Table 24-1
Cancer Gene Census Summary
Aberration Type | Number of Aberrations | Examples of Prominent Affected Genes |
Amplification | 16 | ERBB2, EGFR, MYCN, MDM2, CCND1 |
Frameshift mutation | 100 | APC, RB1, ATM, MLH1, NF1 |
Germline mutation | 76 | BRCA1/2, TP53, ERCC2, RB1, VHL |
Missense mutation | 141 | ARID1A, ATM, PIK3CA, IDH1, KRAS |
Nonsense mutation | 92 | CDKN2A, FANCA, PTCH, PTEN |
Other mutation | 26 | BRAF, PDGFRA, PIK3R1, SOCS1 |
Splicing mutation | 63 | GATA3, MEN1, MSH2, TSC1 |
Translocation | 326 | ABL1, ALK, BCL2, TMPRSS2, MYC |
For more details see http://www.sanger.ac.uk/genetics/CGP/Census.
Table 24-2
Candidate Cancer Hallmark–Associated Aberrant Genes
Cancer Hallmark | Aberrant Gene |
Resisting cell death | BCL2, BAX, FAS |
Genome instability and mutation | TP53, BRCA1/2, MLH1 |
Inducing angiogenesis | CCK2R |
Activating invasion and metastasis | ADAMTSL4, ADAMTS3 |
Tumor-promoting inflammation | IL32 |
Enabling replicative immortality | TERT |
Avoiding immune destruction | HLA loci, TAP1/2, B2M |
Evading growth suppressors | RB1, CCND1, CDKN2A |
Sustaining proliferative signaling | KRAS, ERBB2, MYC |
Deregulating cellular energetics | PIK3CA, PTEN |
Functional Assessment of Cancer Genomes
Computational Approaches
Cataloging Approaches
Integrating Information
Organization into Pathways
Experimental Approaches
Tumor Intrinsic Assessments
Interaction with the Microenvironment
Clinical Applications
Diagnosis and Detection
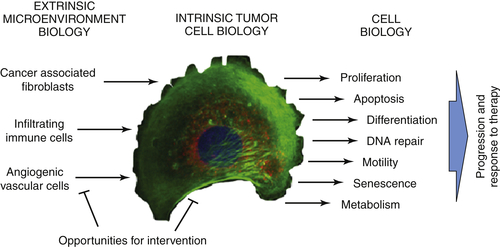
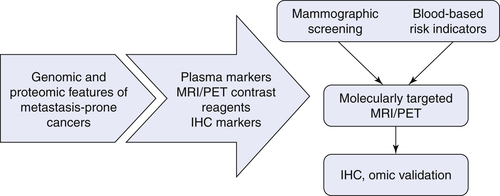
Therapeutic Targets and Predictive Markers
Table 24-3
Genomic Aberrations, Therapeutic Agents, and Relevant Cancers 154
ALL, Acute lymphocytic leukemia; AML, acute myeloid leukemia; CML, chronic myelogenous leukemia; GIST, gastrointestinal stromal tumor.
Summary
1. The transcriptional coactivator TAZ regulates mesenchymal differentiation in malignant glioma . Genes Dev . 2011 ; 25 : 2594 – 2609 .
2. . Comprehensive genomic characterization defines human glioblastoma genes and core pathways . Nature . 2008 ; 455 : 1061 – 1068 .
3. . Integrated genomic analyses of ovarian carcinoma . Nature . 2011 ; 474 : 609 – 615 .
4. . Comprehensive molecular characterization of human colon and rectal cancer . Nature . 2012 ; 487 : 330 – 337 .
5. . Comprehensive genomic characterization of squamous cell lung cancers . Nature . 2012 ; 489 : 519 – 525 .
6. . Comprehensive molecular portraits of human breast tumours . Nature . 2012 ; 490 : 61 – 70 .
7. The genomic landscapes of human breast and colorectal cancers . Science . 2007 ; 318 : 1108 – 1113 .
8. The clonal and mutational evolution spectrum of primary triple-negative breast cancers . Nature . 2012 ; 486 : 395 – 399 .
9. Genomic architecture characterizes tumor progression paths and fate in breast cancer patients . Sci Transl Med . 2010 ; 2 38ra47 .
10. Complex landscapes of somatic rearrangement in human breast cancer genomes . Nature . 2009 ; 462 : 1005 – 1010 .
11. Core signaling pathways in human pancreatic cancers revealed by global genomic analyses . Science . 2008 ; 321 : 1801 – 1806 .
12. Transcriptome sequencing across a prostate cancer cohort identifies PCAT-1, an unannotated lincRNA implicated in disease progression . Nat Biotechnol . 2011 ; 29 : 742 – 749 .
13. Genome and transcriptome sequencing of lung cancers reveal diverse mutational and splicing events . Genome Res . 2012 ; 22 : 2315 – 2327 .
14. Systematic sequencing of renal carcinoma reveals inactivation of histone modifying genes . Nature . 2010 ; 463 : 360 – 363 .
15. Melanoma genome sequencing reveals frequent PREX2 mutations . Nature . 2012 ; 485 : 502 – 506 .
16. Initial genome sequencing and analysis of multiple myeloma . Nature . 2011 ; 471 : 467 – 472 .
17. Clonal evolution in relapsed acute myeloid leukaemia revealed by whole-genome sequencing . Nature . 2012 ; 481 : 506 – 510 .
18. Translating insights from the cancer genome into clinical practice . Nature . 2008 ; 452 : 553 – 563 .
19. Hallmarks of cancer: the next generation . Cell . 2011 ; 144 : 646 – 674 .
20. Functional genomic analysis of chromosomal aberrations in a compendium of 8000 cancer genomes . Genome Res . 2013 ; 23 : 217 – 227 .
21. The genomic and transcriptomic architecture of 2,000 breast tumours reveals novel subgroups . Nature . 2012 ; 486 : 346 – 352 .
22. The genetic landscape of high-risk neuroblastoma . Nat Genet . 2013 ; 45 : 279 – 284 .
23. A landscape of driver mutations in melanoma . Cell . 2012 ; 150 : 251 – 263 .
24. In situ analyses of genome instability in breast cancer . Nat Genet . 2004 ; 36 : 984 – 988 .
25. Telomere dysfunction promotes non-reciprocal translocations and epithelial cancers in mice . Nature . 2000 ; 406 : 641 – 645 .
26. Cancer genetics and epigenetics: two sides of the same coin? Cancer Cell . 2012 ; 22 : 9 – 20 .
27. p53: good cop/bad cop . Cell . 2002 ; 110 : 9 – 12 .
28. Generation of mutator mutants during carcinogenesis . DNA Repair (Amst) . 2006 ; 5 : 294 – 302 .
29. The cancer epigenome—components and functional correlates . Genes Dev . 2006 ; 20 : 3215 – 3231 .
30. Somatic mutations of the protein kinase gene family in human lung cancer . Cancer Res . 2005 ; 65 : 7591 – 7595 .
31. A small-cell lung cancer genome with complex signatures of tobacco exposure . Nature . 2010 ; 463 : 184 – 190 .
32. Distant metastasis occurs late during the genetic evolution of pancreatic cancer . Nature . 2010 ; 467 : 1114 – 1117 .
33. Temporal dissection of tumorigenesis in primary cancers . Cancer Discov . 2011 ; 1 : 137 – 143 .
34. Genetic alterations during colorectal-tumor development . N Engl J Med . 1988 ; 319 : 525 – 532 .
35. A mathematical framework to determine the temporal sequence of somatic genetic events in cancer . Proc Natl Acad Sci U S A . 2010 ; 107 : 17604 – 17609 .
36. A mathematical methodology for determining the temporal order of pathway alterations arising during gliomagenesis. PLoS Comput Biol. 8, e1002337 . 2012 .
37. The life history of 21 breast cancers . Cell . 2012 ; 149 : 994 – 1007 .
38. Histone onco-modifications . Oncogene . 2011 ; 30 : 3391 – 3403 .
39. Colorectal cancer epigenetics: complex simplicity . J Clin Oncol . 2011 ; 29 : 1382 – 1391 .
40. Chromatin and the DNA damage response: the cancer connection . Mol Oncol . 2011 ; 5 : 349 – 367 .
41. Fast and accurate short read alignment with Burrows-Wheeler transform . Bioinformatics . 2009 ; 25 : 1754 – 1760 .
42. An integrated map of genetic variation from 1,092 human genomes . Nature . 2012 ; 491 : 56 – 65 .
43. Pindel: a pattern growth approach to detect break points of large deletions and medium sized insertions from paired-end short reads . Bioinformatics . 2009 ; 25 : 2865 – 2871 .
44. BreakDancer: an algorithm for high-resolution mapping of genomic structural variation . Nat Methods . 2009 ; 6 : 677 – 681 .
45. DELLY: structural variant discovery by integrated paired-end and split-read analysis . Bioinformatics . 2012 ; 28 : i333 – i339 .
46. Differential gene and transcript expression analysis of RNA-seq experiments with TopHat and Cufflinks . Nat Protoc . 2012 ; 7 : 562 – 578 .
47. MicroRNA profiling of clear cell renal cell carcinoma by whole-genome small RNA deep sequencing of paired frozen and formalin-fixed, paraffin-embedded tissue specimens . J Pathol . 2010 ; 222 : 41 – 51 .
48. Strategies to identify long noncoding RNAs involved in gene regulation . Cell Biosci . 2012 ; 2 : 37 .
49. DNA replication timing and higher-order nuclear organization determine single-nucleotide substitution patterns in cancer genomes . Nat Commun . 2013 ; 4 : 1502 .
50. Circular binary segmentation for the analysis of array-based DNA copy number data . Biostatistics . 2004 ; 5 : 557 – 572 .
51. STAC: A method for testing the significance of DNA copy number aberrations across multiple array-CGH experiments . Genome Res . 2006 ; 16 : 1149 – 1158 .
52. The landscape of somatic copy-number alteration across human cancers . Nature . 2010 ; 463 : 899 – 905 .
53. End-sequence profiling: sequence-based analysis of aberrant genomes . Proc Natl Acad Sci U S A . 2003 ; 100 : 7696 – 7701 .
54. Sensitive detection of somatic point mutations in impure and heterogeneous cancer samples . Nat Biotechnol . 2013 ; 31 : 213 – 219 .
55. Functional impact bias reveals cancer drivers . Nucleic Acid Res . 2012 ; 40 : e169 .
56. Systematic and integrative analysis of large gene lists using DAVID bioinformatics resources . Nat Protoc . 2009 ; 4 : 44 – 57 .
57. Gene set enrichment analysis: a knowledge-based approach for interpreting genome-wide expression profiles . Proc Natl Acad Sci U S A . 2005 ; 102 : 15545 – 15550 .
58. The PageRank citation ranking: bringing order to the web . http://infolab.stanford.edu/∼backrub/pageranksub.ps 1999 .
59. A novel signaling pathway impact analysis . Bioinformatics . 2009 ; 25 : 75 – 82 .
60. A human B-cell interactome identifies MYB and FOXM1 as master regulators of proliferation in germinal centers . Mol Syst Biol . 2010 ; 6 : 377 .
61. GeneRank: using search engine technology for the analysis of microarray experiments . BMC Bioinformatics . 2005 ; 6 : 233 .
62. REFERENCE DELETED IN PROOFS.
63. The BioGRID interaction database: 2013 update . Nucleic Acid Res . 2013 ; 41 : D816 – D823 .
64. Human Proteinpedia enables sharing of human protein data . Nat Biotechnol . 2008 ; 26 : 164 – 167 .
65. iRefWeb: interactive analysis of consolidated protein interaction data and their supporting evidence . Database (Oxford) . 2010 baq023 .
66. STRING v9.1: protein-protein interaction networks, with increased coverage and integration . Nucleic Acid Res . 2013 ; 41 : D808 – D815 .
67. Reactome knowledgebase of human biological pathways and processes . Nucleic Acid Res . 2009 ; 37 : D619 – D622 .
68. PID: the Pathway Interaction Database . Nucleic Acid Res . 2009 ; 37 : D674 – D679 .
69. Algorithms for detecting significantly mutated pathways in cancer . J Comput Biol . 2011 ; 18 : 507 – 522 .
69a. . Comprehensive molecular characterization of clear cell renal cell carcinoma . Nature . 2013 ; 499 : 43 – 49 .
70. Mutual exclusivity analysis identifies oncogenic network modules . Genome Res . 2012 ; 22 : 398 – 406 .
71. De novo discovery of mutated driver pathways in cancer . Genome Res . 2012 ; 22 : 375 – 385 .
72. Inference of patient-specific pathway activities from multi-dimensional cancer genomics data using PARADIGM . Bioinformatics . 2010 ; 26 : i237 – i245 btq182 [pii]10.1093/bioinformatics/btq182 .
73. Subtype and pathway specific responses to anticancer compounds in breast cancer . Proc Natl Acad Sci U S A . 2012 ; 109 : 2724 – 2729 .
74. PARADIGM-SHIFT predicts the function of mutations in multiple cancers using pathway impact analysis . Bioinformatics . 2012 ; 28 : i640 – i646 .
75. Network-induced classification kernels for gene expression profile analysis . J Comput Biol . 2012 ; 19 : 694 – 709 .
76. A collection of breast cancer cell lines for the study of functionally distinct cancer subtypes . Cancer Cell . 2006 ; 10 : 515 – 527 S1535-6108060314-X [pii]10.1016/j.ccr. 2006.10.008 .
77. The Cancer Cell Line Encyclopedia enables predictive modelling of anticancer drug sensitivity . Nature . 2012 ; 483 : 603 – 607 .
78. High-throughput sequence analysis of the tyrosine kinome in acute myeloid leukemia . Blood . 2008 ; 111 : 4788 – 4796 .
79. Ba/F3 cells and their use in kinase drug discovery . Curr Opin Oncol . 2007 ; 19 : 55 – 60 .
80. Human tumor xenografts as predictive preclinical models for anticancer drug activity in humans: better than commonly perceived-but they can be improved . Cancer Biol Ther . 2003 ; 2 : S134 – S139 .
81. Contributions of human tumor xenografts to anticancer drug development . Cancer Res . 2006 ; 66 : 3351 – 3354 discussion 3354 .
82. A murine lung cancer co-clinical trial identifies genetic modifiers of therapeutic response . Nature . 2012 ; 483 : 613 – 617 .
83. The APL paradigm and the “co-clinical trial” project . Cancer Discov . 2011 ; 1 : 108 – 116 .
84. Harnessing transposons for cancer gene discovery . Nat Rev Cancer . 2010 ; 10 : 696 – 706 .
85. RNAi in cultured mammalian cells using synthetic siRNAs . Cold Spring Harb Protoc . 2012 : 957 – 961 .
86. A pipeline for the generation of shRNA transgenic mice . Nat Protoc . 2012 ; 7 : 374 – 393 .
87. Production of artificial piRNAs in flies and mice . RNA . 2012 ; 18 : 42 – 52 .
88. Second-generation shRNA libraries covering the mouse and human genomes . Nat Genet . 2005 ; 37 : 1281 – 1288 .
89. A large-scale RNAi screen in human cells identifies new components of the p53 pathway . Nature . 2004 ; 428 : 431 – 437 .
90. A system for stable expression of short interfering RNAs in mammalian cells . Science . 2002 ; 296 : 550 – 553 .
91. High-throughput RNA interference screening using pooled shRNA libraries and next generation sequencing . Genome Biol . 2011 ; 12 : R104 .
92. Systematic investigation of genetic vulnerabilities across cancer cell lines reveals lineage-specific dependencies in ovarian cancer . Proc Natl Acad Sci U S A . 2011 ; 108 : 12372 – 12377 .
93. A cell spot microarray method for production of high density siRNA transfection microarrays . BMC Genomics . 2011 ; 12 : 162 .
94. Systematic knockdown of epigenetic enzymes identifies a novel histone demethylase PHF8 overexpressed in prostate cancer with an impact on cell proliferation, migration and invasion . Oncogene . 2012 ; 31 : 3444 – 3456 .
95. High-content siRNA screening for target identification and validation . Expert Opin Drug Discov . 2008 ; 3 : 551 – 564 .
96. High-content siRNA screening . Mol Biosyst . 2007 ; 3 : 232 – 240 .
97. High-throughput RNAi screening by time-lapse imaging of live human cells . Nat Methods . 2006 ; 3 : 385 – 390 .
98. Synthetic lethal approaches to breast cancer therapy . Nat Rev Clin Oncol . 2010 ; 7 : 718 – 724 .
99. A synthetic lethal siRNA screen identifying genes mediating sensitivity to a PARP inhibitor . EMBO J . 2008 ; 27 : 1368 – 1377 .
100. Large-scale cDNA transfection screening for genes related to cancer development and progression . Proc Natl Acad Sci U S A . 2004 ; 101 : 15724 – 15729 .
101. Deciphering the genetic landscape of cancer—from genes to pathways . Trends Genet . 2009 ; 25 : 455 – 462 .
102. Integrating data on DNA copy number with gene expression levels and drug sensitivities in the NCI-60 cell line panel . Mol Cancer Ther . 2006 ; 5 : 853 – 867 .
103. Systematic identification of genomic markers of drug sensitivity in cancer cells . Nature . 2012 ; 483 : 570 – 575 .
104. EGFR mutations in lung cancer: correlation with clinical response to gefitinib therapy . Science . 2004 ; 304 : 1497 – 1500 .
105. Activity of the dual kinase inhibitor lapatinib (GW572016) against HER-2-overexpressing and trastuzumab-treated breast cancer cells . Cancer Res . 2006 ; 66 : 1630 – 1639 .
106. Changes associated with the development of resistance to imatinib (STI571) in two leukemia cell lines expressing p210 Bcr/Abl protein . Cancer . 2004 ; 100 : 1459 – 1471 .
107. Characterization of a large panel of patient-derived tumor xenografts representing the clinical heterogeneity of human colorectal cancer . Clin Cancer Res . 2012 ; 18 : 5314 – 5328 .
108. A human breast cell model of preinvasive to invasive transition . Cancer Res . 2008 ; 68 : 1378 – 1387 .
109. The VEGF pathway in cancer and disease: responses, resistance, and the path forward . Cold Spring Harb Perspect Med . 2012 ; 2 : a006593 . doi: 10.1101/cshperspect.a006593 .
110. Accessories to the crime: functions of cells recruited to the tumor microenvironment . Cancer Cell . 2012 ; 21 : 309 – 322 .
111. Modeling dynamic reciprocity: engineering three-dimensional culture models of breast architecture, function, and neoplastic transformation . Semin Cancer Biol . 2005 ; 15 : 342 – 352 .
112. Modelling glandular epithelial cancers in three-dimensional cultures . Nat Rev Cancer . 2005 ; 5 : 675 – 688 .
113. Fabrication and use of microenvironment microarrays (MEArrays) . J Vis Exp . 2012 ( 68 ) .
114. Widespread potential for growth-factor-driven resistance to anticancer kinase inhibitors . Nature . 2012 ; 487 : 505 – 509 .
115. Reconstruction of functionally normal and malignant human breast tissues in mice . Proc Natl Acad Sci U S A . 2004 ; 101 : 4966 – 4971 .
116. Mouse Models of Human Cancers Consortium (MMHCC) from the NCI . Dis Model Mech . 2009 ; 2 : 111 .
117. PAM50 assay and the three-gene model for identifying the major and clinically relevant molecular subtypes of breast cancer . Breast Cancer Res Treat . 2012 ; 135 : 301 – 306 .
118. Molecular portraits of human breast tumours . Nature . 2000 ; 406 : 747 – 752 .
119. The landscape of cancer genes and mutational processes in breast cancer . Nature . 2012 ; 486 : 400 – 404 .
120. . Oncology (Williston Park) . 2006 ; 20 : 789 – 790 .
121. Commercialized multigene predictors of clinical outcome for breast cancer . Oncologist . 2008 ; 13 : 477 – 493 .
122. Converting a breast cancer microarray signature into a high-throughput diagnostic test . BMC Genomics . 2006 ; 7 : 278 .
123. Molecular diagnosis of Burkitt’s lymphoma . N Engl J Med . 2006 ; 354 : 2431 – 2442 .
124. Transcriptional profiles predict disease outcome in patients with cutaneous T-cell lymphoma . Clin Cancer Res . 2010 ; 16 : 2106 – 2114 .
125. Gene expression profiling in colorectal cancer using microarray technologies: results and perspectives . Cancer Treat Rev . 2009 ; 35 : 201 – 209 .
126. Subtypes of pancreatic ductal adenocarcinoma and their differing responses to therapy . Nat Med . 2011 ; 17 : 500 – 503 nm.2344 [pii]10.1038/nm.2344 .
127. An immune response enriched 72-gene prognostic profile for early-stage non-small-cell lung cancer . Clin Cancer Res . 2009 ; 15 : 284 – 290 .
128. miR-103/107 promote metastasis of colorectal cancer by targeting the metastasis suppressors DAPK and KLF4 . Cancer Res . 2012 ; 72 : 3631 – 3641 .
129. Role of microRNAs in lung cancer: microRNA signatures in cancer prognosis . Cancer J . 2012 ; 18 : 268 – 274 .
130. Predicting progression of bladder urothelial carcinoma using microRNA expression . BJU Int . 2013 ; 112 : 1027 – 1034 .
131. Prospective gene signature study using microRNA to identify the tissue of origin in patients with carcinoma of unknown primary . Clin Cancer Res . 2011 ; 17 : 4063 – 4070 .
132. Molecular classification of unknown primary cancer . Semin Oncol . 2009 ; 36 : 38 – 43 .
133. Immune signatures predict prognosis in localized cancer . Cancer Invest . 2010 ; 28 : 765 – 773 .
134. Leukocyte complexity predicts breast cancer survival and functionally regulates response to chemotherapy . Cancer Discov . 2011 ; 1 : 54 – 67 .
135. A human proteome detection and quantitation project . Mol Cell Proteomics . 2009 ; 8 : 883 – 886 .
136. Lectin chromatography/mass spectrometry discovery workflow identifies putative biomarkers of aggressive breast cancers . J Proteome Res . 2012 ; 11 : 2508 – 2520 .
137. Detection of promoter hypermethylation in salivary rinses as a biomarker for head and neck squamous cell carcinoma surveillance . Clin Cancer Res . 2011 ; 17 : 4782 – 4789 .
138. Analysis of mutations in DNA isolated from plasma and stool of colorectal cancer patients . Gastroenterology . 2008 ; 135 : 489 – 498 .
139. Noninvasive identification and monitoring of cancer mutations by targeted deep sequencing of plasma DNA . Sci Transl Med . 2012 ; 4 136ra168 .
140. The discovery and application of gene fusions in prostate cancer . BJU Int . 2008 ; 102 : 276 – 282 .
141. ESRRA-C11orf20 is a recurrent gene fusion in serous ovarian carcinoma . PLoS Biol . 2011 ; 9 e1001156 .
142. Hybrid PET-optical imaging using targeted probes . Proc Natl Acad Sci U S A . 2010 ; 107 : 7910 – 7915 .
143. Synthesis and biological evaluation of two agents for imaging estrogen receptor beta by positron emission tomography: challenges in PET imaging of a low abundance target . Nucl Med Biol . 2012 ; 39 : 1105 – 1116 .
144. Noninvasive measurement of androgen receptor signaling with a positron-emitting radiopharmaceutical that targets prostate-specific membrane antigen . Proc Natl Acad Sci U S A . 2011 ; 108 : 9578 – 9582 .
145. In vivo imaging in cancer . Cold Spring Harb Perspect Biol . 2010 ; 2 a003848 .
146. Annotating MYC status with 89Zr-transferrin imaging . Nat Med . 2012 ; 18 : 1586 – 1591 .
147. Immuno-PET of the hepatocyte growth factor receptor Met using the 1-armed antibody onartuzumab . J Nucl Med . 2012 ; 53 : 1592 – 1600 .
148. Topographic enhancement mapping of the cancer-associated breast stroma using breast MRI . Integr Biol (Camb) . 2011 ; 3 : 490 – 496 .
149. Prognostic value of a combined estrogen receptor, progesterone receptor, Ki-67, and human epidermal growth factor receptor 2 immunohistochemical score and comparison with the Genomic Health recurrence score in early breast cancer . J Clin Oncol . 2011 ; 29 : 4273 – 4278 .
150. Quantitative in situ measurement of estrogen receptor mRNA predicts response to tamoxifen . PLoS One . 2012 ; 7 e36559 .
151. The genetic basis for cancer treatment decisions . Cell . 2012 ; 148 : 409 – 420 .
152. Efficacy and safety of a specific inhibitor of the BCR-ABL tyrosine kinase in chronic myeloid leukemia . N Engl J Med . 2001 ; 344 : 1031 – 1037 .
153. Pegram MD, et al. Phase II study of receptor-enhanced chemosensitivity using recombinant humanized anti-p185HER2/neu monoclonal antibody plus cisplatin in patients with HER2/neu-overexpressing metastatic breast cancer refractory to chemotherapy treatment. J Clin Oncol. 2098;16:2659-2671.
154. An emerging toolkit for targeted cancer therapies . Genome Res . 2012 ; 22 : 177 – 182 .
155. http://www.phrma.org/sites/default/files/1000/medicinesindevelopmentcancer2011_0.pdf 2011 .
156. Targeted cancer therapy . Nature . 2004 ; 432 : 294 – 297 .
157. Pathological complete response and accelerated drug approval in early breast cancer . N Engl J Med . 2012 ; 366 : 2438 – 2441 .
158. Potential of selective estrogen receptor modulators as treatments and preventives of breast cancer . Anticancer Agents Med Chem . 2009 ; 9 : 481 – 499 .
159. Targeting the androgen receptor . Urol Clin North Am . 2012 ; 39 : 453 – 464 .
160. PMID:24071849.