CHAPTER 83 Basic Three-Dimensional Postprocessing in Computed Tomographic and Magnetic Resonance Angiography
The three-dimensional data acquired by computed tomography angiography (CTA) or magnetic resonance angiography (MRA) can be processed off-line using a variety of commercially available techniques that enable isolation and improved viewing of specific vascular segments and their anatomic relationships. The most popular and widely available postprocessing tools for CTA and MRA data are multiplanar reformation (MPR), maximum intensity projection (MIP), and volume rendering (VR).1–5 This chapter reviews these basic methods for postprocessing of CTA and MRA data, highlighting their strengths and pitfalls. For different clinical applications, the specific methods of highest value will vary. Individual techniques for various anatomic regions are covered elsewhere in this text.
MULTIPLANAR REFORMATION
MPR refers to the reconstruction of three-dimensional data into a new orientation. For example, a volumetric CTA data set acquired in the axial plane can be reconstructed for viewing in a coronal plane or a plane of any obliquity using MPR. MPR images can be reconstructed into linear (e.g., coronal, sagittal, axial, oblique; Figs. 83-1 and 83-2) or curved plane images (Fig. 83-3). The process does not change the original data or voxels. In the MPR process, interpolation is needed to rearrange data into a different coordinate system. Curved reformatting is useful for viewing tortuous vessels such as the coronary arteries on CTA because it unravels the tortuous segments for linear viewing of the vessel (i.e., straightens out the vessel in length). It is particularly useful for showing vascular detail in cross-sectional profile along the vessel length, facilitating characterization of stenoses or other intraluminal abnormalities. The pitfall is that manual definition of curved planes may not be accurate for actual measurements and is potentially a time-consuming process because operator interaction is often required. Automated curve detection methods can expedite processing but fail if there are image artifacts within the data (e.g., motion blurring or high-value nonvascular structures such as calcium on CTA). These may be erroneously labeled as vessel lumen, thereby introducing inaccurate curved vascular lumen reformation.
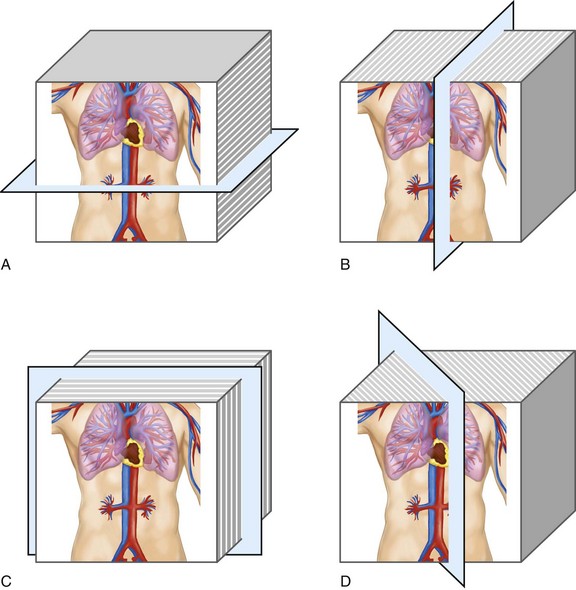
FIGURE 83-1 Multiplanar reformation (MPR). A, The diagram represents a stack of axial images that can be reconstructed and interpolated to a three-dimensional volume. Sagittal (B) and coronal (C) MPR views can be sampled and generated from it. D, It can also be sampled in an arbitrary oblique MPR view, which can be used for improved visualization of specific vascular segments.
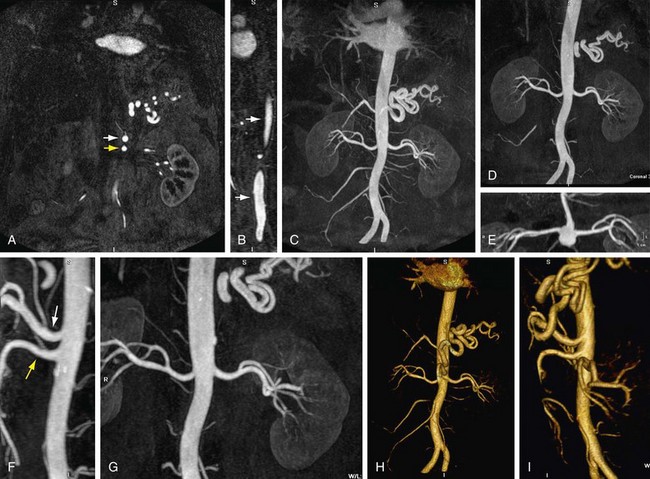
FIGURE 83-2 Normal renal contrast-enhanced three-dimensional MPR. With MPR, a single-voxel view of the three-dimensional data set can be individually visualized. A, On the coronal MPR, the celiac trunk (white arrow) and superior mesenteric artery (yellow arrow) are seen but their relationship to the renal arteries and aorta is not evident because the coronal MPR does not include these structures. B, Similarly, on a sagittal MPR, only a portion of the abdominal aorta (arrows) is included on this coronal MPR obtained at the edge of the abdominal aorta. C, On a whole-volume coronal MIP, the renal arteries and their origin from the abdominal aorta are much more clearly viewed than on the thin single-voxel MPR. On subvolume MIP (D, coronal; E, axial; F, sagittal; G, oblique coronal), overlapping structures can be excluded from the slab to visualize the renal arteries preferentially. F, On the sagittal subvolume MIP, the origins of the celiac trunk (white arrow) and superior mesenteric artery (yellow arrow) can be clearly seen arising anteriorly from the abdominal aorta. On VR (H, coronal; I, oblique sagittal), the observer is provided with improved depth perception of the abdominal aorta and renal arteries compared with MIP images (compare H with C).
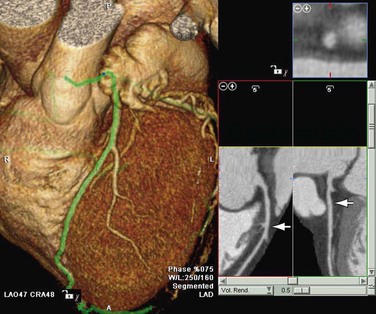
FIGURE 83-3 Normal coronary CTA. Lower right images, The proximal left anterior descending (LAD) coronary artery (arrows) is well seen on curved MPR. Top right image, Cross section of the proximal LAD is shown. Left, Actual green line tracing used to predict the curved MPR on a VR projection of the coronary CTA. Ao, aortic root.
Nearest Neighbor Interpolation
Interpolation is an important concept in the context of image reconstruction. As demonstrated in Figure 83-4, a slice of an original acquired image is composed of known data points. In this example, the circles are known data grids. Triangles are points that need to be interpolated because of MPR needs. The left known data signal intensity (SI) value is SIa = 100, and right known signal intensity value is SIb = 100. The simplest interpolation is the nearest neighbor. In nearest neighbor interpolation, the new interpolated data point (i.e., triangle) is closer to the right data point, where SIb is, so the unknown interpolated signal intensity (SIi) is set to be the same as SIb, that is, SIi.
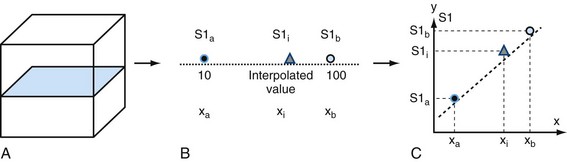
FIGURE 83-4 Multiplanar reformation. A, A slice of the original acquired image composed of known data points. B, Example of two known grids with an oblique line that are defined by oblique MPR. Circles are data grids that are known. Triangles are points that need to be interpolated because of MPR needs. C, Explanation in SI – x coordinate.
Linear Interpolation
Another simple method is linear interpolation. Using the same example as in Figure 83-4, the distance for the points are xa = 1, xi = 3, and xb = 4. The signal intensities for known points are SIa = 10 and SIb = 100. The interpolated value SIi for the triangle is linear interpolation as given by equation (1):