Figure 23-1 PCR and capillary sequencing of exons A generalized workflow is shown for the PCR-based amplification of specific exons in the human genome, their sequencing, and separation of the nucleotide sequence by a fluorescent capillary sequencing approach. Nucleotide changes can be identified by the appropriate software, such as PolyPhred, which was widely used for analysis of capillary data. A single base substitution mutation is shown in the trace data example. Pros and cons of the approach are listed and described in the text.
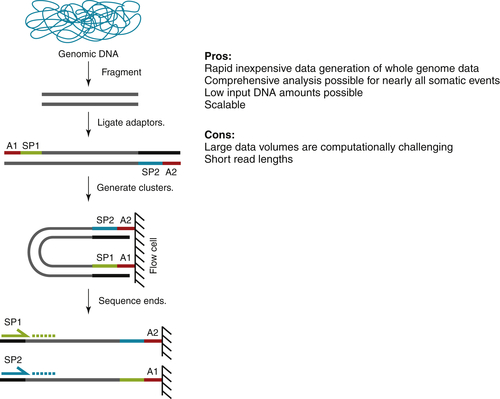
Figure 23-2 Next-generation sequencing (NGS) of whole genomes A generalized workflow for the production of whole-genome sequencing data by next-generation or massively parallel sequencing is shown. Several pros and cons of this approach are listed and described in the text. Computational identification of the genome-wide differences between tumor and normal genomes requires highly specialized pipelines for each variant type (point mutation, copy number alteration, insertion/deletion variant, structural variant).
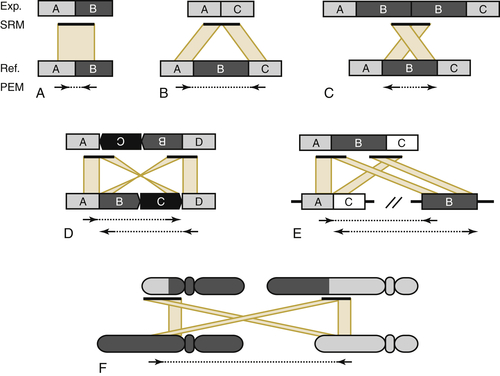
Figure 23-3 Read placement distance and orientation is indicative of structural variation of various types In each figure, the paired end mapping (PEM) orientation and distance on the reference genome (Ref) of the NGS data is shown relative to the short read mapping (SRM) of the experimental data (Exp). (A) The anticipated read mapping distance and orientation are shown. (B) A deletion in the experimental genome. (C) An insertion. (D) An inversion. (E) A complex rearrangement. (F) A translocation. With permission from Quinlan AR, Hall IM. Characterizing complex structural variation in germline and somatic genomes. Trends Genet. 2012;28:43-53.
Challenges to NGS Analysis of Cancer Nucleic Acids
The search for somatic variation in cancer DNA and RNA has a distinct advantage over other complex diseases: The exact comparison of tumor to normal nucleic acids within an individual patient distinctly identifies those alterations that are tumor unique. Furthermore, there are increasing amounts of data from various projects that have begun using NGS methods to catalog large numbers of cancer cases across different tumor types (ICGC [icgc.org], TCGA [cancergenome.nih.gov], PCGP [www.pediatriccancergenomeproject.org] 21 that can be used to inform individual analyses about previously described alterations. In spite of these decided advantages, there are several significant challenges that confound experimental design and analytical approaches in cancer genomics studies. Several examples of these challenges are described next, along with the ways researchers attempt to overcome them, where applicable.
Tumor Cellularity
Cancerous cells in solid tumors do not exist in isolation in the body. Rather, they are always in close proximity to normal cells of various types, including stromal cells, immune cells, and components known as the extracellular matrix (ECM). The proportion of tumor cells can be estimated by an experienced pathologist examining the tumor section under hematoxylin and eosin staining, and this estimate is expressed as a “percent tumor nuclei” or “percent tumor cellularity” value. As a result of the association of tumor and normal cells, an isolate of DNA or RNA derived from a solid cancer sample will contain both tumor and normal cells unless a specific procedure such as flow cytometry or laser capture microdissection (LCM) is used first to significantly enrich the percentage of tumor cells in the isolate. Also, certain tumor types, such as those from prostate or pancreas, are more prone to low tumor cellularity. Based on the pathology estimate, decisions in sequencing must be made in the context of tumor cellularity percentages. Namely, if the tumor cellularity is below 60%, the decision must be made either to enrich the tumor by flow cytometry (more common for blood cancers such as lymphoma or leukemia) or by LCM (used for solid tumors), or to try oversampling the tumor NGS library (increased sequencing coverage) by an amount commensurate with the tumor cellularity estimate. Although sorting or LCM seems the most obvious choice, one limitation of either approach is that significantly reduced yields of DNA or RNA will be obtained. Unless specialized procedures are in hand, the low yield may limit the ability to derive high-quality data from such samples. By contrast, oversampling may be effective for DNA sequencing but will be more expensive to generate and will require adjustment of variant calling parameters, or use of a more sensitive variant caller, to effectively identify somatic variants. Oversampling for RNA-seq from a sample with low tumor cellularity is generally not advised, as the tumor transcripts will be too difficult to discern from those of the normal cells unless LCM or sorting is first used to separate the tumor cells from the adjacent normal/nonmalignant cells.
Heterogeneity (Regional versus Genotypic)
Heterogeneity is a fundamental aspect of cancer cells found within the same tumor of which there are two types, regional and genotypic. Regional heterogeneity reflects the differences that emerge in solid tumors as they grow and progress. It refers to the different regions present in a tumor mass, such as areas of necrosis or areas of invasion (of surrounding normal tissue). Genotypic heterogeneity reflects the fact that cancer cells evolve during the process of tumor progression, so that not all tumor cells share the same somatic genotype. In genotypic heterogeneity, the use of NGS has demonstrated that by comparing the genomes from progression samples (a de novo leukemia compared to its relapse) using high-depth sequencing of somatic mutations, an initiating or “founder” clone can be identified that contains the core mutational load that initiates tumor growth as well as more advanced clones that combine newer mutations with those in the founder clone. 22 One shared aspect of regional and genotypic heterogeneity is that as a tumor mass increases in size, both are more likely to occur in that areas of regional heterogeneity are likely to have genotypic heterogeneity. There are so far only two studies to examine this at the DNA level; one study of two advanced-stage renal cell carcinomas that exhibited extreme genotypic heterogeneity 23 and one study of five early-stage (2/3) breast cancers that showed little to no genotypic heterogeneity 24 when sampled and studied at multiple sites.
Ploidy and Copy Number Alterations in DNA
Altered numbers of chromosomes (more or fewer than 2) have been widely observed in cancer cells, likely reflecting errors in chromosomal segregation that occur during rapid division and growth cycles. Observing ploidy alterations requires cytogenetic examination of the tumor cells in metaphase, which may or may not be part of the pathology-based diagnosis for the patient sample. Alternatively, ploidy alterations and large chromosomal arm or subarm amplifications and deletions (somatic copy number alterations or SCNAs) can be inferred from signal strength–based analysis of genotyping array data. 25,26 Ploidy increases and arm or subarm amplifications are important in DNA sequencing of the tumor because these regions will contribute more DNA to the library, and hence more reads will result than for the diploid (or haploid) regions of the genome. Thus increased coverage must be obtained for the tumor library to compensate for amplified regions or ploidy-altered chromosomes so the coverage of diploid genomic regions is sufficient for variant detection. Careful analysis of aligned reads in copy number–altered regions can provide exquisite resolution of the genes involved and of the relative timing of somatic mutation and copy number alteration when both occur in the same locus. 27
FFPE Preservation and Nucleic Acid Integrity
Most pathology assays used in cancer diagnosis and characterization require stability of proteins and cellular structure. Hence, fixation in formalin and embedding in paraffin have been the standard pathology preparation methods for more than 100 years. As this practice is unlikely to change in the near future, and because so many clinically valuable specimens already have been preserved by this method, the study of formalin-fixed, paraffin-embedded (FFPE)-preserved nucleic acid isolates by NGS methods is increasing. The chemical reaction between formaldehyde, proteins, and nucleic acids leads to crosslinking proteins and nucleic acids, and ultimately the DNA/RNA backbone breaks because of the presence of abasic lesions. 28 This is a random interaction, and DNA/RNA fragmentation increases with longer exposure of nucleic acids to formalin and over time of storage. Therefore, the older a tumor FFPE block, the more likely to be advanced the degradation of the nucleic acid components. Nonetheless, careful examination of the nucleic acid integrity will identify those samples suitable for library construction for DNA or RNA, based on the average size and distribution of degraded nucleic acid isolated from the sample. In DNA isolates, the average fragment size should be 300 bp or greater or a suitable NGS library is unlikely to result. In RNA, the 28S and 18S rRNA peaks should be visible by gel electrophoresis, with an RNA integrity number (RIN) of at least 5.
Applications of NGS to Study and Analyze Nucleic Acids
The genomic DNA isolated from cancer cell nuclei can be studied in a variety of ways, several of which are profiled here. Because cancer develops from alterations of the nuclear genome that are distinct from the germline genome, an inherent and powerful comparison can be obtained by studying the paired tumor and normal genomic DNA from individual cancer patients. In discovery efforts, large numbers of such cases can be studied to add information about the frequency of different types of somatic alterations and the genes whose protein products will be altered as a result. Higher level analyses of the pathways affected by somatic alterations in DNA can further inform the resulting tumor biology. Studies of RNA by NGS methods have deepened our understanding of the numerous types of RNAs, their membership, and how they are altered in the course of carcinogenesis, although not all alterations are comprehensible in the biological context. The latter reflects our ignorance of the many roles these molecules play in cellular biology, emphasizing the need for functional studies as a follow-on to NGS-mediated discovery efforts.
Whole-Genome Sequencing
The most comprehensive approach to identifying the somatic alterations present in cancer genomics is obtained by whole-genome sequencing (WGS) of the tumor and normal DNAs. In this approach (see Figure 23-2), the isolated high-molecular-weight genomic DNA from each tissue is fragmented by the application of high-frequency sound waves or other physical shearing methods and then enzymatically treated to blunt the fragment ends that result. Finally, short synthetic adapters are added to make a whole-genome library. After limited PCR amplification by primers that correspond to the forward and reverse adapters, a gel-based sizing allows specific size fractions to be isolated (two to four insert sizes are typical to enhance library diversity and genome representation). The more precise the size fraction, the more precisely structural variants can be identified by virtue of their relative position once mapped to the reference genome (see Figure 23-3). Libraries are then quantitated, diluted to the appropriate concentration, and amplified in situ to produce collections of fragments, each of which originated from a single library fragment. Thus the data generated from WGS are “digital” in nature and can be interpreted later in this context, to provide highly precise information about chromosomal amplification and deletion events genome-wide, and the relative frequency of mutations in the tumor cell genomes sampled by DNA isolation. 22 Read pair data are then generated from tumor and normal libraries to a minimum depth of 30-fold, allowing for a mapping rate of around 85% of read pairs; this equates roughly to 120 Gbp of data per genome. Following data generation, the signals obtained from the stepwise sequencing process are interpreted by instrument-specific software, culled for low-quality sequences, paired, and provided to the mapping algorithm for alignment to the Human Reference Genome as outlined earlier. Alignment is done for tumor-specific reads and for normal-specific reads separately. Variants are identified and then compared to one another. 29 There are many specialized algorithms that have been specifically developed to evaluate the somatic variants that are carried by the cancer cell genomes decoded by whole genome sequencing data. Depending upon the algorithm type, one can identify somatic single nucleotide variants (SNVs), focused insertion and deletion events of one to several nucleotides (in/dels), and larger, structural events such as translocations, inversions, deletions, and amplifications. Loss of heterozygosity (LOH) is a common somatic genome event, and there also are algorithms to identify stretches of LOH along chromosomes. Each algorithm has an associated false positive rate, so secondary validation of putative somatic variants is the best practice. The identification of structural variants is particularly prone to a high false positive rate due to the difficulty of identifying these regions, as illustrated in Figure 23-3. Here, the distance and orientation of read pair mapping to the Human Genome Reference for multiple unique read pairs is required to identify a structural event, as indicated in the figure. By using the read pairs that identify the event, and a short read assembly algorithm, one can reassemble the structural variant event to nucleotide resolution. Finally, one makes “sense” of the variants identified genome-wide by annotation, effectively overlaying our current understanding of genes, regulatory regions, and other identified features that help define the tumor-unique profile of genomic alterations.
As sequencing costs have dropped and instrument throughput has increased, the amount of read data and hence the coverage of the tumor genome has increased. This increase has occurred for several reasons: notably, the confidence of detecting somatic variations typically increases with increasing coverage. Furthermore, the heterogeneous nature of cancer cell genomes means that increased coverage provides enhanced characterization of the mutational spectrum within the cells. Tumor progression is at its essence an evolutionary process in which new mutations arise from the fundamental tumor genome (often referred to as the “founder clone”) and expand into new subpopulations of cells. 22,30 Thus, the higher the tumor genome coverage, the more likely it is that subpopulations can be identified.
Exome Sequencing
Although WGS data are straightforward to produce and provide comprehensive genome-wide information about somatic alterations, their production remains expensive, and they are difficult to accurately interpret. Much like the early PCR-directed methods used to characterize cancer somatic mutations, technology development efforts in NGS have resulted in an application typically referred to as “hybrid capture” to selectively isolate regions of the genome followed by NGS. 31–33 Sequence-based comparison of the isolated regions between tumor and normal generate specific information about somatic and germline SNVs and indels. Hybrid capture protocols combine the whole-genome library fragments from tumor and normal with a collection of specific probe sequences designed to capture, by hybridization, those fragments in the population that contain the same loci the probes represent. Because capture probes carry covalently attached biotin moieties, the probe:library fragment hybrids can be selectively removed from solution by association with streptavidin-coated magnetic particles and the application of a magnet. Unhybridized fragments are removed with the supernatant, and a secondary wash eliminates many (but not all) spurious hybridization events (typically referred to as “off-target effects”). The resulting captured fragments are eluted from the beads by denaturation, quantitated, and sequenced to about 100-fold average depth. In one commonly used version of hybrid capture, probes representing nearly all of the annotated exons in the human genome (the “exome”) permit selective capture of these exons so they can be sequenced, compared, and annotated with respect to the somatic alterations identified. Exome capture reagents are available from commercial manufacturers, and the associated methods can be automated readily to provide a very high throughput of exome capture reactions, suitable for large-scale cancer discovery. As an alternative, custom capture probe sets can be designed and manufactured by one of several commercial suppliers to selectively isolate genes/loci of interest and characterize their mutational status in a large number of cancer cases or as a diagnostic reagent to assess specific mutational hotspots. This approach also can be used to select loci from a whole-genome library that carry putative variants, as a means of validation that mutations indeed exist. 22 Hybrid capture becomes of limited utility when the target loci (also referred to as “regions of interest” or ROIs) to be isolated fall below a combined length of approximately 500 kbp, mainly because the amount of off-target hybridization increases as the target space decreases. Because off-target captured fragments contribute to the overall fragment pool that is recovered for sequencing, the amount of sequence data actually mapping to the loci of interest decreases steadily to the point that data generation becomes too expensive to obtain the necessary coverage in desired targets. Below an ROI of about 500 kbp, either PCR and amplicon pooling or multiplex PCR are typically used.
DNA Methylation
One predominant mechanism of transcriptional control in cells is the covalent modification of the cytosine bases in DNA by methyl groups and their derivatives. Understanding the ways that methylation status changes in tumor cells can provide insights into changes in gene expression patterns, as well as new prognostic markers if sufficient clinical data and samples exist. This type of analysis requires comparator normal methylation data, ideally obtained from adjacent nonmalignant tissue genomic DNA isolates. Correlative analyses then can link DNA methylation changes to gene expression changes, providing insights into tumor biology that cannot be obtained by directly sequencing genomic DNA. Several approaches to identifying methylated cytosines in genomic DNA use chemical modification, antibody-based recognition of methylC, or comparative restriction enzyme digestion patterns from exposure to a methylation-sensitive versus non–methylation-sensitive isoschizomer. However, the most widely used approach is bisulfite modification. 34,35 In bisulfite modification, native genomic DNA is treated with sodium bisulfite to convert unmethylated cytosines to uracils (see Figure 23-4
Buy Membership for Hematology, Oncology and Palliative Medicine Category to continue reading. Learn more here