Since the tissue grows through the cone and anchors it in the cortex, the recording wires in the cone move with the recorded tissue during normal movements of the brain, thus providing both mechanical and signal stability. The actual duration for which recordings can be made has not been determined but individual units have been recorded for up to 19 months in monkeys and 5 years in humans.9,58 This type of integration provides a potentially more stable environment and the same single units can be recorded for a long period of time. A disadvantage is that only a small number of these will be adequately isolated for use in conditioning. Although multiple neurotrophic electrodes can be placed and appear not to significantly damage the adjacent cortex, the increased number of electrodes will again produce problems in transmitting multiple signals for analysis. Modifications are underway to reduce the cone size and increase the number of units detected. Other disadvantages are the irregular manufacturing of the electrode, the delay of months for the electrode to mature after implantation, and the very extensive and intensive training required to gain fine control of a cursor. Finally, this approach also appears to be adequate for recording cortical activity but suboptimal for subcortical activity.
Single-unit conditioning is believed to be the key to implementing proportional signals for real-time interfaces for prosthetics. Fetz and Finocchio59 showed that individual and multiple neurons in the primary motor cortex could change firing patterns if they were rewarded for generating appropriate patterns of neural activity. Thus, a cortical motor neuron could be used to voluntarily control movement in a single direction. In 1973, Fetz and Baker60 demonstrated using operant conditioning in monkeys that one neuron in the motor cortex could be conditioned to fire while another suppresses firing by biofeedback. This suggests that not only can the desired neurons be conditioned to fire at specific rates, but also that undesirable neurons can be suppressed if contributing to unwanted background activity, thus physiologically improving the signal-to-noise ratio. Burnod et al.61 confirmed that conditioning of motor neuronal firings in monkeys is possible during task-specific conditioning.
Wyler et al.62 confirmed that monkeys could control firing rates within predetermined firing ranges or levels (expressed as the modal interspike intervals of unit firings). Wyler also demonstrated63 that when pairs of units were recorded and reward was contingent upon the firing rate of one of the pair, the other unit’s firing rate did not co-vary with the firing rate of the conditioned unit. This result, in addition to the results of Fetz et al.,64 suggests that two units can be conditioned separately especially if they are related to different movements, or separate aspects of one movement (i.e., agonist/antagonist pairs). By implanting neurotrophic electrodes into monkeys, Kennedy and Bakay provide evidence that chronic multiple units that fire reciprocally could be conditioned from a single electrode.9,10 Nicolelis and his colleagues demonstrated multiple precise control functions in three-dimensional arm movement can be achieved by the isolation of multiple independent single units and an algorithm developed to predict movement from a population of neurons.65,66 Not until 2002 was it possible to demonstrate a three-dimensional, closed-loop, real-time control of a cursor. Lebedev et al.67 argues that the brain reorganizes to create a new network representation of the robotic appendage separate from the representation of the animal’s own limbs. There are now a number of primate studies16,68,69 demonstrating that neural output from motor cortex can be used to control computer cursors or prostheses as effectively as natural hand movement while recording signals from far fewer neurons than Nicolelis required (15–30 neurons versus 50–200 neurons). Rhesus monkeys could be trained to use a BCI to track visual targets on a computer screen with or without assistance of a joystick. In addition to developing prediction kinematic and kinetic parameters of limb movements, BCIs that predict electromyographic or electrical activity of muscles are being developed that could be used to restore mobility in paralyzed limbs by electrically stimulating muscles.70 Andersen’s group used recordings of premovement activity from the posterior parietal cortex in their BCI.68
The work of Wyler and colleagues also suggested that closed-loop control was required for operant conditioning to occur: If the spinal cord dorsal columns were transected at C1-2, operant conditioning was diminished but not lost.71 If the monkey’s contralateral ventral roots were sectioned, however, operant control was totally lost.72 This provides evidence against open-loop control. However, the role of vision and auditory compensatory closing the loop were not systematically investigated and other data suggest visual and auditory feedback may be sufficient.73 Closed-loop or open-loop BCI can function as control devices. Thus, a monkey while operating a joystick or reaching for food can operate an open-loop BCI in real-time that controlled a separate unseen robot arm.65 However, control of grip force in the absence of somatosensory feedback may be very inaccurate.74–77 These results have important implications for patients since their lesion would leave an open feedback loop. It is desirable that the intended movement (imagined) be consistent with the actual prosthetic movement. There are differences in imagined movements among controls, paraplegics, and quadriplegics.78,79 However, it is well documented that motivated paralyzed humans can condition units using auditory and visual feedback via internal hemispheric, cerebellar, and/or brain-stem loops. It should also be noted that the best results were obtained when some residual function was present.
For simple tasks such as a binary switch, all the leads will provide effective signals. Less invasive and robust recording of LFP could be favored for this binary function. Dual switches provide information for communication with a cursor on a two-dimensional screen and simple environmental controls. For complex communication such as speech generation or prosthetic control, multiple functionally independent real-time signals (probably single unit potentials) are needed. The number of units is still being determined, but the clear advantage here is that the signals can be proportioned, which has far greater impact than a binary switch. Translating the multiple neural signals into a useful device control signal is called “decoding.” It can either recover specific information about precise movement intentions from a population of neurons involved in motor processing or the signal can be used as a surrogate for the actual process. The conventional strategy is to apply linear or nonlinear mathematical methods to convert the population activity for movement to specific control commands that emulate the intended movement. This decoding takes time to calibrate and confirm but once established are reliable control systems.44 Increasingly, it is being realized that motor cortex can adopt rapidly to new motor skills80,81 such that arbitrary signals unrelated to the task can be modulated after brief training sessions to provide high quality control signals.60,82,83 These individual signals may provide the equivalent information as a population of signals for prosthetic control. Clearly, conditioning and plasticity still play a role and the model cannot be simple “wire tapping” to reconstruct the motor system.
One aspect of BCI that has not been addressed is the potential for stimulation to augment control. Such augmentation clearly has been demonstrated in the past and certain types of behaviors can be initiated.1 Subthreshold stimulation could enhance performance of the recording neurons as has been done for stroke or head injury.22,23 Alternatively, stimulation could arrest unwanted activity such as seizures.84,85 There is also the potential to put in sensory feedback signals by converting mechanical position, directional, and force information into microstimulation of the sensory cortex. The experimental basis to provide this type of substitute feedback already exists,86–88 indicating that direct bidirectional communication between the brain and neuroprosthetic devices can be achieved. The possibilities are endless but so may be the ethical problems of mind control. However, the possibility of true mind control is extremely remote.89 Whether this type of stimulation would be helpful for controlling prosthetics or communication devices remains to be determined. Regarding feedback stimulation, the bioelectrode is least useful and penetrating metal electrodes are most useful.
Lead Location
Location of the electrode will dramatically depend on the type of electrode. Most electrodes record cortical activity and require only a gyral surface. There is the possibility that interhemispheric, subfrontal, or even subtemporal sites can be useful. Initial work has been undertaken to place electrodes in specific sites such as motor cortex or speech cortex in order to use the highly segmented activity to generate specific impulses. This type of work does not require that motor activity necessarily be performed by motor neurons. Thus, the movement of a cursor across a computer screen can serve the function of a positive feedback for cortical activity. Motor cortex is useful in this regard only if it has controllable firing patterns (functional activity). The use of fMRI to document changes in cortical activity with imagined movement is essential. With neurosurgical image-guidance techniques, it is relatively straight forward to identify the exact area of the cortex for implantation. At the time of craniotomy, macroscopic optical imaging of blood flow could further refine localization for implants. While these areas may be useful in generating potentials for prosthetics, it is also quite possible that premotor, prefrontal, supplementary motor, or even parietal areas may be as useful.
Likewise, the use of the Broca’s motor speech area may be very useful for developing the use of individual phonemes to generate words and speech, but other areas such as Wernicke’s area may be equally useful. It is simply unknown at this time. The intent to speak is detectable as heightened neural activity in Broca’s area. Implanted neurotrophic electrodes in Broca area have been used to detect this electrical activity.90 The electrical data recorded by the electrode is exported to a computer that decodes the pattern of firings of the neural signals. Decoding these patterns into the 39 phonemes in English has crudely permitted reconstruction of simple speech. The same units are active for phoneme detection and vowel production, suggesting that the common underlying function is articulation. Thus far this has been achieved only offline.
Electrical activity, especially motor activity can be highly concentrated in subcortical sites and this has been used in at least a test of principle.91 These were on awake, intact patients; and following injury, the results may be less robust. We know in disease states that units no longer only respond to a single joint movement but commonly respond to multiple joints.92 There is growing evidence that rhythmic movements are not part of a more generalized discrete movement system but are separate neurophysiologically.93 One could question at least on a theoretical basis whether extrapyramidal sites would be as useful as pyramidal sites in motor control. Clearly, they could be used for other types of control. There is the potential for multiple sites. This may be especially important for positive and negative feedback controls as well as other safety issues. The minimum requirement would be the functional activity in the target area of brain. There is at least one instance where inadequate cortical activity failed to produce anticipated results, despite fMRI indications of activity.21 Not only must there be activity, but there must be functional activity that can be able to be regulated. Another large unknown is the effect of sensorimotor reorganization.
Implantation of Electronics
The type of electrodes will determine exactly where the opening will be made, how the lead will be inserted, what type of support devices will be needed, and finally, how to protect the electrode and its wires from damage. Obviously, if the cerebrospinal fluid space is entered, repair of that space will be essential. Preventing migration of the electrodes is important, while allowing flexibility so that micromovements do not traumatize the adjunct brain. Once the wires exit the calvarium, they are then connected to the electronics. The electronics may simply exit to a post on the skull. This type of device has been used with cyberkinetics.18 The advantage is that cyberkinetics is the simplest means of getting the data from the 100 electrodes. The disadvantages are clear that infection can cause continuous problems for these plugs and that osteomyelitis may eventually weaken the supports. Trauma can dislodge the device, and mechanical connections mandate support personnel and makes stand-alone capabilities impossible. Thus, this technology will not be used in the future. Since Delgado’s work in 1969, the use of completely implanted electronics has been employed in both monkeys and humans.1 Internalized systems are being investigated.94–96 A cortical microelectrode array with 16-channel broadband recording is being tested to determine whether digital infrared impulses can be transcutaneously transmitted effectively.94 Future surgery must include total internalization of wireless devices for real-time biotelemetry. Power for the internal electronics can come from an induction source that requires the placement of an external device over the induction coil in order to transmit the energy through the skin. “Smart caps” and other types of devices have been used for this purpose. However, in the future this also restricts the mobility and utility of the device. With the potential for the device to be “on” 24/7, a control “switch” to activate or inactivate the device will be needed to save energy. Operating at low power will be essential for long-term energy conservation and heat dissipation of an implanted device. Ultimately, some type of internal battery, preferably rechargeable through induction, should provide the energy, mobility, and independence necessary for optimal function. While not yet available, one can conceive of batteries that draw on energy sources from the patients and thus may be self-sustaining.
Maintenance
Maintenance of the device needs to be simplified. Materials will have to be nontoxic, capable of withstanding minor trauma, waterproof, and not subject to breakdown over the duration of need for the implant. Microelectromechanical systems are undergoing revolutionary advances in performance and capabilities.96,97 These will be needed to reach the goals of the ideal device (Table 120-2). Upgrades may come in rapid succession and the device should have components that can be interchangeable when upgraded. In addition, the electronic components must have internal sensing devices and fail-safe type capability to protect patients from short circuits, power surges, and so on. Currently, none of the devices have such protection for patients. Seizures can easily disrupt such a system and with a damaged brain, this may be highly likely. Similarly, sleep states could dramatically affect the firing patterns and require safety considerations. One of the major problems is getting the information from the electrodes to the computer for analysis. With a great number of potential signals, there is too much data that has to be rapidly transferred. Increasingly, more and more data processing will have to be performed closer and closer to the electrodes. Information sent from this source to the receiving unit will have to be processed to a high degree in order to obtain mobility. Single one-to-one correspondence from 100 electrodes is simply not possible. If only a few of those have information that is of value, then restricting transmission to only those electrodes decreases some of the tremendous amount of information that would have to be transferred. There is a tremendous amount of work trying to define specific ensembles of neurons with weighted values that are greater than the population as a whole.98–100 Further development of robust decoding analogs to decrease this to 10 to 20 channels of information will make a workable real-time system. The key will be to accurately and rapidly transmit highly enriched information.
Safe | No harmful effects from internal or external electrical or magnetic interference. |
Dependable | Available 24/7 on demand without need for caregivers to activate or interface except for upgrades or emergencies. |
Accurate | Information transfer must have a very high level of fidelity to be effective. |
Real-Time | Able to perform immediately and appropriately at the speed of normal speech or movement. |
Reliable | Durable construction and long-term performance without the need for frequent maintenance adjustments or resettings. |
Interactive | Sophisticated analogs to anticipate and simplify activity so that fatigue does not become the primary limiting factor. |
Available | Off-the-shelf products capable of individualized performance at reasonable prices. |
Aesthetic | Minimal obtrusion and maximum acceptable for the patient. |
The growth in this field will continue to be slow as long as the work is supported by small grants and small companies. Too much equipment is “off the shelf” and not specifically designed for this work. Too much computer programming is simply transferred by “cutting and pasting” from old programs instead of developing new types of programming that is necessary to advance the field more rapidly. The electrodes are decades old and new innovations are not rapidly forthcoming. While it is true that we don’t know enough about the electrophysiology of the nervous system, the main problem for slow growth is lack of resources. This all may change in the near future as the National Institutes of Health increases support for BCI101 and countries such as Japan and France make BCI part of their national scientific goals.
Future
The biggest impediment of BCI technology at present is the lack of a sensor modality that provides safe, accurate, and robust access to brain signals. It is conceivable or even likely that such a sensor will be developed within the next 20 years. The use of such a sensor should greatly expand the range of communication functions that can be provided using a BCI. Currently, another form of neuromodulation, the Neuropace system (RNS, NeuroPace, Inc., Mountain View, CA), is being investigated in a multicenter study. This is capable of analyzing the patient’s ECoG and then triggering electrical stimulation when specific ECoG characteristics reach a threshold for a possible antecedent of an ictal event. An initial small open-label study reported that seven of eight patients had greater than 45% reduction in seizures.84,85 Though much work is needed to optimize microelectrodes for BCI and ultimately to develop BCIs that are capable of three-dimensional control, it is possible that a combination of BCI technology with high-frequency stimulation may lead to further advances in neurorehabilitation. This symbiotic relationship between neural interface technology and the nervous system is expected to maximize functional gain for individuals with various sensory, motor, and cognitive impairments, eventually leading to better quality of life. To serve patients, BCI systems must become safe, reliable, cosmetically acceptable, quickly mastered with minimal ongoing technical support, and highly accurate even in the face of mental distractions and the uncontrolled environment beyond a laboratory. Because stimulation itself may result in more responsive cellular milieu, biotechnology can provide solutions for nerve regeneration via studies of guidance channels, stem cells, growth factors, and gene therapy for regenerative nerve systems, via improvement of electrode material and design.102–105
Electrophysiology is the “gold standard” for investigating neuronal signaling. A new generation of optical probes is challenging that function. The photon may progressively replace the electron for probing neuronal function, particularly for targeted stimulation and silencing of neuronal populations. These probes allow us to measure and control neuronal signals with spatial resolution and neuronal specificity that already greatly surpass those of electrophysiology. In the future, light reactive BCI devices could be developed. These might involve implanting lasers inside the skull that would be focus on neurons and the neuron’s reflectance measured by a separate sensor. When a monitored neuron fires, the change in laser light wavelengths reflects the change in the calcium concentration from rest to increase during evoked and spontaneous neuronal activity.106,107 This would allow neuronal signals to be recording without contact with tissue and reduce the risk of scar tissue. New combinations of electrophysiology and imaging should lead to transformational discoveries in BCI.
Another “wireless” approach uses light-sensitive ion channel proteins (opsins) such as channel rhodopsin to control the activity of transfected neurons in vivo. Specific brain cells could be transfected to express the gene for the opsins, which function as ion pumps, moving ions across the cell membrane to alter the cell resting potential. The ion pumps are light-sensitive, so they pump chloride ions into cells when activated by yellow-green light. Lowering voltage inside the cells silences their firing.108 Using optics to systematically drive or inhibit an array of distinct circuit elements in freely moving parkinsonian rodents, Gradinaru and colleagues109 found therapeutic effects within the subthalamic nucleus by direct selective stimulation of afferent axons projecting to this region. The results demonstrate an optical approach for controlling disease circuitry by selectively controlling individual components. Preclinical testing of this approach has started in non-human primates, to assess its safety as a potential therapy for Parkinson’s disease, epilepsy, and chronic pain.
As with all advances in technology, the U.S. military has been exploring applications for BCIs to enhance troop performance as well as to develop systems for silent communication. Since 2000, the Pentagon has funded efforts to build prototype cockpits, missile control stations and infantry trainers that can sense the operator’s focus of attention, and adjust how they present information to find targets easier by tapping their unconscious reactions.110 The Silent Talk program111 aims to “allow user-to-user communication on the battlefield without the use of vocalized speech through analysis of neural signals.” Before being vocalized, speech exists as word-specific neural signals in the mind. The research aims to detect and analyze the word-specific “pre-speech” neural signals (EEG), which occur before speech is vocalized. After identifying the patterns, they could then transmit the statement to another soldier. They are also testing in a project to devise mind-reading binoculars. The “soldier-portable visual threat warning devices” would include a software system to monitor brain activity and quickly alert soldiers to potential threats (the idea being that EEG can recognize “neural signatures” for target detection before the conscious mind becomes aware of a potential threat or target).
Bakay R.A.E. Limits of brain–computer interface. Neurosurg Focus. 20(5), 2006. e6
Benabid A.L. What the future holds for deep brain stimulation. Expert Rev Med Devices. 2007;4:895-903.
Benfey M., Aguayo A.J. Extensive elongation of axons from rat brain into peripheral nerve grafts. Nature. 1982;296:105-107.
Birbaumer N., Ghanayim N., Hinterberger T., et al. A spelling device for the paralysed. Nature. 1999;398:297-298.
Delgado J.M. Radio-controlled behavior. NY State J Med. 69(3), 1969. 413-417
Donoghue J.P. Bridging the brain to the world: a perspective on neural interface systems. Neuron. 60(3), 2008. 511-521
Farwell L.A., Donchin E. Talking off the top of your head: a mental prosthesis utilizing event-related brain potentials. Electroencephalogr Clin Neurophysiol. 1988;70:510-513.
Fetz E.E. Volitional control of neural activity: implications for brain-computer interfaces. J Physiol (Lond). 2007;579:571-579.
Fetz E.E., Finnocchio D.V. Operant conditioning of specific patterns of neural and muscular activity. Science. 1971;174:431-435.
Fraser G.W., Chase S.M., Whitford A., et al. Control of a brain–computer interface without spike sorting. J Neural Eng. 6(5), 2009. 55004
Gradinaru V., Mogri M., Thompson K.R., et al. Optical deconstruction of parkinsonian neural circuitry. Science. 324(5925), 2009. 354-359
Guenther F.H., Brumberg J.S., Wright E.J., et al. A wireless brain–machine interface for real-time speech synthesis. PLoS ONE. 4(12), 2009. e8218
Kennedy P.R.. Comparing electrodes for use as cortical control signals: tiny tines, tiny wires, or tiny cones on wires: which is best?, 3rd ed. The Biomedical Engineering Handbook, Boca Raton, FL, CRS/Taylor and Francis, 2006 :vol. 1 Chapter 32-1
Kennedy P.R., Bakay R.A. Activity of single action potentials in monkey motor cortex during long-term learning. Brain Res. 1997;760:251-254.
Kennedy P.R., Bakay R.A. Restoration of neural output from a paralyzed patient by a direct brain connection. NeuroReport. 1998;9:1707-1711.
Kennedy P.R., Bakay R.A., Moore M.M., et al. Direct control of a computer from the human central nervous system. IEEE Trans Rehab Eng. 2000;8:198-202.
Leuthardt E.C., Schalk G., Wolpaw J.R., et al. A brain-computer interface using electrocorticographic signals in humans. J Neural Eng. 2004;1:63-71.
Loeb G.E., Walker A.E., Uematsu S., et al. Histological reaction to various conductive and dielectric films chronically implanted in the subdural space. J Biomed Mater Res. 1977;11:195-210.
Musallam S., Corneil B.D., Greger B., et al. Cognitive control signals for neural prosthetics. Neuroscience. 2004;305:259-262.
Park M.C., Zerris V., Hochberg L., et al. First experience with an implantable human neuromotor prosthesis. Neuromodulation. 9(1), 2006. 19-20
Patil P.G., Carmena J.M., Nicolelis M.A.L., et al. Ensemble recordings of human subcortical neurons as a source of motor control signals for a brain–machine interface. Neurosurgery. 55(1), 2003. 27-35
Pfurtscheller G., Guger C., Muller G., et al. Brain oscillations control hand orthosis in a tetraplegic. Neurosci Lett. 2000;292:211-214.
Truccolo W., Hochberg L.R., Donoghue J.P. Collective dynamics in human and monkey sensorimotor cortex: predicting single neuron spikes. Nat Neurosci. 13(1), 2010. 105-111
Wyler A.R., Lange S.C., Neafsey E.J., et al. Operant control of precentral neurons: control of model interspike intervals. Brain Res. 1980;190:29-38.
Yeun T.G., Agnew W.F., Bullara L.A. Tissue response to potential neuroprosthetic materials implanted subdurally. Biomaterials. 1987;8:138-141.
1. Delgado J.M. Radio-controlled behavior. NY State J Med. 69(1), 1969. 413-417
2. Benabid A.L. What the future holds for deep brain stimulation. Expert Rev Med Devices. 4(6), 2007. 895-903
3. Vidal J.. Toward direct brain–computer communication, Mullin L.J., editor, Annual Review of Biophysics and Bioengineering, Palo Alto, CA, Annual Reviews, Inc., 1973;vol. 2:157-180.
4. Farwell L.A., Donchin E. Talking off the top of your head: a mental prosthesis utilizing event-related brain potentials. Electroencephalogr Clin Neurophysiol. 1988;70:510-513.
5. Kubler A., Furdea A., Halder S., et al. A brain–computer interface controlled auditory event–related potential (p300) spelling system for locked-in patients. Ann N Y Acad Sci. 2009;1157:90-100.
6. Bayliss J.D.. Use of the evoked potential P3 component for control in a virtual apartment. IEEE Trans Neural Syst Rehabil Eng2, 2003;11:113-116.
7. Birbaumer N., Ghanayim N., Hinterberger T., et al. A spelling device for the paralysed. Nature. 1999;398:297-298.
8. Smith B., Tang Z., Johnson M.W., et al. An externally powered, multichannel, implantable stimulator-telemeter for control of paralyzed muscle. IEEE Trans Biomed Eng. 45(4), 1998. 463-475
9. Kennedy P.R., Bakay R.A. Activity of single action potentials in monkey motor cortex during long-term learning. Brain Res. 1997;760:251-254.
10. Kennedy P.R., Bakay R.A., Sharpe S.M. Behavioral correlates of action potentials recorded chronically in the Cone electrode. NeuroReport. 1992;3:605-608.
11. Kennedy P.R., Mirra S., Bakay R.A.E. The cone electrode: ultrastructural studies following long-term recording. Neurosci Lett. 1992;142:89-94.
12. Kennedy P.R., Bakay R.A. Restoration of neural output from a paralyzed patient by a direct brain connection. NeuroReport. 1998;9:1707-1711.
13. Kennedy P.R., Bakay R.A., Moore M.M., et al. Direct control of a computer from the human central nervous system. IEEE Trans Rehab Eng. 2000;8:198-202.
14. Donoghue J.P., Sanes J.N., Hatsopoulos N.G., et al. Neural discharge and local field potential oscillations in primate motor cortex during voluntary movements. J Neurophysiol. 79(1), 1998. 159-173
15. Serruya M.D., Hatsopoulos N.G., Paninski L., et al. Instant neural control of a movement signal. Nature. 2002;416:141.
16. Truccolo W., Hochberg L.R., Donoghue J.P. Collective dynamics in human and monkey sensorimotor cortex: predicting single neuron spikes. Nat Neurosci. 13(1), 2010. 105-111
17. Donoghue J.P. Connecting cortex to machines: recent advances in brain interfaces. Nat Neurosci. 2002;5(suppl):1085-1088.
18. Park M.C., Zerris V., Hochberg L., et al. First experience with an implantable human neuro-motor prosthesis. Neuromodulation. 9(1), 2006. 19-20
19. Duncan D.E. Implanting hope. Technol Rev. 2005;March:69-74.
20. Haselager P., Vlek R., Hill J., et al. A note on ethical aspects of BCI. Neural Netw. 22(9), 2009. 1352-1357
21. Bakay R.A.E. Limits of brain–computer interface. Neurosurg Focus. 20(5), 2006. e6
22. Brown J.A., Lutsep H.L., Weinand M., et al. Motor cortex stimulation for the enhancement of recovery from stroke: a prospective, multicenter safety study. Neurosurgery. 58(3), 2006. 464-473
23. Frazer C, Power M, Hamdy S, et al. Driving plasticity in human adult motor cortex is associated with improved motor function after brain injury. Neuron 34:831-840, 202.
24. Enzinger C., Ropele S., Fazekas F., et al. Brain motor system function in a patient with complete spinal cord injury following extensive brain–computer interface training. Exp Brain Res. 90(2), 2008. 215-223
25. Wang W., Collinger J.L., Perez M.A., et al. Neural interface technology for rehabilitation: exploiting and promoting neuroplasticity. Phys Med Rehabil Clin North Am. 21(1), 2010. 157-178
26. Kubler A., Neumann N., Kaiser H., et al. Brain–computer communication: self-regulation of slow cortical potentials for verbal communication. Arch Phys Med Rehabil. 2001;82:1533-1539.
27. Pfurtscheller G., Guger C., Muller G., et al. Brain oscillations control hand orthosis in a tetraplegic. Neurosci Lett. 2000;292:211-214.
28. Wolpaw J.R., Birbaumer N., McFarland D.J., et al. Brain–computer interfaces for communication and control. Clin Neurophysiol. 2002;113:767-791.
29. Wolpaw J.R., McFarland D.J., Neat G.W., Forneris C.A. An EEG-based brain–computer interface for cursor control. Electroencephalogr Clin Neurophysiol. 1991;78:252-259.
30. Leuthardt E.C., Schalk G., Wolpaw J.R., et al. A brain–computer interface using electrocorticographic signals in humans. J Neural Eng. 2004;1:63-71.
31. Leuthardt E.C., Freudenberg Z., Bundy D., et al. Microscale recording from human motor cortex: implications for minimally invasive electrocorticographic brain–computer interfaces. Neurosurg Focus. 27(1), 2009. E10
32. Felton E.A., Wilson J.A., Williams J.C., et al. Electrocorticographically controlled brain–computer interfaces using motor and sensory imagery in patients with temporary subdural electrode implants. Report of four cases. J Neurosurg. 106(3), 2007. 495-500
33. McFarl D.J., Miner L.A., Vaughan T.M., et al. μ and β rhythm topographies during motor imagery and actual movements. Brain Topogr. 2000;12:177-186.
34. Kelly S.P., Lalor E.C., Reilly R.B., et al. Visual spatial attention tracking using high-density SSVEP data for independent brain–computer communication. IEEE Trans Neural Syst Rehabil Eng. 13(2), 2005. 172-8
35. Hinterberger T., Schmidt S., Neumann N., et al. Brain–computer communication and slow cortical potentials. IEEE Trans Biomed Eng. 51(6), 2004. 1011-1018
36. Loeb G.E., Walker A.E., Uematsu S., et al. Histological reaction to various conductive and dielectric films chronically implanted in the subdural space. J Biomed Mater Res. 1977;11:195-210.
37. Yeun T.G., Agnew W.F., Bullara L.A. Tissue response to potential neuroprosthetic materials implanted subdurally. Biomaterials. 1987;8:138-141.
38. Nijboer F., Sellers E.W., Mellinger J., et al. A P300-based brain–computer interface for people with amyotrophic lateral sclerosis. Clin Neurophysiol (Netherlands). 119(8), 2008. 1909-1916
39. Andersen R.A., Buneo C.A. Intentional maps in posterior parietal cortex. Ann Rev Neurosci. 2002;25:189-220.
40. Desmurget M., Reilly K.T., Richard N., et al. Movement intention after parietal cortex stimulation in humans. Science. 324(8), 2009. 811-813
41. Kennedy P.R., Kirby M.T., King B., et al. Computer control using human cortical local field potentials. IEEE Trans. Neural Syst. Rehab. Eng. 12(3), 2004. 339-344
42. Maynard E.M., Nordhausen C.T., Normann R.A. The Utah intercortical electrode array: a recording structure for potential brain–computer interfaces. Electroencephalogr Clin Neurophysiol. 1997;102:228-239.
43. Taylor D.M., Tillery S.I., Schwartz A.B. Direct cortical control of 3-D neuroprosthetic devices. Science. 2002;7:1829-1832.
44. Donoghue J.P. Bridging the brain to the world: a perspective on neural interface systems. Neuron. 60(3), 2008. 511-521
45. Kennedy P.R.. Comparing electrodes for use as cortical control signals: tiny tines, tiny wires, or tiny cones on wires: which is best?, 3rd ed. The Biomedical Engineering Handbook, Boca Raton, FL, CRS/Taylor and Francis, 2006 :vol. 1 Chapter 32-1
46. Polikov V.S., Block M.L., Fellous J.M., et al. In vitro model of glial scarring around neuroelectrodes chronically implanted in the CNS. Biomaterials. 27(31), 2006. 5368-5376
47. Vetter R.J., Williams J.C., Hetke J.F., et al. Chronic neural recording using silicon-substrate microelectrode arrays implanted in cerebral cortex. IEEE Trans Biomed Eng. 2004;51:896-904.
48. Kreiman G., Koch C., Fried I. Category specific visual responses of single neurons in the human medial temporal lobe. Nat Neurosci. 2000;3:946-953.
49. Nicolelis M.A., Dimitrov D., Carmena J.M., et al. Chronic, multisite, multielectrode recordings in macaque monkeys. Proc Natl Acad Sci U S A. 2003;100:11041-11046.
50. Moxon K.A., Kalkhoran N.M., Markert M., et al. Nanostructured surface modification of ceramic-based microelectrodes to enhance biocompatibility for a direct brain–machine interface. IEEE Trans Biomed Eng. 51(6), 2004. 881-889
51. Buzsaki G. Large-scale recordings of neuronal ensembles. Nat Neurosci. 2004;7:446-451.
52. Richardson-Burns S.M., Hendricks J.L., Martin D.C. Electrochemical polymerization of conducting polymers in living neural tissue. J Neural Eng (Engl). 4(2), 2007. L6-L13
53. Kim K.H., Moldovan N., Ke C., et al. Novel ultrananocrystalline diamond probes for high-resolution low-wear nanolithographic techniques. Small. 1(89), 2005. 866-874
54. Bajaj P., Akin D., Gupta A., et al. Ultrananocrystalline diamond film as an optimal cell interface for biomedical applications. Biomed Microdevices (US). 2007;9(6):787-794.
55. Shain W., Spataro L., Dilgen J., et al. Controlling cellular reactive responses around neural prosthetic devices using peripheral and local intervention strategies. IEEE Transneural Syst Rehabil Eng. 2003;11:186-188.
56. Benfey M., Aguayo A.J. Extensive elongation of axons from rat brain into peripheral nerve grafts. Nature. 1982;296:105-107.
57. Gillen C., Korfhage C., Muller H.W. Gene expression in nerve regeneration. Neuroscientist. 1997;3:112-122.
58. Bartels J.L., Andreasen D., Ehirim P., et al. Neurotrophic electrode: method of assembly and implantation into human motor speech cortex. J Neurosci Meth. 174(2), 2008. 168-176
59. Fetz E.E., Finnocchio D.V. Operant conditioning of specific patterns of neural and muscular activity. Science. 1971;174:431-435.
60. Fetz E.E., Baker M.A. Operantly conditioned patterns of precentral unit activity and correlated responses in adjacent cells and contralateral muscles. J Neurophysiol. 1973;36:179-204.
61. Burnod Y., Maton B., Calvet J. Short-term changes in cell activity of areas 4 and 5 during operant conditioning. Exp Neurol. 1982;78:227-240.
62. Wyler A.R., Lange S.C., Neafsey E.J., et al. Operant control of precentral neurons: control of model interspike intervals. Brain Res. 1980;190:29-38.
63. Wyler A.R. Interneuronal synchrony in precentral cortex of monkeys during operant conditioning. Exp Neurol. 1983;80:697-707.
64. Fetz E.E., Finnochio D.V., Baker M.A., et al. Sensory and motor responses of precentral cortex cells during comparable passive and active joint movements. J Neurophysiol. 43(4), 1980. 1070-1089
65. Wessberg J., Stambaugh C.R., Kralik J.D., et al. Real-time prediction of hand trajectory by ensembles of cortical neurons in primates. Nature. 2000;408:361-365.
66. Carmena J.M., Lebebev M.A., Crist R.E., et al. Learning to control a brain–machine interface for reaching and grasping by primates. PloS Biol. 2003;1(2):E42.
67. Lebedev M.A., Carmena J.M., O’Doherty J.E., et al. Cortical ensemble adaptation to represent velocity of an artificial actuator controlled by a brain–machine interface. J Neurosci. 25(9), 2005. 4681-4893
68. Musallam S., Corneil B.D., Greger B., et al. Cognitive control signals for neural prosthetics. Neuroscience. 2004;305:259-262.
69. Taylor D.M., Tillery S.I., Schwartz A.B. Direct cortical control of 3D neuroprosthetic devices. Science. 2002;296:1829-1832.
70. Santucci D.M., Kralik J.D., Lebedev M.A., et al. Frontal and parietal cortical ensembles predict single-trial muscle activity during reaching movements. Eur J Neurosci. 2005;22:1529-1540.
71. Wyler A.R., Burchiel K.J., Robbins C.A. Operant control of precentral neurons: evidence against open loop control. Brain Res. 1979;171:29-39.
72. Wyler A.R., Burchiel K.J. Operant control of pyramidal tract neurons: role of spinal dorsal. Brain Res. 157(2), 1978. 257-265
73. Schmidt E.M. Single neuron recording from motor cortex as a possible source of signals for control of external devices. Ann Biomed Eng. (8):1980. 339-349
74. Nowak D., Glasauer S. Hermsdorfer J: How predictive is grip force control in the complete absence of somatosensory feedback? Brain. 2003;127:182-192.
75. Kim H.K., Biggs S.J., Schloerb D.W., et al. Continuous shared control for stabilizing reaching and grasping with brain–machine interfaces. IEEE Trans Biomed Eng. 53(6), 2006. 1164-1173
76. MKh Hazrati, Erfanian A. An on-line BCI for control of hand grasp sequence and holding using adaptive probabilistic neural network. Conf Proc IEEE Eng Med Biol Soc. 2008;1009-:1012.
77. Hochberg L.R., Serruya M.D., Friehs G.M., et al. Neuronal ensemble control of prosthetic devices by a human with tetraplegia. Nature. 2006;442:164-171.
78. Lacourse M.G., Cohen M.J., Lawrence K.E., et al. Cortical potentials during imagined movements in individuals with chronic spinal cord injuries. Behav Brain Res. 104(12), 1999. 73-88
79. Neuper C., Scherer R., Wriessnegger S., et al. Motor imagery and action observation: modulation of sensorimotor brain rhythms during mental control of a brain–computer interface. Clin Neurophysiol. 120(2), 2009. 239-247
80. Gandolfo F., Li C., Benda B.J., et al. Cortical correlates of learning in monkeys adapting to a new dynamical environment. Proc Natl Acad Sci U S A. 2000;97:2259-2263.
81. Nudo R.J., Milliken G.W., Kenkins W.M., et al. Use-dependent alterations of movement representations in primary motor cortex of adult squirrel monkeys. J Neurosci. 1996;16:785-807.
82. Fetz E.E. Volitional control of neural activity: implications for brain–computer interfaces. J Physiol (Lond). 2007;579:571-579.
83. Fraser G.W., Chase S.M., Whitford A., et al. Control of a brain–computer interface without spike sorting. J Neural Eng. 6(5), 2009. 055004
84. Fountas K.N., Smith J.R., Murro A.M., et al. Implantation of a closed-loop stimulation in the management of medically refractory focal epilepsy: a technical note. Stereotact Funct Neurosurg. 83(4), 2005. 153-158
85. Morrell M. Brain stimulation for epilepsy: can scheduled or responsive neurostimulation stop seizures? Curr Opin Neurol. 19(2), 2006. 164-168
86. Romo R., Hernandez A., Zainos A., et al. Sensing without ouching: psychophysical performance based on cortical microstimulation. Neuron. 2000;26:273-278.
87. Wickersham I., Groh J.M. Neurophysiology: electrically evoking sensory experience. Curr Biol. 1998;8:R412-R414.
88. O’Doherty J.E., Lebedev M.A., Hanson T.L., et al. A brain–machine interface instructed by direct intracortical microstimulation. Front Integr Neurosci. 2009;3:20.
89. Horgan J. The myth of mind control. Discover. 28(10), 2004. 40-47
90. Guenther F.H., Brumberg J.S., Wright E.J., et al. A wireless brain–machine interface for real-time speech synthesis. PLoS ONE. 4(12), 2009. e8218
91. Patil P.G., Carmena J.M., Nicolelis M.A.L., et al. Ensemble recordings of human subcortical neurons as a source of motor control signals for a brain–machine interface. Neurosurgery. 55(1), 2003. 27-35
92. Vitek J.L., Bakay R.A.E., Hashimoto T., et al. Microelectrode-guided pallidotomy: technical approach and its application in medically intractable Parkinson’s disease. J Neurosurg. 88(6), 1998. 1027-1043
93. Ehrlenspiel F., Wei K., Sternad D. Open-loop, closed-loop and compensatory control: performance improvement under pressure in a rhythmic task. Exp Brain Res. 2010;201:729-741.
94. Song Y.K., Borton D.A., Park S. Active microelectronic neurosensor arrays for implantable brain communication interfaces. IEEE Trans Neural Syst Rehabil Eng. 17(4), 2009. 339-345
95. Mavoori J., Jackson A., Diorio C., et al. An autonomous implantable computer for neural recording and stimulation in unrestrained primates. J Neurosci Methods. 2005;148:71.
96. Roy S., Ferrara L.A., Fleischman A.J., et al. Microelectromechanical systems and neurosurgery: a new era in a new millennium. Neurosurgery. 49(4), 2001. 779-797
97. Leuthardt E.C., Schalk C., Moran D., et al. The emerging world of motor neuroprosthetics: a neurosurgical perspective. Neurosurgery. 2006;59:1-14.
98. Gao Y., Black M.J., Bienenstock E., et al. Probabilistic inference of hand motion from neural activity in motor cortex. Adv Neural Info Processing Syst. 2002;14:213-220.
99. Helms Tillery S.I., Taylor D.M., Schwartz A.B. Training in cortical of neuroprosthetic devices improves signal extraction from small neuronal ensembles. Rev Neurosci. 2003;14:107-119.
100. Serruya M.D., Hatsopoulos N.G., Fellows M.R., et al. Robustness of neuroprosthetic decoding algorithms. Biol Cybern. 88(3), 2003. 219-228
101. Pancrazio J.J. National Institute of Neurological Disorders and Stroke support for brain–machine interface technology. Neurosurg Focus. 27(1), 2009. E14
102. Olson L. Regeneration in the adult central nervous system: experimental repair strategies. Nat Med. 3, 1997. (12):1329-1335
103. Woerly S. Restorative surgery of the central nervous system by means of tissue engineering using NeuroGel implants. Neurosurg Rev. 23(2), 2000. 59-77
104. Nagappan G., Zaitsev E., Senatorov V.V., et al. Control of extracellular cleavage of ProBDNF by high frequency neuronal activity. Proc Natl Acad Sci U S A. 106(4), 2009. 1267-1272
105. Palanker D., Huie P., Vankov A., et al. Migration of retinal cells through a perforated membrane: implications for a high-resolution prosthesis. Invest Ophthalmol Vis Sci. 45(9), 2004. 3266-3270
106. Wilms C.D., Hausser M. Lighting up neural networks using a new generation of genetically encoded calcium sensors. Nat Methods. 6(12), 2009. 871-872
107. Aravanis A.M., Wang L.P., Zhang F., et al. An optical neural interface: in vivo control of rodent motor cortex with integrated fiberoptic and optogenetic technology. J Neural Eng. 4(3), 2007. 143-S156
108. Wang H., Peca J., Matsuzaki M. High-speed mapping of synaptic connectivity using photostimulation in Channelrhodopsin-2 transgenic mice. Proc Natl Acad Sci U S A. 104(19), 2007. 8143-8148
109. Gradinaru V., Mogri M., Thompson K.R., et al. Optical deconstruction of parkinsonian neural circuitry. Science. 324(5925), 2009. 354-359
110. Shachtman N. Pentagon’s PCs Bend to Your Brain. Wired Magazine. March 21, 2007. Available at http://www.wired.com/dangerroom/2007/03/the_us_military
111. Drummond K. Pentagon Preps Soldier Telepathy Push. Wired Magazine. May 14, 2009. Available at http://www.wired.com/dangerroom/2009/05/pentagon-preps-soldier-telepathy-push