Chapter 40 Clinical Neurogenetics
Genetics in Clinical Neurology
Gene Expression, Diversity, and Regulation
Types of Genetic Variation and Mutations
Chromosomal Analysis and Abnormalities
Disorders of Mendelian Inheritance
Mendelian Disease Gene Identification by Linkage Analysis and Chromosome Mapping
Non-Mendelian Patterns of Inheritance
Common Neurological Disorders and Complex Disease Genetics
Whole Genome/Exome Sequencing in Disease Gene Discovery
Future Role of Systems Biology in Neurogenetic Disease
Environmental Contributions to Neurogenetic Disease
Genetics and the Paradox of Disease Definition
Clinical Approach to the Patient with Suspected Neurogenetic Disease
Genetics in Clinical Neurology
Since the discovery of the structure of deoxyribonucleic acid (DNA) and the elucidation of the genetic mechanisms of heredity, clinical neurology has benefited from advances in genetics and neuroscience. This clinically relevant basic research has permitted dissection of the cellular machinery supporting the function of the brain and its connections while establishing causal relationships between such dysfunction, human genetic variation, and various neurological diseases. In the modern practice of neurology, the use of genetics has become widespread, and neurologists are confronted daily with data from an ever-increasing catalogue of genetic studies relating to conditions such as developmental disorders, dementia, ataxia, neuropathy, and epilepsy, to name but a few. The use of genetic information in the clinical evaluation of neurological disease has expanded dramatically over the past decade. More efficient techniques for discovering disease genes has led to a greater availability of genetic testing in the clinic. Approximately one-third of pediatric neurology hospital admissions are related to a genetic diagnosis, and there are many dozens of genetic tests available to the practicing neurologist, including several related to common diseases. This number will surely continue to increase rapidly (Fig. 40.1).
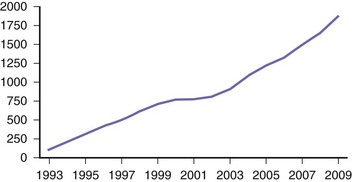
(Data from GeneTests: Medical Genetics Information Resource. Available at http://www.ncbi.nlm.nih.gov/sites/GeneTests/.)
As neuroscience and genetic research have progressed, we have been led to a deeper understanding of the sources and nature of human genetic variation and its relationship to clinical phenotypes. In the past there has been a tendency to consider genetic traits as either present or absent, and correspondingly, patients were either healthy or diseased; this is the traditional view of Mendelian, or single gene, conditions. Although certain relatively rare neurological diseases—Friedreich ataxia or Huntington disease (HD), for example—can be traced to a single causal gene, the common forms of other diseases such as Alzheimer dementia, stroke, epilepsy, or autism usually arise from an interplay of multiple genes, each of which increases disease susceptibility and likely interacts with environmental factors. Subsequently, the realm of the “sporadic” and the “idiopathic” has been challenged by the identification of genetic susceptibility factors, which has sparked a flurry of investigation into a variety of genes and genetic markers that confer a risk of illness yet are not wholly causative. Disease status may lie on the end of a continuum of individual variation and thus can be considered a quantitative rather than purely qualitative trait (Plomin et al., 2009). So, rather than using what might be considered an arbitrary cutoff point, such as a specific number of senile plaques or neuritic tangles that define affected or unaffected patients, one might instead think in terms of a continuum of pathology that relates to different levels of burden or susceptibility.
Gene Expression, Diversity, and Regulation
The basic principles of molecular genetics are outlined in Fig. 40.2 and Table 40.1, and more detailed descriptions can be found elsewhere (Alberts et al., 2008; Griffiths et al., 2002; Lodish et al., 2008; Strachan and Read, 2003). To briefly summarize, deoxyribonucleic acid (DNA), found in the nucleus of all cells, comprises the raw material from which heritable information is transferred among individuals, with the simplest heritable unit being the gene. DNA is composed of a series of individual nucleotides, all of which contain an identical pentose (2′-deoxyribose)-phosphate backbone but differ at an attached base that can be either adenine (A), guanine (G), thymine (T), or cytosine (C). A and G are purine bases and pair with the pyrimidine bases T and C, respectively, to form a double-stranded helical structure which allows for semiconservative bidirectional replication, the means by which DNA is copied in a precise and efficient manner. In total, there are approximately 3.2 billion base pairs in human DNA. By convention, a DNA sequence is described by listing the bases as they are expressed from the 5′ to 3′ direction along the pentose backbone (e.g., 5′-ATGCAT-3′…etc.), as this is the order in which it is typically used by the cellular machinery, also called the sense strand (compare to RNA, later). The opposite paired, or antisense, strand is arranged antiparallel (3′ to 5′) and can also be referred to when discussing sequence; however, by convention this is generally not done unless that strand is also transcribed into RNA.
Allele | Alternate forms of a locus (gene) |
Anticipation | Earlier onset and/or worsening severity of disease in successive generations |
Antisense | Nucleic acid sequence complementary to mRNA |
Chromosome | Organizational unit of the genome consisting of a linear arrangement of genes |
Cis-acting | A regulatory nucleotide sequence present on the molecule being regulated |
Codon | A three-nucleotide sequence representing a single amino acid |
Complex disease | Disease exhibiting non-Mendelian inheritance involving the interaction of multiple genes and the environment |
De novo | A mutation newly arising in an individual and not present in either parent |
Diploid | A genome having paired genetic information, half-normal number is haploid |
DNA | Deoxyribonucleic acid; used for storage, replication, and inheritance of genetic information |
Dominant | Allele that determines phenotype when a single copy is present in an individual |
Endophenotype | Subset of phenotypic characteristics used to group patients manifesting a given trait |
Exome | Portion of the genome representing only the coding regions of genes |
Exon | Segment of DNA that is expressed in at least one mature mRNA |
Expressivity | The range of phenotypes observed with a specific disease-associated genotype |
Frameshift | DNA mutation that adds or removes nucleotides, affecting which are grouped as codons |
Gene | Contiguous DNA sequence that codes for a given messenger RNA and its splice variants |
Genome | A complete set of DNA from a given individual |
Genotype | The DNA sequence of a gene |
Haplotype | A group of alleles on the same chromosome close enough to be inherited together |
Hemizygous | Genes having only a single allele in an individual, such as the X chromosome in males |
Heteroplasmy | A mixture of multiple mitochondrial genomes in a given individual |
Heterozygous | Genes having two distinct alleles in an individual at a given locus |
Homozygous | Genes having two identical alleles in an individual at a given locus |
Intron | Segment of DNA between exons that is transcribed into RNA but removed by splicing |
Kilobase | 1000 bases or base-pairs |
Linkage disequilibrium | The co-occurrence of two alleles more frequently than expected by random chance, suggesting they are in close proximity to one another |
Locus | Location of a DNA sequence (or a gene) on a chromosome or within the genome |
Lyonization | The process of random inactivation of one of the pair of X chromosomes in females |
Marker | Sequence of DNA used to identify a gene or a locus |
Megabase | 1,000,000 bases or base-pairs |
Meiosis | Process of cellular division that produces gametes containing a haploid amount of DNA |
Mendelian | Obeying standard single-gene patterns of inheritance (e.g., recessive or dominant) |
Microarray | A glass or plastic support (e.g., slide or chip) to which large numbers of DNA molecules can be attached for use in high-throughput genetic analysis |
Missense | DNA mutation that changes a given codon to represent a different amino acid |
Mitosis | Process of cellular division during which DNA is replicated |
Nonsense | DNA mutation that changes a given codon into a translation termination signal |
Penetrance | The likelihood of a disease-associated genotype to express a specific disease phenotype |
Phenotype | The clinical manifestations of a given genotype |
Polymorphism | Sequence variation among individuals, typically not considered to be pathogenic |
Probe | DNA sequence used for identifying a specific gene or allele |
Promoter | DNA sequences that regulate transcription of a given gene |
Protein | Functional cellular macromolecules encoded by a gene |
Recessive | Allele that determines phenotype only when two copies are present in an individual |
Relative risk | The ratio of the chance of disease in individuals with a specific genetic susceptibility factor over the chance of disease in those without it |
Resequencing | A method of identifying clinically relevant genetic variation in a candidate gene of interest by comparing the sequence in individuals with disease to a reference sequence |
RNA | Ribonucleic acid; expressed form of a gene, called messenger or mRNA if protein coding |
Sense | Nucleic acid sequence corresponding to mRNA |
Silent | DNA mutation that changes a given codon but does not alter the corresponding amino acid |
SNP | Single nucleotide polymorphism |
Splicing | RNA processing mechanism where introns are removed and exons joined to create mRNA; in alternative splicing, exons are utilized in a regulated manner within a cell or tissue |
Trans-acting | A regulatory protein that acts on a molecule other than that which expressed it |
Transcription | Cellular process where DNA sequence is used as template for RNA synthesis |
Transcriptome | The complete set of RNA transcripts produced by a cell, tissue, or individual |
Translation | Cellular process where mRNA sequence is converted to protein |
The expression of a gene is tightly and coordinately regulated (see Fig. 40.2), an important consideration for understanding the molecular mechanisms of disease. The typical gene contains one or more promoters, DNA sequences that allow for the binding of a cellular protein complex that includes RNA polymerase and other factors that faithfully copy the DNA in the 5′ to 3′ direction in a process known as transcription. The resulting single-stranded molecule contains a ribose sugar unit in its backbone and, thus the resulting molecule is termed ribonucleic acid, or RNA. RNA also differs from the template DNA by the incorporation of uracil (U) in place of thymine (T), as it also pairs efficiently with adenine, and thymine serves a secondary role in DNA repair that is not necessary in RNA. The sequence of the RNA matches the sense DNA strand and is therefore complementary to (and hence derived from) the antisense strand.
Transcribed coding RNA must be processed to become protein-encoding messenger RNA (mRNA), a term used to differentiate these RNAs from all other types of RNA in the cell. To become mature, RNA is stabilized by modification at the ends with a 7-methylguanosine 5′ cap and a long poly-A 3′ tail. A further critical stage in the maturation of the RNA molecule involves a rearrangement process termed RNA splicing (Fig. 40.3). This is necessary because the expressed coding sequences in DNA, called exons, of virtually every gene are discontinuous and interspersed with long stretches of generally non-conserved intervening sequences referred to as introns. This, along with other mechanisms, likely plays an evolutionary role in the development of new genes by allowing for the shuffling of functional sequences (Babushok et al., 2007). Nascent RNA molecules are recognized by the spliceosome, a protein complex that removes the introns and rejoins the exons. Not every exon is utilized at all times in every RNA derived from a single gene. Exons may be skipped or included in a regulated manner through alternative splicing, which occurs in nearly 95% of all genes to create different isoforms of that mRNA. The dynamic nature of this observation is critical to a complete understanding of cellular gene expression. DNA is essentially a storage molecule, and with few exceptions in the absence of mutagens, its sequence remains static and, aside from a few epigenetic events, is therefore limited to a genetic regulatory role as a transcriptional rheostat. Current estimates place the number of individual human genes at just over 22,000 (Pertea and Salzberg, 2010), so it is difficult to reconcile biological and clinical diversity with simple variations in expression. Alternative splicing provides a means of dramatically elevating this diversity by enabling a single gene to encode multiple proteins with a wide array of functions. Supporting this, recent analysis of RNA complexity in human tissues suggest that there are at least seven alternative splicing events per multi-exon gene, generating over 100,000 alternative splicing events (Pan et al., 2008). Because alternative splicing and other forms of RNA processing can be subject to complex layers of temporal and spatial regulation, particularly in the human brain (Licatalosi and Darnell, 2010; Ward and Cooper, 2010), it is a robust source for both biological diversity and disease-causing mutations (see Polymorphisms and Point Mutations).
DNA to RNA to Protein
The central dogma of genetics has been that DNA is transcribed into RNA that is than translated into protein—the “business” end of the process. So, following its transcription from DNA in the nucleus, mRNA is transported out of the nucleus to the cytoplasm, and possibly to a specific subcellular location depending on the mRNA, where it can be deciphered by the cell. This takes place via interaction with a complex known as the ribosome, which binds the mRNA and converts its genetic information into protein via the process of translation. The ribosome initiates translation at a pre-encoded start site and converts the mRNA sequence into protein until a designated termination site is reached. Sequence information is read in three-nucleotide groups called codons, each of which specifies an individual amino acid. With the four distinct bases, there are mathematically 64 possible codons, but these have an element of redundancy and code for only 20 different amino acids and 3 termination signals (UAG, UGA, and UAA), also called stop codons. The start codon is ATG and codes for methionine. These amino acids are joined by the ribosome to synthesize a protein. This protein, which may undergo further modification, will ultimately carry out a programmed biological function in the cell. Regulation of this process is highly coordinated and important in learning, for example, where activity-dependent translation at the synapse underlies some aspects of synaptic plasticity, which may go awry in certain disorders such as fragile X syndrome and autism (Morrow et al., 2008).
Over the last decade, the discovery of several classes of functional non–protein coding RNAs has added additional complexity to our understanding of how the genetic code is manifest at the level of cellular function. Of these, microRNAs (miRNAs) are increasingly being recognized as vital players in gene regulation and neurological disease (Weinberg and Wood, 2009). Nascent miRNA molecules are processed to form short (approximately 22-nucleotide) RNA duplexes that target endogenous cellular machinery to specific coding RNAs and induce posttranscriptional gene silencing through a diverse repertoire including RNA cleavage, translational blocking, transport to inactive cell sites, or promotion of RNA decay (Filipowicz et al., 2008; Weinberg and Wood, 2009). Depending on the cell and the context, miRNA activity can result in specific gene inactivation, functional repression, or more subtle regulatory effects and may involve multiple RNAs in a given biological pathway (Flynt and Lai, 2008). Estimates suggest that miRNAs may regulate 30% of protein-coding genes, implicating these molecules as important targets for future research into the biology of neurological disease (Filipowicz et al., 2008; Weinberg and Wood, 2009).
It is important to emphasize that most genes are not simply “on” or “off.” In reality, cells maintain strict regulatory control over their genes. Some genes, such as those required for cell structure or maintenance, must be expressed constitutively, but genes with specific precise functions may only be needed in certain cells at certain times under certain conditions. Potential levels of regulation are depicted in Fig. 40.2 and include virtually every stage of gene expression. Initially, genes can be regulated at the level of transcription, ranging from the regulated binding of histone proteins, which leads to chromosome condensation, inactivating genes, to the coordinated activity of protein factors that activate or repress gene transcription in response to cell state, environmental conditions, or other factors. Once expressed, the RNA is subject to processing regulation, particularly through alternative splicing as already discussed. Transport of the mRNA and its translation provide additional steps for cellular regulation. Lastly, the final protein can be subject to control via posttranslational modifications or interactions with other proteins. To operate, all these levels of regulation require trans-acting factors, such as proteins, which stimulate or repress a particular step, as well as cis-acting elements, sequences recognized and bound by the regulatory factors.
These detailed levels of regulation provide a dynamic and expansive capability to precisely control cellular function, essential for growth, development, and survival in an unpredictable environment. However, this also provides many potential points at which disease can arise from disrupted regulation. Consequently, a defective gene could cause disease directly through its own action or indirectly by disrupting regulation of other cellular pathways. For example, the forkhead box P2 (FOXP2) transcription factor regulates the expression of genes thought to be important for the development of spoken language (Konopka et al., 2009). Mutations in this gene cause an autosomal dominant disorder characterized by impairment of speech articulation and language processing (Lai et al., 2001). However, other mutations in this gene are responsible for approximately 1% to 2% of sporadic developmental verbal dyspraxia (MacDermot et al., 2005), likely via downstream effects. Mutation of the methyl-CpG-binding protein 2 (MECP2), which regulates chromatin structure, causes the neurodevelopmental disorder, Rett syndrome, but other mutations in this gene can cause intellectual disability or autism (Gonzales and LaSalle, 2010). Similarly, the FOX1 protein (also called ataxin 2 binding protein 1, or A2BP1), a neuron-specific RNA splicing factor (Underwood et al., 2005) predicted to regulate a large network of genes important to neurodevelopment (Yeo et al., 2009; Zhang et al., 2008), causes autistic spectrum disorder when disrupted (Martin et al., 2007) but has also been implicated as a susceptibility gene associated with both primary biliary cirrhosis (Joshita et al., 2010) and hand osteoarthritis (Zhai et al., 2009), presumably due to downstream effects or specific effects in non-neural tissues. This concept of genes acting on other genes will be further explored later (see Common Neurological Disorders and Complex Disease Genetics).
In addition to the complexity of regulatory mutations that affect gene expression by altering RNA or protein levels or by disrupting RNA splicing, there are certain mutations that do not cause protein dysfunction, but instead have effects restricted to the RNA itself. For example, RNA inclusions are found in several forms of triplet repeat disorders (see Repeat Expansion Disorders) including myotonic dystrophy type 1 and the fragile X–associated tremor/ataxia syndrome (FXTAS) (Garcia-Arocena and Hagerman, 2010; Orr and Zoghbi, 2007). The latter is particularly interesting from a genetic standpoint, because a disorder of late-onset progressive ataxia, tremor, and cognitive impairment occurs in carriers of FMR1 alleles of intermediate sizes, which are not full fragile X–causing mutations (Garcia-Arocena and Hagerman, 2010). FXTAS is a dominant gain-of-function disease that occurs via an entirely different mechanism than the recessive loss-of-function disease, fragile X syndrome (Garcia-Arocena and Hagerman, 2010; Penagarikano et al., 2007). Primary disorders of RNA still represent relatively uncharted territory, and it is likely that more RNA-specific diseases will be identified. This is particularly exciting for many reasons, not the least of which is that certain classes of these disorders may be amendable to therapy (Nakamori and Thornton, 2010; Wheeler et al., 2009).
Types of Genetic Variation and Mutations
Rare versus Common Variation
As dictated by the principles of natural selection, most genetic variation is not deleterious, and the induced phenotypic variability can be beneficial as a source on which evolution may act. From a clinical standpoint, it is helpful to dichotomize genetic variation into common and rare variation, while accepting that genetic variation is likely a continuum, and the choice of cutoff could be considered arbitrary. Rare genetic variants are of low frequency in the population (<1% frequency), either because they are deleterious and selected against, or because they are new and most often benign. Common genetic variation (>1% to 5% population frequency), on the other hand, is either adaptive, neutral, or not deleterious enough to be subject to strong negative selection; such variants are referred to as polymorphisms. The preeminent genetic model has been that common disease susceptibility is related to common genetic variation, and more rare forms of disease are caused by rare genetic variants, so-called mutations, which act in a Mendelian fashion. In contrast, common variants or polymorphisms may increase susceptibility for disease, but alone are not sufficient to cause disease (see Common Neurological Disorders and Complex Disease Genetics).
Polymorphisms and Point Mutations
The most prevalent form of genetic polymorphism is the single nucleotide polymorphism (SNP), which occurs on average every 300 to 1000 base pairs in the human genome. Most of these SNPs are relatively benign on their own and do not directly cause disease, so for the purposes of this initial discussion, we will concern ourselves primarily with mutations: rare genetic variants sufficient to cause disease. Pathogenic mutations can occur in numerous ways and vary from single nucleotide changes to gross rearrangements of chromosomes (Fig. 40.4). Owing to the large volume of DNA in the human genome, heritable mutations can arise spontaneously in the germline over time through errors in DNA replication or from DNA damage by metabolic or environmental sources despite the constant surveillance of extensive cellular preventive proofreading and repair mechanisms. Thus, mutations can be inherited from the parent or occur de novo in the germline. An example of a common de novo variant is trisomy 21, which causes Down syndrome (discussed further in Chromosomal Analysis and Abnormalities). The smallest pathogenic alterations, termed point mutations, involve a change in a single nucleotide within a DNA sequence. A point mutation can result in one of three possible effects with respect to protein: (1) a change to a different amino acid, called a missense mutation, (2) a change to a termination codon, called a nonsense mutation, or (3) creation of a new sequence that is silent with regard to protein sequence but alters some aspect of gene regulation, such as RNA splicing or transcriptional expression levels. Nonsense mutations can cause premature truncation of a protein, whereas a missense mutation can affect a protein in different ways depending on the chemical properties of the new amino acid and whether the change is located in a region of functional importance.
It should be emphasized that not all point mutations are disease-causing variants, although until recently, many considered that a premature stop codon was a “smoking gun.” Whole genome sequencing demonstrates that more than 100 such nonsense mutations may exist per genome, and the vast majority are expected to be relatively benign (Lupski et al., 2010; see Whole Genome/Exome Sequencing in Disease Gene Discovery). So in many cases, the pathogenicity of rare variants is not immediately discernable, and without strong statistical or functional evidence, labeling such genetic variation a mutation is premature and may be misleading. It is likely that most of these, including some variants thought previously to cause rare Mendelian diseases, may simply be benign genetic variation. This is because even a complete knockout of one allele caused by a premature stop codon (haploinsufficiency) may have no discernable effect on gene function for a majority of genes in the human genome (Lupski et al., 2010; Ng et al., 2009; Yngvadottir et al., 2009).
Occasionally, silent coding mutations or point mutations in noncoding regions may be significant for disease if they damage sequences important for gene expression (e.g., transcriptional and/or RNA processing regulatory elements). It has been estimated that up to half of all disease-causing mutations impact RNA splicing, which can have dire consequences given the importance of splicing to regulated gene expression. Such is the case for frontotemporal dementia with parkinsonism linked to chromosome 17 (FTDP-17), where in some populations, the most common mutations disrupt splicing, causing a pathogenic imbalance in tau isoforms (D’Souza and Schellenberg, 2005). As for noncoding mutations, given the large volume of such sequences in the human genome—perhaps up to 96%—and our still imprecise ability to predict sequences required for regulation or to interpret identified sequence changes without direct experimentation, the majority of these mutations likely go unrecognized. It is hoped that the next generation of sequencing and bioinformatic technologies will allow for a better understanding of the role of these types of mutation in human disease.
Structural Chromosomal Abnormalities and Copy Number Variation
Small deletions and insertions can occur through slippage and strand mispairing at regions of short, tandem DNA repeats during replication. If the deletion or insertion is not a multiple of three, a frameshift will result, which leads to the translation of an altered protein sequence from the site of the mutation. On a larger scale, errors of chromosomal replication or recombination can result in inversions, translocations, deletions, duplications, or insertions (Stankiewicz and Lupski, 2010). When the region of deletion or duplication is greater than 1 kb, this is referred to as a copy number variation (CNV). Copy number variation is far more common than previously suspected, and it is estimated that at least 4% of the human genome varies in copy number (Conrad et al., 2010; Redon et al., 2006), much of which is commonly observed in the population and benign (Conrad et al., 2010). However, some rare CNVs such as the recurrent chromosome 17p12 duplication underlying most cases of Charcot-Marie-Tooth type 1A (Shchelochkov et al., 2010) or the alpha-synuclein triplication that can cause Parkinson disease (PD)(Singleton et al., 2003) are pathogenic and act in a Mendelian fashion. Even though such changes may be extensive, they may not be pathogenic if they do not disrupt expression of any key genes. This is particularly true for balanced translocations where genetic material is rearranged between chromosomes, yet no significant portion is actually lost. Although an individual with such a condition may be normal, if the germline is affected, their offspring may receive unbalanced chromosomal material and consequently develop a clinical phenotype (Kovaleva and Shaffer, 2003). CNVs will be discussed in greater detail when we consider common and complex disease genetics (see Copy Number Variation and Comparative Genomic Hybridization).
Repeat Expansion Disorders
Most mutations thus far discussed pass from parent to offspring unaltered, and in large affected families, the identical mutation can potentially be traced back generations. In contrast, there is a specific class of mutation, the repeat expansion (Orr and Zoghbi, 2007) (Table 40.2), which is unstable and can present with earlier onset and increasing severity in successive generations, a process known as anticipation. There are several examples of diseases caused by expanded repeats in coding sequence (e.g., most spinocerebellar ataxias, HD), as well as examples in noncoding sequence (e.g., fragile X syndrome, myotonic dystrophy) and within an intron (e.g., Friedreich ataxia). Interestingly, virtually all these disorders show neurological symptoms that can include such features as ataxia, intellectual disability, dementia, myotonia, or epilepsy, depending on the disease. The most common repeated sequence seen in these diseases is the CAG triplet, which codes for glutamine and expansion of which is seen in a variety of the spinocerebellar ataxias (SCAs) including SCA types 1, 2, 3, 6, 7, 17, and dentatorubropallidoluysian atrophy (DRPLA). In addition to protein-specific effects, these disorders likely share a common pathogenesis due to the presence of the polyglutamine repeat regions. In some disorders, the phenotype can be quite different depending on the number of repeats, such as in the FMR1 gene, where more than 200 CCG repeats causes fragile X syndrome, but repeats in the premutation range of 60 to 200, from which fully expanded alleles arise, can result in FXTAS or premature ovarian failure (Oostra and Willemsen, 2009). Although in general, the underlying mutation is similar, each specific repeat expansion has distinct effects on its corresponding gene, and thus in addition to varying phenotypes, they may also show very different inheritance patterns as illustrated later (see Disorders of Mendelian Inheritance).
Chromosomal Analysis and Abnormalities
The DNA coding for an individual gene is generally too small to be visualized microscopically, but it is possible to observe the chromosomes as they condense during mitosis as part of cell division (Griffiths et al., 2002; Strachan and Read, 2003). Traditionally, various staining techniques (e.g., Giemsa) are applied, producing a detailed pattern of banding along the chromosomes that are then photographed and aligned for comparative analysis. This arrangement and analysis of the chromosomes is known as a karyotype (Fig. 40.5). Through these methods, it is possible to visually identify large chromosomal deletions, duplications, or rearrangements. If high-resolution banding techniques are employed, structural alterations on the order of as small as 3 Mb (3 million base pairs) can be detected. More sophisticated techniques can also be employed, such as fluorescent in situ hybridization (FISH). In this method, a short DNA sequence, or probe, that corresponds to a chromosomal region of interest is hybridized with the patient’s DNA and detected visually via excitation of a fluorescent label. FISH can improve on visual resolution by 10- to 100-fold and is in common use for detection of a large number of well-defined genetic syndromes (Speicher and Carter, 2005) such as 15q duplication syndrome, DiGeorge syndrome (22q11 deletion), and Smith-Magenis syndrome (17p11 deletion).
More recent technological developments involving microarray technology (Geschwind, 2003) permit screening of the entire genome at high resolution (from kilobase to single nucleotide level) and are rapidly replacing techniques based on microscopic analysis. This technology is responsible for the emerging appreciation for the structural chromosomal variation in humans mentioned earlier, most of which is submicroscopic. For this section, we will focus on chromosomal alterations that can be detected microscopically, since the clinical implications of many small or rare structural variants identified are not yet clear (see Copy Number Variation and Comparative Genomic Hybridization).
The most common chromosomal abnormalities encountered clinically involve sporadic aneuploidy, either a deletion leaving one chromosome, or a monosomy, or a duplication leaving three chromosomes, or a trisomy (Strachan and Read, 2003). This occurs most frequently via nondisjunction, whereby chromosomes fail to separate during meiosis in the production of the gametes. The majority of aneuploidies are lethal, although there are a few that are viable and will be briefly discussed. Monosomy X (45,XO), also called Turner syndrome, is seen in approximately 1 of every 5000 births and results in sterile females of small stature with a variety of mild physical deformities including webbing of the neck, multiple nevi, and hand and elbow variations, with a very specific cognitive profile in patients with the full deletion (Strachan and Read, 2003). Individuals with additional copies of the X chromosome are also seen. While both females (47,XXX) and males (47,XXY) may have varying degrees of learning disabilities, especially involving language and attention (Geschwind et al., 2000), the males are referred to as having Klinefelter syndrome (KS) due to a phenotype also involving gynecomastia and infertility. XYY males have cognitive profiles similar to XXY males but several studies have suggested more severe social and behavioral problems in some individuals, especially increased aggression, which is rare in KS. Trisomy 21 (47, +21), or Down syndrome, includes profound intellectual impairment, flat faces with prominent epicanthal folds, and a predisposition to cardiac disease. At 1 in approximately 700 births, this is the most common genetic cause of intellectual disability and is associated with advanced maternal age at the time of conception. The other aneuploidies which can survive to term (trisomy 13 [47, +13], Edwards syndrome; trisomy 18 [47, +18], Patau syndrome) have much more severe phenotypes with drastically decreased viability, and death generally occurs within weeks to months after birth.
Disorders of Mendelian Inheritance
In this section we will consider genetic disorders caused by mutation of a single gene. Associating a clinical disease phenotype to the mutation of a specific gene has long been the goal of clinically based, or translational, neuroscience. It is expected that gene identification will eventually lead to an understanding of the disease etiology as well as more accurate diagnosis and better treatments. The ability to determine the genetic nature of most single-gene disease is ultimately based upon the laws of inheritance devised by Mendel in the late 1800s (Griffiths et al., 2002). To summarize these findings in a clinical context, the assumption is made that a phenotypic trait (or in this example, a disease) is caused by the alteration of a single gene. It is important to emphasize that this assumption does not always hold true, particularly for the more complex genetic diseases, as we will discuss later, but it is still true for many diseases seen by neurologists, and more than 3000 Mendelian conditions have been identified to date (OMIM, 2010). Now, if we accept the premise that a given disease is caused by a single gene, we know that for any individual, the gene exists as a pair of alleles with one copy from each parent. However, the alleles may not be equal, and one member of the pair may control the phenotype despite the presence of the other copy. In this case, we say that allele is dominant over the other, the latter of which is labeled as recessive. Depending on the gene and the mutation, as discussed later, a disease allele may be either dominant or recessive. Next, during the development of the gametes, these alleles segregate randomly in a process independent from all other genes. Therefore, the chance of a child receiving a particular allele is entirely random. If these laws all hold true, the observed inheritance of the clinical disease in families will follow a specific pattern that can be used to identify the nature of the causative gene. Although diseases showing Mendelian inheritance are either rare conditions or rare forms of common conditions (e.g., early-onset Alzheimer dementia or PD), identification of such genes are seminal biological advances that can have enormous impact on our understanding of these neurological conditions.
Autosomal Dominant Disorders
Autosomal dominant inheritance is characterized by direct transmission of the disorder from parent to child (Fig. 40.6). Affected individuals are seen in all generations, and a vertical line can be drawn on the pedigree to illustrate the passage of the disorder. Since only one deleterious copy of the disease gene is necessary, risk of transmission from an affected parent is 50%. Since the disorder is autosomal, there is no sex preference, and both males and females can present with the disease. One caveat involves the concept of penetrance, or the percent likelihood that a trait will manifest in a person with a specific genotype. A dominant gene is considered to have complete penetrance if all individuals with a given mutation develop disease. In practice, however, many autosomal dominant genes show varying degrees of penetrance or expressivity, most likely due to the influence of other genes and environmental factors.
There are over 400 examples of diseases with neurological phenotypes that show autosomal dominant inheritance (OMIM, 2010). These conditions include hyperkalemic periodic paralysis (voltage-gated sodium channel NaV1.4 on chromosome 17, often caused by missense mutations), HD (Huntington on chromosome 4, caused by CAG repeat expansion), SCA type 3 (ataxin-3 on chromosome 14, caused by CAG repeat expansion), Charcot-Marie-Tooth type 1B (myelin protein zero on chromosome 1, often caused by missense mutations), early-onset familial Alzheimer disease (AD)(presenilin-1, often caused by missense mutations), frontotemporal dementia with parkinsonism (microtubule-associated protein tau on chromosome 17, often caused by missense or splicing mutations), tuberous sclerosis type 1 (hamartin on chromosome 9, often caused by nonsense mutations and frameshifts), neurofibromatosis type 1 (neurofibromin on chromosome 17, caused by point mutations, frameshifts, and splicing mutations), and familial amyotrophic lateral sclerosis (ALS) (superoxide dismutase-1 on chromosome 21, caused by missense mutations), to name a few. Even rare Mendelian forms of more common syndromes such as epilepsy or sleep disorders (e.g., familial advanced sleep-phase syndrome) have been identified. More detailed lists can be found using the recommended online resources (Table 40.3).
Disease-specific and gene-specific resources | GeneReviews at GeneTests University of Washington, Seattle, WA USA US National Center for Biotechnology Information http://www.ncbi.nlm.nih.gov/sites/GeneTests/ |
Locus Specific Mutation Databases Human Genome Variation Society, Australia http://www.hgvs.org/dblist/glsdb.html |
|
Neuromuscular Disease Center Washington University, St. Louis, MO USA http://neuromuscular.wustl.edu/ |
|
Online Mendelian Inheritance in Man Johns Hopkins University, Baltimore, MD USA US National Center for Biotechnology Information http://www.ncbi.nlm.nih.gov/omim/ |
|
Clinical genetic testing and clinical trials | ClinicalTrials.gov US National Institutes of Health http://clinicaltrials.gov/ |
GeneTests University of Washington, Seattle, WA USA US National Center for Biotechnology Information http://www.ncbi.nlm.nih.gov/sites/GeneTests/ |
|
Genomic variation and other genome resources | Alternative Splicing and Transcript Diversity Database European Molecular Biology Laboratory—European Bioinformatics Institute http://www.ebi.ac.uk/astd/ |
Catalog of Published Genome-Wide Association Studies US National Human Genome Research Institute http://www.genome.gov/gwastudies/ |
|
Ensembl Databases European Molecular Biology Laboratory—European Bioinformatics Institute Wellcome Trust Sanger Institute, UK http://www.ensembl.org/ |
|
Database of Genomic Variants The Centre for Applied Genomics, Canada http://projects.tcag.ca/variation/ |
|
International HapMap Project http://hapmap.ncbi.nlm.nih.gov/index.html |
|
Single Nucleotide Polymorphism Database US National Center for Biotechnology Information http://www.ncbi.nlm.nih.gov/projects/SNP/ |
|
1000 Genomes Project http://www.1000genomes.org/ |