5 Prediction of Radiation Response
The prescription for clinical radiotherapy is usually based on such factors as tumor site, stage, and grade. All patients with tumors falling in the same category with regard to these clinical parameters receive the same radiation schedule, conforming to the institute’s current policy. An increasing body of evidence now shows that even for similar histologic type and extent of tumor, wide variations exist in the response to irradiation. For example, the fractionation scheme (e.g., conventional, accelerated, hyper- or hypo-fractionation) has been shown to influence outcome in clinical trials.1–5 Total dose also influences outcome. It is therefore likely that a single radiotherapy prescription is not optimum for all patients, even those within the same clinical category. Some patients, but not all, may benefit from accelerated fractionation (depending on proliferation rate of the tumor), some but not all may benefit from hyperfractionation (depending on the shape of the survival curve of the tumor cells), some may require higher doses (radioresistant tumors), others may be overtreated with conventional doses (radiosensitive tumors), while for others, conventional radiotherapy of 1.8 to 2 Gy per day for 6 to 7 weeks will be the best choice.
Genetic Assays: Data-Driven Approach
Tumors
The clinical value of microarrays was shown by studies in breast cancer,6,7 lymphoma,8 lung adenocarcinoma,9 glioma,10 and others that showed tumors could be subdivided into groups based on their gene expression profiles—and that these subdivisions have clinical relevance, since the different groups have different prognoses (Fig. 5-1). In addition, the prognostic potential of microarrays was shown by van’t Veer and colleagues,11 who defined a 70-gene signature that predicted the chance of distant metastases in young women with breast cancer. Several other signatures with the same utility have been found in subsequent studies, often containing different genes.12,13 Of note is that these different signatures usually select the same patients as being at high or low risk, suggesting that many different gene sets may have similar prognostic potential for the same clinical situation and may represent different genes on common deregulated pathways.14–16 In addition to distant metastases, it also appears to be possible to predict the chance of developing regional lymph node metastases in sites such as head and neck, breast, and others.17–19
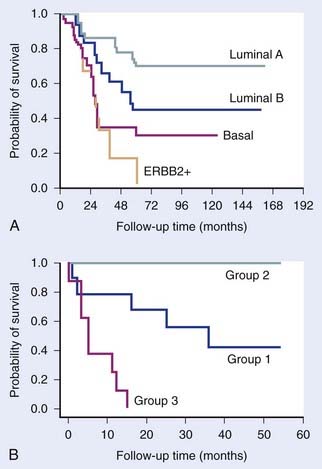
FIGURE 5-1 • Examples of the clinical value of gene expression profiling by microarrays. (A) Study by Sorlie and colleagues7 on breast tumors showing that clusters defined by differences in expression patterns have significantly different outcomes. (B) Study by Garber et al.9 showing a similar result for lung tumors.
(Redrawn from Sorlie T, Tibshirani R, Parker J, et al: Repeated observation of breast tumor subtypes in independent gene expression data sets, Proc Natl Acad Sci USA 100:8418–8423, 2003; Garber ME, Troyanskaya OG, Schluens K, et al: Diversity of gene expression in adenocarcinoma of the lung, Proc Natl Acad Sci USA 98:13784–13789, 2001.)
It is useful at this stage to distinguish between the terms prognostic and predictive. Several gene signatures have been described that appear to discriminate between good and poor outcome, but in a variety of disease sites and in patients given a variety of different treatments. Such signatures can be described as prognostic and probably reflect degree of malignancy. Examples are the “wound” signature,20 hypoxic signatures,21 genetic instability signatures,22 and stem cell signatures.23 By contrast, a signature that discriminates between good and bad responders to a specific treatment can be described as predictive. Such signatures can help in treatment selection, whereas prognostic signatures are inherently less useful. A predictive signature can also, in principle, help unravel causes of resistance, leading to potential new intervention strategies.
To date, very few predictive signatures have been described. Chung and colleagues24 defined a 75-gene high risk signature for patients with head and neck cancer treated with primary surgery followed by radiation and/or chemotherapy. Pramana and colleagues25 subsequently showed that this signature also predicted locoregional control in an independent series of head and neck cancer patients treated with radiotherapy and concomitant cisplatin (Fig. 5-2). However, the specificity of this signature for radiotherapy, with or without chemotherapy, remains to be determined. Nuyten and colleagues26 described a signature for predicting local recurrence after breast conserving therapy (local excision plus radiotherapy). Signatures have also been described that predict tamoxifen resistance in breast cancer.27,28 Validated signatures predicting response to radiotherapy alone in cancer patients have not yet been reported.
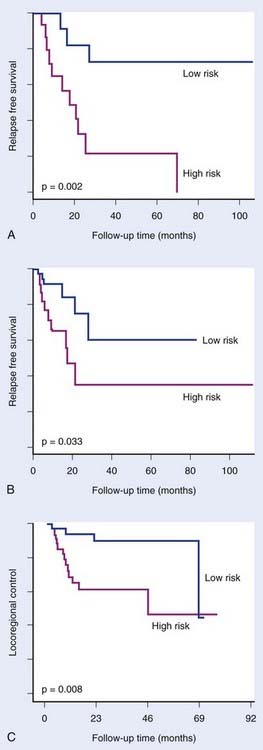
FIGURE 5-2 • Chung et al.24 defined an expression signature comprising 75 genes associated with high risk of relapse in head and neck cancer treated with primary surgery with or without subsequent treatment with radiation and/or chemotherapy. The signature significantly distinguished good and poor prognosis groups in an initial group of 28 patients (A) and in a subsequent group of 60 patients (B). Pramana et al.25 found this signature to predict locoregional control after concurrent radiotherapy and cisplatin in an independent group of 70 head and neck tumors (C).
(Redrawn from Chung CH, Parker JS, Ely K, et al: Gene expression profiles identify epithelial-to-mesenchymal transition and activation of nuclear factor–kappaB signaling as characteristics of a high-risk head and neck squamous cell carcinoma, Cancer Res 66:8210–8218, 2006; Pramana J, Van den Brekel MW, van Velthuysen ML, et al: Gene expression profiling to predict outcome after chemoradiation in head and neck cancer, Int J Radiat Oncol Biol Phys 69:1544–1552, 2007.)
MicroRNAs (miRNA) also have been shown to have predictive or prognostic potential. These are small 18- to 22-nucleotide single-stranded RNAs. They are transcribed from genomic DNA like messenger RNA (mRNA), but do not code for proteins. Instead, they bind to partially complementary sequences on target messenger RNAs, thereby inhibiting translation and sometimes causing mRNA degradation. It is estimated that the expression of up to half of all genes are regulated by miRNAs. It is therefore not surprising that they have been shown to be involved in carcinogenesis and treatment response. Reports are now appearing of the predictive potential of miRNAs,29,30 which may ultimately prove to be at least as powerful as mRNA profiling for prediction (Fig. 5-3). To date, no study testing miRNA as a predictor of outcome after radiotherapy has been reported, although specific miRNAs have been shown to be induced by hypoxia and to correlate with outcome in breast cancer patients treated by surgery and adjuvant therapies.29
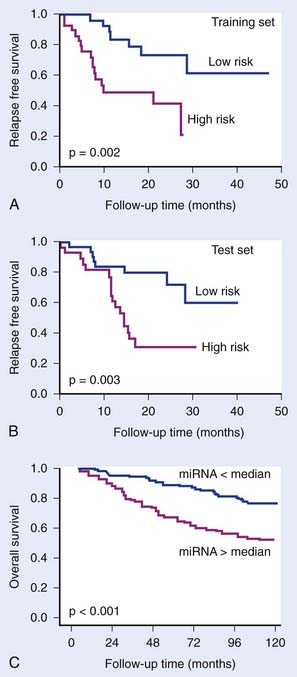
FIGURE 5-3 • The prognostic potential of microRNAs (miRNA). Yu et al.30 showed that a 5-gene miRNA signature distinguished good and poor outcome groups in non–small cell lung cancer in a training group of 56 patients (A) and in a test group of 56 patients (B). Camps et al.29 showed that a single miRNA, hsa-miR-210, which is induced under hypoxia, could distinguish good and poor outcome in 219 breast cancer patients. Camps et al. showed that a single miRNA, hsa-miR-210, which is induced under hypoxia, could distinguish good and poor outcome in 219 breast cancer patients (C).
(Redrawn from Yu SL, Chen HY, Chang GC, et al: MicroRNA signature predicts survival and relapse in lung cancer, Cancer Cell13:48-57, 2008, and Camps C, Buffa FM, Colella S, et al: hsa-miR-210 Is induced by hypoxia and is an independent prognostic factor in breast cancer, Clin Cancer Res 14:1340-1348, 2008.)
Methylation of DNA in the so-called CpG islands in gene promoter regions can silence expression of those genes. Conversely, demethylation can activate transcription. Deregulation of methylation can thus lead to silencing of tumor suppressors or activation of oncogenes. Deregulation of genes involved in drug metabolism, the DNA damage response, and others can affect response to chemotherapy and radiotherapy. The most consistent result concerning prediction is in glioblastoma, where methylation of the O-6-methylguanine-DNA methyltransferase (MGMT) promoter is associated with improved survival in patients treated with radiotherapy plus alkylating agents, particularly temozolamide.31,32 The MGMT enzyme can reverse drug-induced DNA alkylation, thus reversing its cytotoxic effects. Gene methylation will reduce expression of this DNA repair gene, leading to increased tumor responses. Studies in other tumors have also shown correlations between methylation status of specific genes and outcome in colorectal cancer,33 neuroblastoma,34 and others. No clinical studies on radiotherapy alone have reported on methylation status. Several methods have been developed to measure DNA methylation, some of them approaching a genome-wide scale, and are now being increasingly applied to test their predictive potential.
Comparative genomic hybridization (CGH) measures copy number variations (CNV; amplifications and deletions) in regions of genomic DNA, currently at a 5 to 10 kilobase resolution. This method allows characterization of recurrent chromosome changes in tumors, which can pinpoint relevant known or novel oncogenes and suppressor genes. In addition, CNVs can be correlated with outcome to define CNV predictors, analogous to gene expression predictors. The resolution of current arrays is such that candidate genes in affected loci can be rapidly traced and tested further for their involvement if desired or necessary. Reports have appeared showing the prognostic potential of CNVs in several cancer types, including breast,35 gliomas,36 colorectal cancers,37 Wilms’ tumor38 and others (Fig. 5-4). Few studies have been done on radiotherapy patients, although van den Broek and colleagues39 reported finding specific gains and losses in head and neck cancers that correlated with outcome after treatment with combined chemoradiotherapy. It is likely that CGH will complement gene expression and other genome wide assays in defining robust predictors.
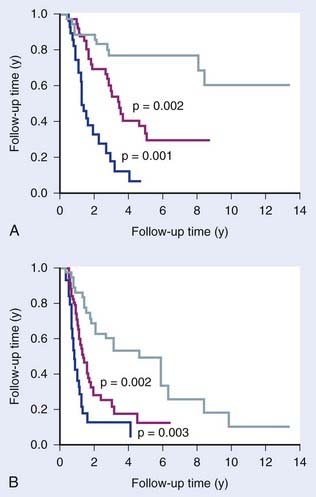
FIGURE 5-4 • The prognostic potential of comparative genomic hybridization (CGH). Idbaih et al.36 defined three groups of gliomas based on their patterns of genomic amplifications and deletions that showed prognostic significance for overall survival (A) and progression free survival (B).
(Redrawn from Chin SF, Teschendorff AE, Marioni JC, et al: High-resolution aCGH and expression profiling identifies a novel genomic subtype of ER negative breast cancer, Genome Biol 8:R215, 2007.)
Normal Tissues
Predicting the chance of adverse normal tissue reactions after radiation would also be a valuable aid, allowing dose adjustments and/or protective measures (radioprotective or ameliorating drugs) to be prescribed to patients at risk. It further opens the possibility of increasing tumor radiation doses for the remainder (the majority) of patients, leading to potential increases in cure rates for the patient population as a whole. Several factors are known to affect the response of normal tissues to irradiation. The extent of cell kill of parenchymal cells in the organ at risk is clearly important but is not the only factor. Cytokines are known to play an important role,40,41 TGF-β being a key cytokine in fibrosis development, influencing fibroblast proliferation and differentiation.
Attempts have been made to predict the chance of adverse reactions from the transcriptional response of patient’s lymphocytes irradiated ex vivo.42 The underlying assumption is that the genetic profile of the patient will affect radiation response in all tissues and can therefore be monitored in easily accessible peripheral blood lymphocytes. The further assumption is that sensitivity is best monitored not necessarily by the basal level of gene expression but by transcriptional response to radiation. In the Svensson study,42 an expression signature in ex vivo irradiated lymphocytes was identified that discriminated prostate cancer patients with severe late complications following radiotherapy (over-responders) from patients without such complications (nonresponders). Lymphocytes have also been used with some success for predicting radiation induced complications using colony survival43 and apoptosis assays,44 supporting the use of these cells for predictive purposes.
Gene polymorphisms have also been studied in relation to adverse radiotherapy induced reactions. Single-nucleotide polymorphisms (SNP) in several genes have been found to correlate with radiation induced fibrosis,45 particularly in the TGF-β gene. Lymphocytes represent ideal test material, since they are easy to obtain and SNPs will be the same in all cells (in contrast to gene expression). SNP predictors of adverse reactions are not yet robust, because the studies carried out to date have been small and only a few candidate genes have been investigated. The method appears promising, but evaluation will have to await the results of several ongoing larger studies looking at genome-wide SNPs.
Genetic Assays: Hypothesis-Driven Approach
Radiosensitivity
Many radiosensitivity genes have been discovered using knockout, knock-down or chemical inhibitor strategies. Many of these genes are involved in DNA repair. However, in recent years it has become clear that the DNA damage response is highly complex, so that predicting the extent of radiation induced killing for any random cell line or tumor has proven to be difficult. One approach has therefore been to use a genome-wide strategy, not dependent on known data or pathways, in which genetic signatures are sought that correlate with intrinsic radiosensitivity in a series of cell lines or tumors. Torres-Roca et al.46 described such an approach on selected cell lines from the National Cancer Institute (NCI) panel and found a small set of genes correlating with radiosensitivity. More recently, Amundson and colleagues47 extended the studies to the complete NCI panel of cell lines and found a larger set of genes correlating with intrinsic radiosensitivity, with some overlap with the Torres-Roca set. Khodarev and colleagues48 used a different approach combining animal tumor and cell line studies, creating a radioresistant and radiosensitive pair of cell lines, to define a radiosensitivity signature. Little has so far been reported on testing radiosensitivity signatures. Pramana et al.25 tested one of these signatures (Torres-Roca) on a group of head and neck cancer patients treated with radiation plus cisplatin, but no significant correlation was found. Recently, this signature has been independently refined, and the updated signature showed a strong trend (P = .06) that patients with tumors predicted to be radiosensitive had a better outcome after chemoradiotherapy (Torres-Roca J, et al., presented at ASTRO, Boston, 2008).
Hypoxia
It has been known for more than half a century that hypoxic cells are up to three times more radioresistant than normoxic cells. In addition, hypoxic cells in tumors are often more slowly proliferating and harder to reach with drugs, because they often are at a distance from blood vessels, making them more chemoresistant. Studies using glass electrodes to measure oxygen tension in human tumors have shown, firstly, how ubiquitous hypoxia is, and secondly, that it is a negative prognostic factor for all the three current major treatment modalities of surgery, radiotherapy, and chemotherapy.49–54
Cells react to hypoxia by reducing the expression of many genes. However, in contrast, there are a smaller number of genes that are up-regulated. Many of these are dependent on HIF-1α (hypoxia inducible factor), a protein that is stabilized under hypoxic conditions, leading to accumulation in the cell. HIF-1α, a transcription factor, then switches on transcription of a plethora of other genes that have an HRE (HIF responsive element, a specific short DNA sequence) in their promoter region. This allows the cell to adapt to hypoxic stress by, among others, increasing glucose uptake and stimulating angiogenesis. Such up-regulated genes represent a hypoxic signature. Several such signatures have been defined by growing cells under hypoxic conditions and subsequently measuring their expression profiles. Chi et al.21 showed that such in vitro–defined signatures have prognostic significance and are more predictive of outcome (overall and relapse-free survival) in breast and ovarian cancer than present clinical parameters. As expected, patients with tumors showing high expression of hypoxia genes, indicating high tumor hypoxia, did worse.
The cell’s response to hypoxia also depends on the degree and duration of hypoxia. More severe hypoxia and longer times under hypoxia are reflected by altered gene expression patterns. Signatures have therefore been described for both acute and chronic hypoxia, and for different degrees of hypoxia.21,55 These are potentially important distinctions, since there is accumulating evidence that acute hypoxia is more dangerous than chronic hypoxia (equally radioresistant, more viable, more DNA repair–proficient).56,57 Indeed, Seigneuric and colleagues55 showed that in vitro signatures derived from short exposures to hypoxia (acute) predicted outcome in breast cancers whereas those derived from longer exposures (chronic) did not (Fig. 5-5).
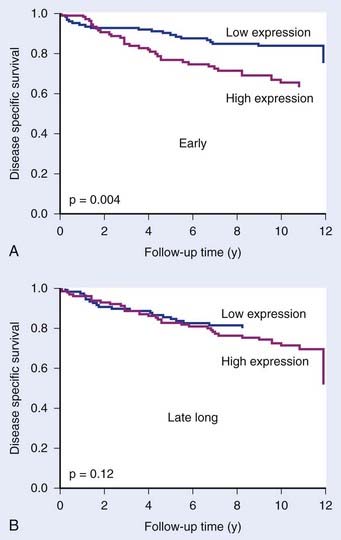
FIGURE 5-5 • The prognostic potential of gene expression signatures for hypoxia. Seigneuric et al.55 analyzed cell line data to define signatures for genes up-regulated at early times during hypoxic incubation (“early”; acute hypoxia) or after long times (“late long”) chronic hypoxia. Acute signatures (A) had greater potential to distinguish good and poor prognosis in breast cancer patients than chronic signatures (B).
(From Seigneuric R, Starmans MH, Fung G, et al: Impact of supervised gene signatures of early hypoxia on patient survival, Radiother Oncol 83:374-382, 2007.)
An alternative method of deriving hypoxic signatures was described by Winter et al.58 They first chose 10 genes known to be HIF-1α (and thus hypoxia)–dependent. They then looked for other genes whose expression correlated with expression of these 10 “seed” genes across a series of human head and neck carcinomas. In this way they defined a hypoxic metagene of 99 genes. This metagene had prognostic value in both head and neck and breast cancer, supporting the notion that hypoxia is an important negative prognostic factor and that gene signatures can be used to monitor it.
Repopulation
During the course of fractionated radiotherapy, tumor cells that have survived (remained clonogenic) up to that point can begin to divide and will increase the number of cells to be killed with the remaining dose fractions. Such tumor cell repopulation is a particular risk during weekends and other gaps in therapy. Cell kill by radiotherapy is thus counteracted by cell production (repopulation) in the treatment gaps. The greater the capacity of a tumor for repopulation, the greater the effective resistance of that tumor will be. Further, the more gaps in treatment there are, the greater the opportunity will be for repopulation. Evidence for this is strongest in head and neck cancer where a number of studies on the influence of treatment time, including split dose and accelerated fractionation, have consistently shown either worse local control if the treatment time is longer, or that higher radiation doses are needed for a given level of local control.1–4 This is most likely to be due to repopulation, which can therefore limit cure in some cancers. The influence of repopulation will vary between cancers of the same type, and between different types of cancer. Cancers that are in general more slowly growing, (e.g., breast and prostate), may be at less risk from repopulation, although some tumors within these types may be capable of rapid repopulation. It is therefore important to be able to predict repopulation in individual tumors.